Fast point completion network
Neural Computing and Applications(2024)
摘要
Point clouds in the real world are often sparse and incomplete. Point cloud completion aims to restore incomplete point clouds into meaningful shapes. In recent years, point cloud completion has attracted the interest of many researchers. In most existing methods, the efficiency of completion is generally ignored in favor of producing meaningful shapes with adequate details. This paper proposes a fast point completion network (FPCN). FPCN is mainly composed of a multi-scale attention encoder (MSAE) and a structural refinement (SR) module. MSAE first obtains multi-scale geometric information by extracting incomplete inputs at different resolutions. After that, MSAE fuses multi-scale geometric information through cross-attention mechanisms. Compared with existing mainstream encoders, MSAE can extract rich geometric information from input point clouds with low complexity. The SR module aims to extract local information from the coarse point clouds to guide the process of extending points. Furthermore, the process of extending points is achieved by a replication strategy. Compared to existing folding-based decoders, the SR module can produce fine point clouds with more local details. Compared to existing transformer-based decoders, the SR module has a lower calculation price by employing a replication strategy to generate high-resolution point clouds. In conclusion, FPCN can restore partial point clouds into meaningful shapes efficiently, and the outcomes of completion contain sufficient details. Extensive experiments on various datasets demonstrate the performance and efficiency of the FPCN in point cloud completion. Source code is available at https://github.com/doldolOuO/FPCN .
更多查看译文
关键词
Deep learning,Point cloud,Shape completion
AI 理解论文
溯源树
样例
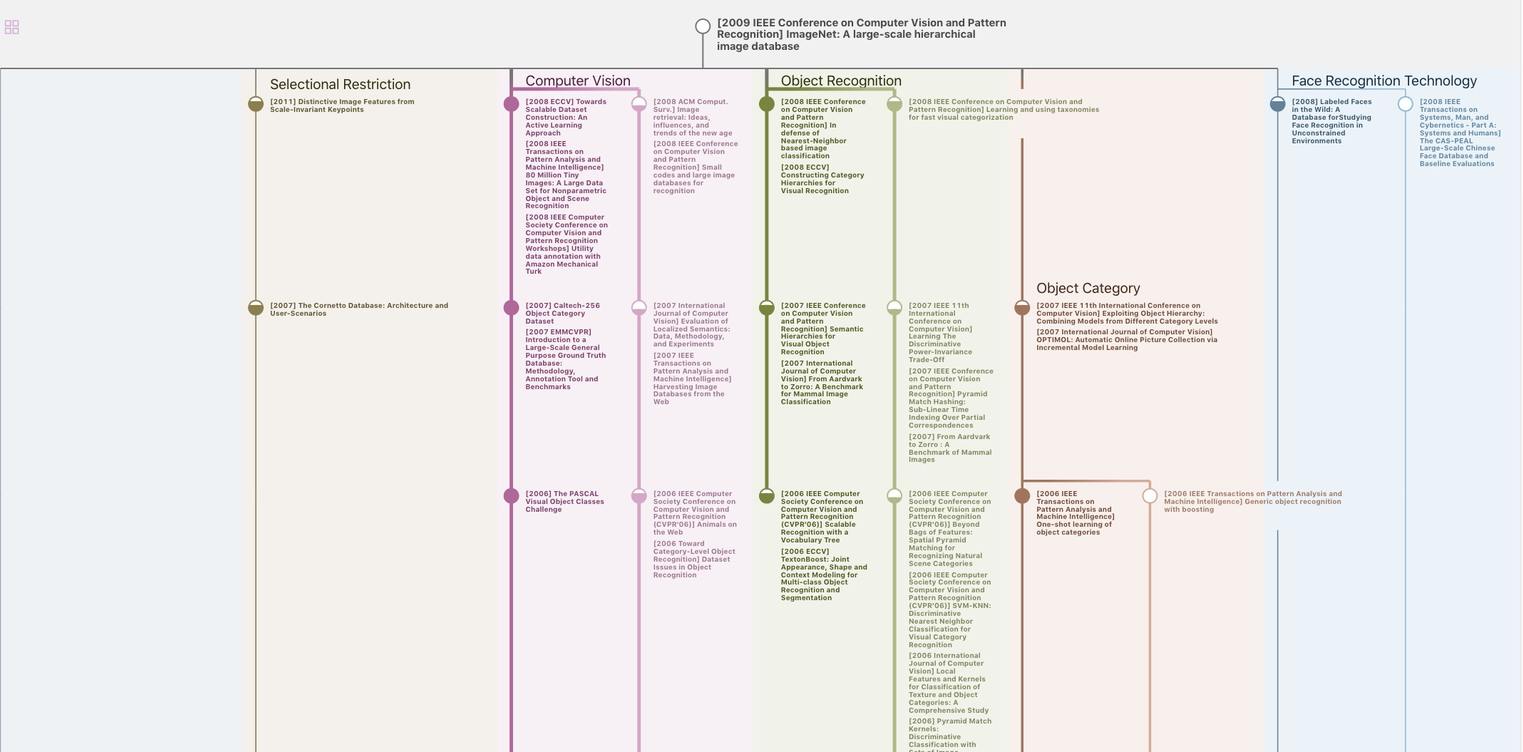
生成溯源树,研究论文发展脉络
Chat Paper
正在生成论文摘要