Sensor-Based Quantification of MDS-UPDRS III Subitems in Parkinson’s Disease Using Machine Learning
Sensors(2024)
摘要
Wearable sensors could be beneficial for the continuous quantification of upper limb motor symptoms in people with Parkinson’s disease (PD). This work evaluates the use of two inertial measurement units combined with supervised machine learning models to classify and predict a subset of MDS-UPDRS III subitems in PD. We attached the two compact wearable sensors on the dorsal part of each hand of 33 people with PD and 12 controls. Each participant performed six clinical movement tasks in parallel with an assessment of the MDS-UPDRS III. Random forest (RF) models were trained on the sensor data and motor scores. An overall accuracy of 94% was achieved in classifying the movement tasks. When employed for classifying the motor scores, the averaged area under the receiver operating characteristic values ranged from 68% to 92%. Motor scores were additionally predicted using an RF regression model. In a comparative analysis, trained support vector machine models outperformed the RF models for specific tasks. Furthermore, our results surpass the literature in certain cases. The methods developed in this work serve as a base for future studies, where home-based assessments of pharmacological effects on motor function could complement regular clinical assessments.
更多查看译文
关键词
Parkinson’s disease,motor symptoms,MDS-UPDRS,wearable devices,inertial measurement unit,machine learning
AI 理解论文
溯源树
样例
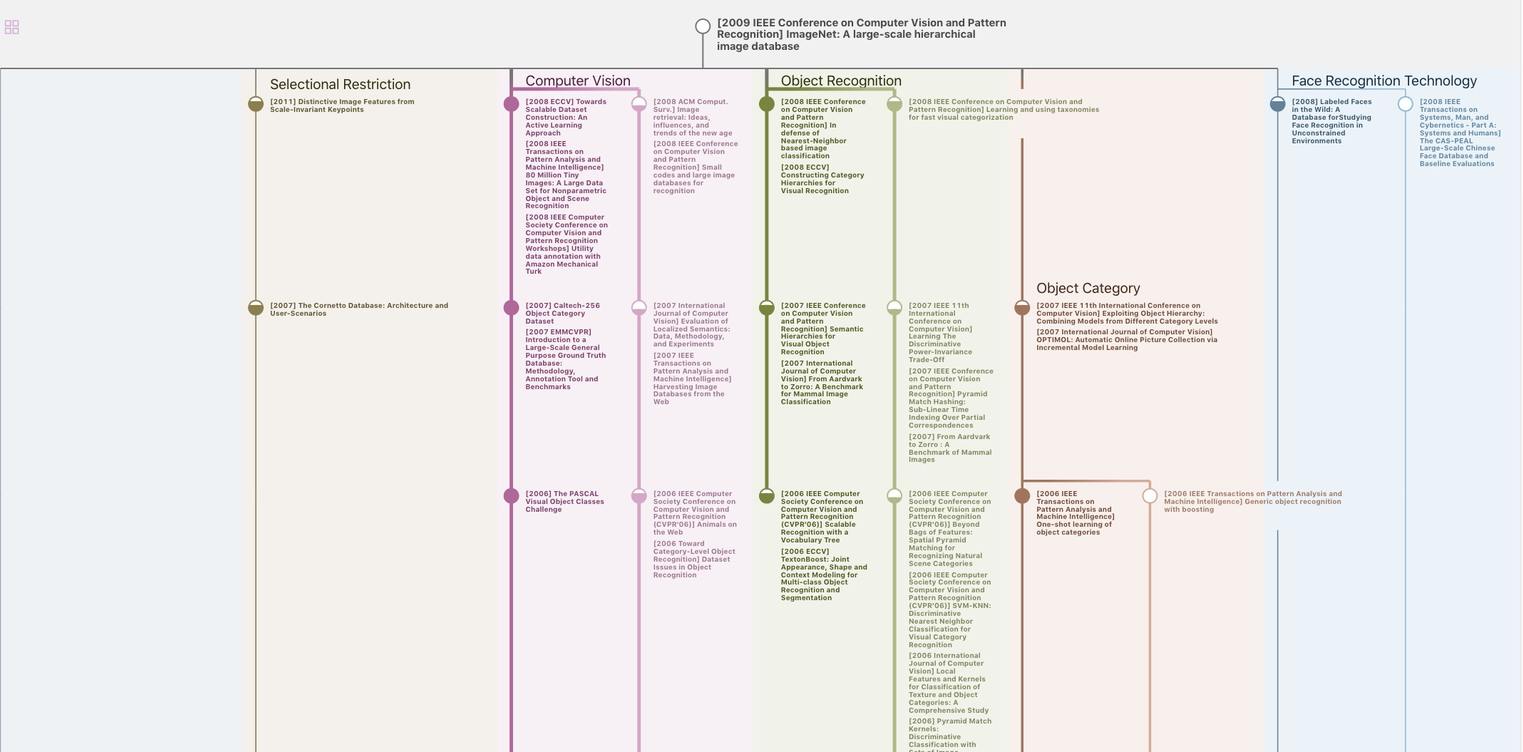
生成溯源树,研究论文发展脉络
Chat Paper
正在生成论文摘要