TirSA: A Three Stage Approach for UAV-Satellite Cross-View Geo-Localization Based on Self-Supervised Feature Enhancement
IEEE Transactions on Circuits and Systems for Video Technology(2024)
摘要
Cross-view geo-localization aims to associate geographical location with different view images shot from different platforms. One of the critical challenges is how to effectively emphasize architectural features and reducing background interference to achieve robust cross-view matching. Most of the existing methods fail to adequately address the features of buildings, treating foreground and background equally. Leveraging prior knowledge to enhance the features of crucial architectural foreground yields greater benefits in Geo-Localization. A comprehensive three stage approach (TirSA) is proposed in this paper, which consists of three components: Pre-processing, Generate Feature Embedding, and Post-processing. In the Pre-processing stage, we employ a self-supervised feature enhancement method (SFEM) to obtain the building aware mask. Without adding additional auxiliary information, the model is guided to learn from discriminative building regions. Besides, in the Generate Feature Embedding stage, we propose an adaptive feature integration module (AFIM) to enhance feature representation capability. We also train the Siamese network using a novel improved cross-domain triplet loss to reduce the impact of inter-view domain gap. Finally, in the Post-processing stage, we employ a re-ranking method to optimize the initial retrieval list, further enhancing the matching accuracy. Remarkably, extensive experiments show that our proposed TirSA exceeds state-of-the-art by a large margin and achieves optimality in both drone-view target localization and drone navigation. Especially in the drone navigation task, our method is superior to the existing methods, achieving an improvement of approximately 5%. Code will be released at https://github.com/SunJ1025/TirSA.
更多查看译文
关键词
self-supervised learning,geo-localization,image retrieval,cross domain
AI 理解论文
溯源树
样例
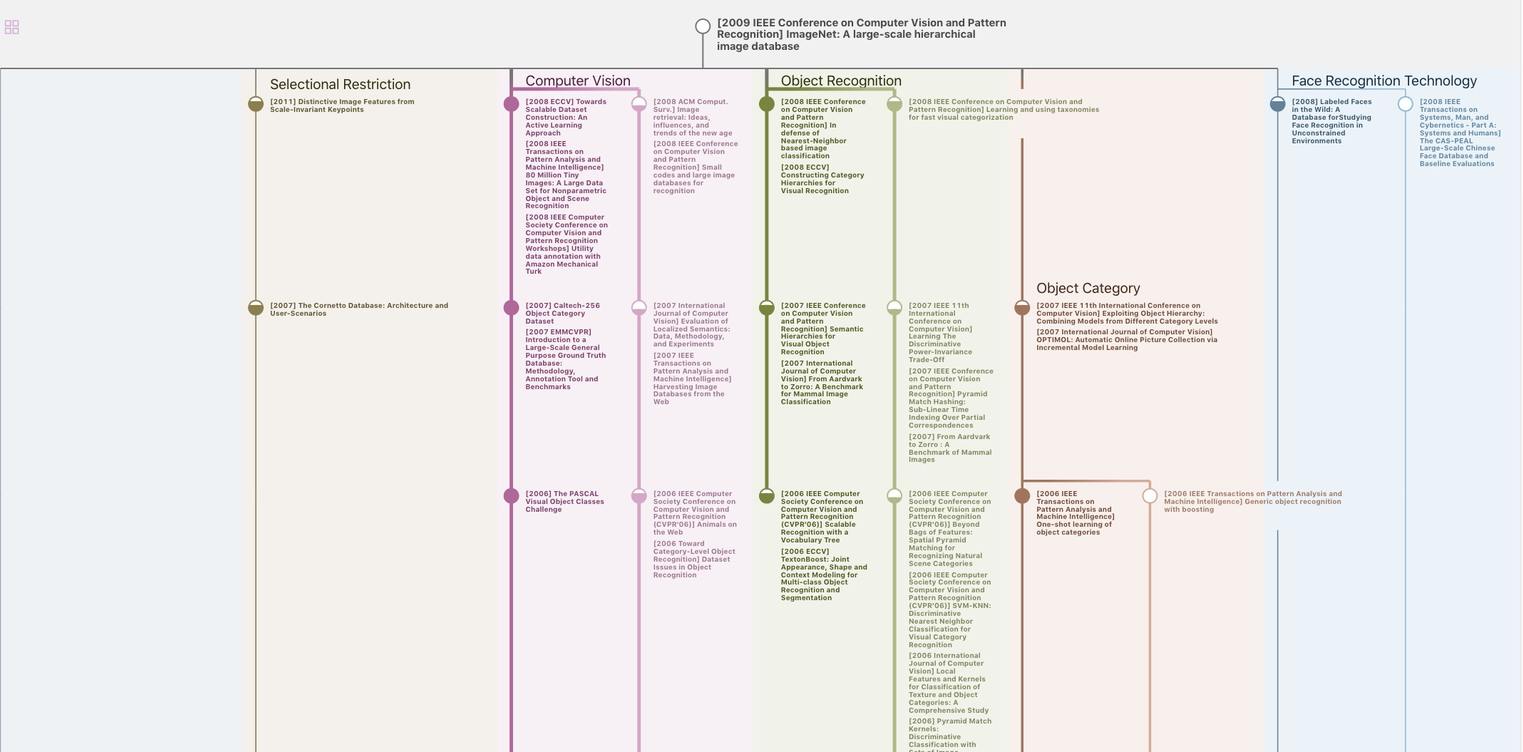
生成溯源树,研究论文发展脉络
Chat Paper
正在生成论文摘要