Exploring the Potential and the Practical Usability of a Machine Learning Approach for Improving Wall Friction Predictions of RANS Wall Functions in Non-equilibrium Turbulent Flows
Flow, Turbulence and Combustion(2024)
摘要
A data-driven wall function estimation approach is proposed, aimed at accounting for non-equilibrium effects in turbulent boundary layers in RANS simulations of wall bounded flows. While keeping key simplifying hypothesis of standard wall functions and their general structure, the law-of-the-wall is replaced by a fully connected feed-forward neural network. The latter is trained to infer wall friction from the local flow state at the first of-wall nodes, described by an extended set of flow variables and gradients. For this purpose, the neural network is trained on high-fidelity wall resolved simulation data. It is then applied to formulate two different wall functions trained on high-fidelity data: a backward-facing step and a round jet impacting a flat wall. After integration into an industrial CFD code, they are applied to perform RANS simulations of the flow configurations they were trained for, and are shown to yield a largely improved prediction of wall friction as compared to standard wall functions. Finally, key issues related to the practical usability in RANS applications of the proposed data-driven approach are critically discussed.
更多查看译文
关键词
Deep learning,Data-driven CFD modeling,Wall functions,RANS
AI 理解论文
溯源树
样例
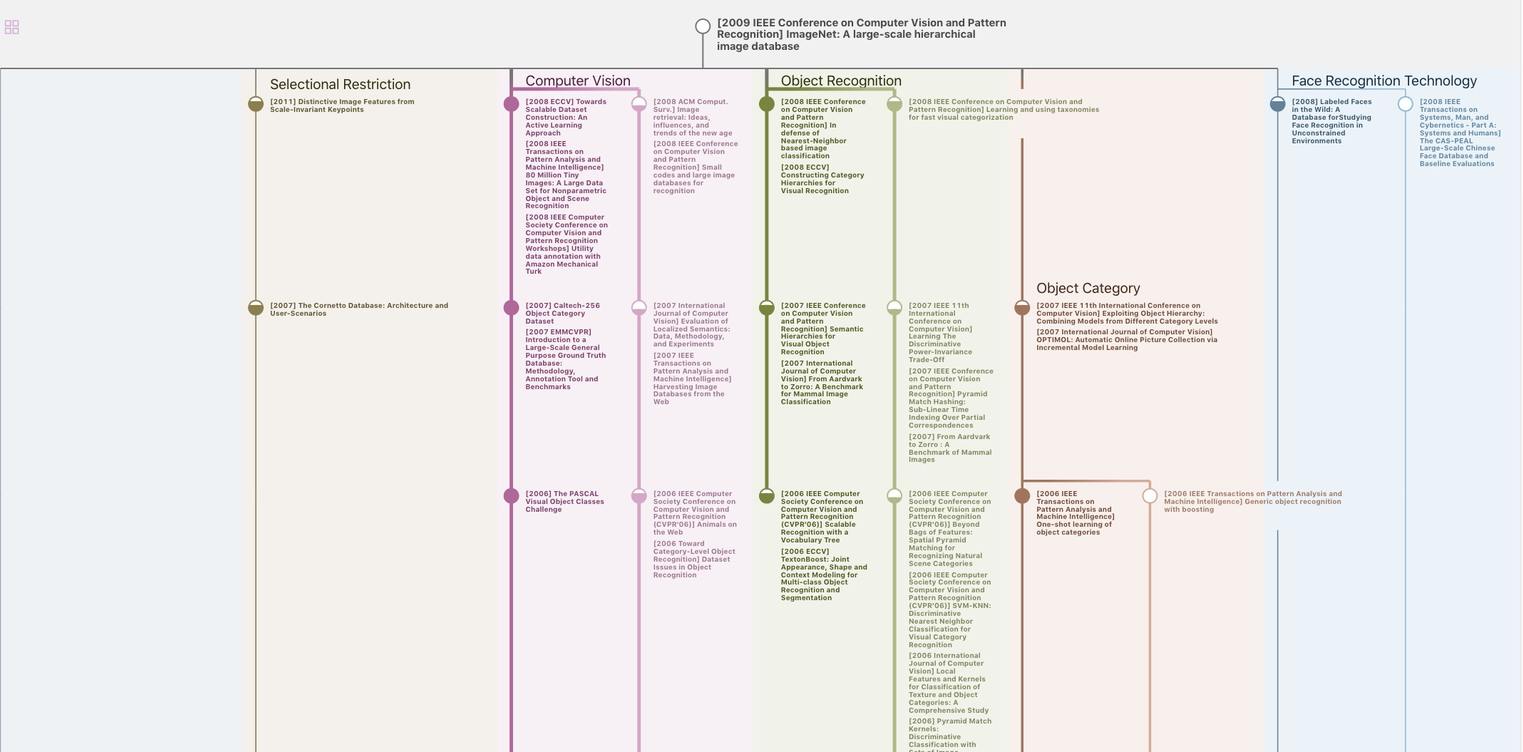
生成溯源树,研究论文发展脉络
Chat Paper
正在生成论文摘要