Dynamic Reasoning Network for Image-Level Supervised Segmentation on Metal Surface Defect.
IEEE Trans. Instrum. Meas.(2024)
摘要
Segmenting defects on metal surfaces is crucial for assuring product quality in industrial manufacturing. Current defect segmentation solutions based on deep learning heavily depend on extensive annotations for model training, resulting in a time-consuming and labor-intensive process. In order to alleviate the burden of accurate annotation in pixel-level, this paper proposes a novel weakly supervised network to segment metal surface defects only using the image-level label. Specifically, the network input comprises two defective images belonging to the same category and one normal image without any defects. The triplet images are put into a weight-shared backbone to extract the representation of foreground and background. This combination can efficiently offer similarity data for identical defects and distinguish between normal and abnormal samples. Then, a novel attention activation aggregation module is responsible for combining the features of different levels and obtaining more accurate maps, which is beneficial to alleviate the false positive and negative predictions. Finally, we design a dynamic reasoning module and triplet loss to explore the relevance of a common category and identify the difference between the foreground and the background. It also improves the model’s generalization to different morphological changes of defects. Extensive comparison experiments and ablation studies prove that the proposed method achieves state-of-the-art performance on six metal surface defect datasets.
更多查看译文
关键词
Metal surface defect segmentation,weakly supervised learning,classification activation map,dynamic reasoning,attention mechanism
AI 理解论文
溯源树
样例
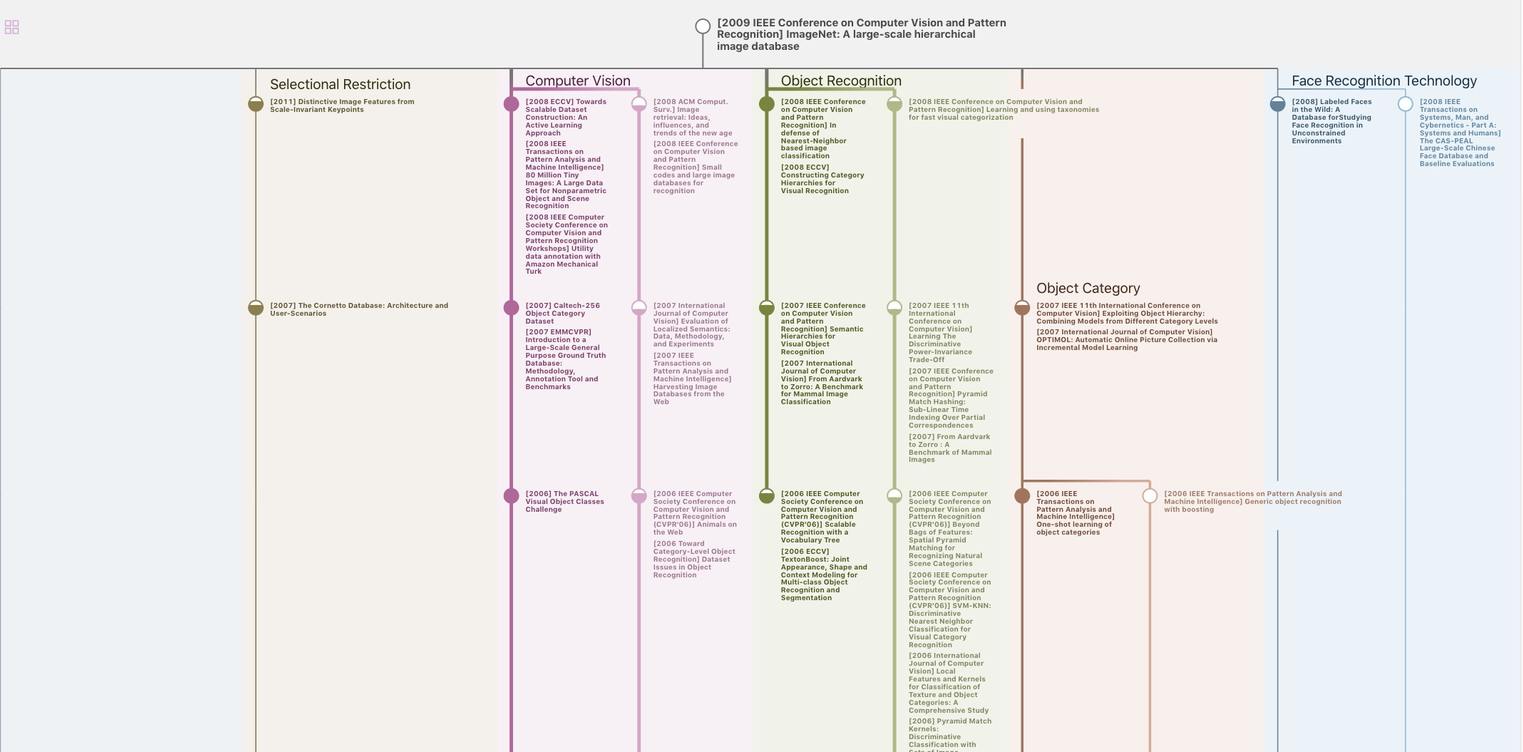
生成溯源树,研究论文发展脉络
Chat Paper
正在生成论文摘要