Road Extraction from Remote Sensing Imagery with Spatial Attention Based on Swin Transformer
REMOTE SENSING(2024)
摘要
Road extraction is a crucial aspect of remote sensing imagery processing that plays a significant role in various remote sensing applications, including automatic driving, urban planning, and path navigation. However, accurate road extraction is a challenging task due to factors such as high road density, building occlusion, and complex traffic environments. In this study, a Spatial Attention Swin Transformer (SASwin Transformer) architecture is proposed to create a robust encoder capable of extracting roads from remote sensing imagery. In this architecture, we have developed a spatial self-attention (SSA) module that captures efficient and rich spatial information through spatial self-attention to reconstruct the feature map. Following this, the module performs residual connections with the input, which helps reduce interference from unrelated regions. Additionally, we designed a Spatial MLP (SMLP) module to aggregate spatial feature information from multiple branches while simultaneously reducing computational complexity. Two public road datasets, the Massachusetts dataset and the DeepGlobe dataset, were used for extensive experiments. The results show that our proposed model has an improved overall performance compared to several state-of-the-art algorithms. In particular, on the two datasets, our model outperforms D-LinkNet with an increase in Intersection over Union (IoU) metrics of 1.88% and 1.84%, respectively.
更多查看译文
关键词
remote sensing applications,road extraction,spatial self-attention,Spatial MLP
AI 理解论文
溯源树
样例
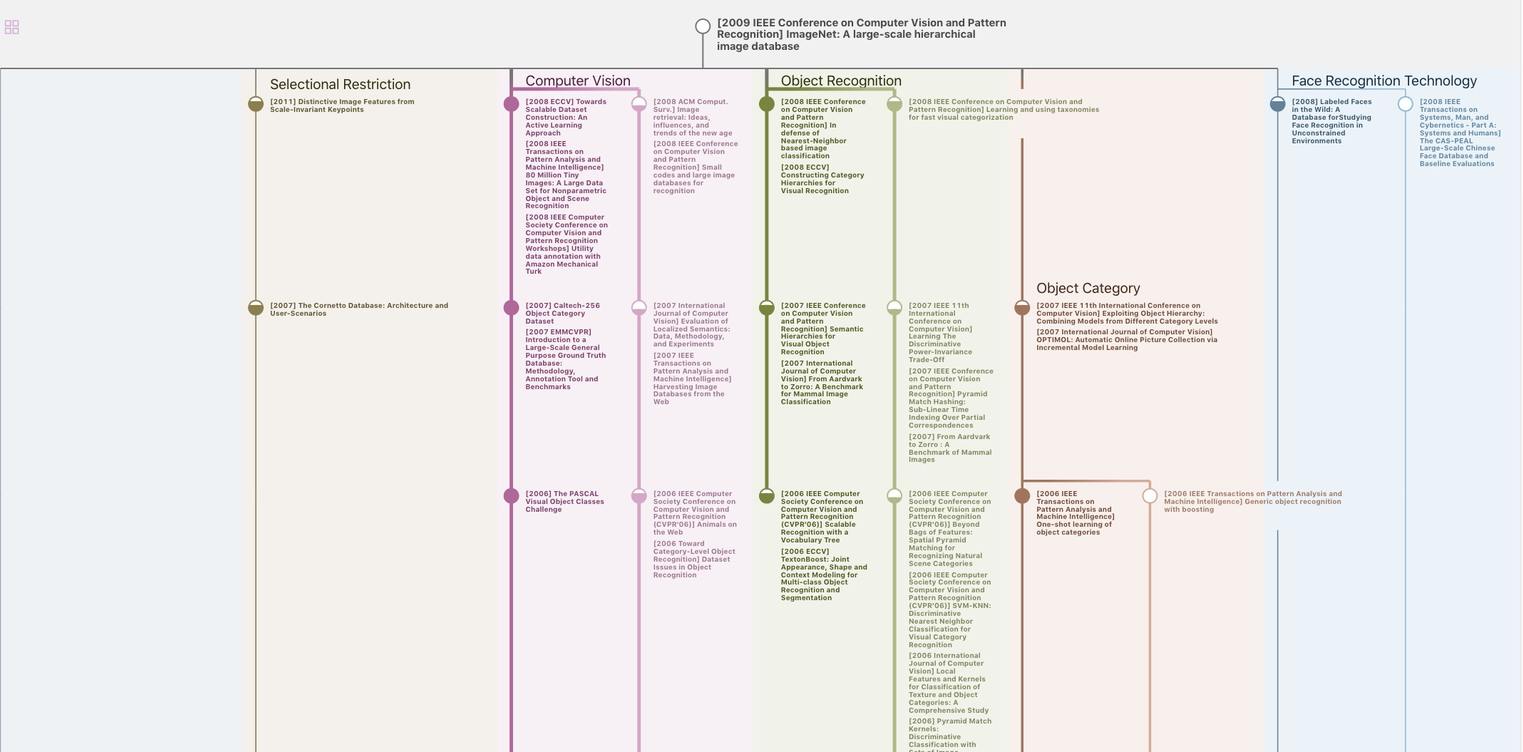
生成溯源树,研究论文发展脉络
Chat Paper
正在生成论文摘要