An Improved Analytic Dictionary Learning Method for Sparse Representation in Gas Recognition and Classification
IEEE Sensors Journal(2024)
摘要
The sparse dictionary learning algorithm has extensive applications in various domains, including electronic nose systems (EN) and pattern recognition. To address the challenges associated with slow processing times and inefficiencies in the sparse representation classification algorithm (SRC), this study proposes a novel dictionary learning method named the mapping discriminant dictionary learning algorithm (MDDL). Leveraging the Frobenius norm for model minimization, MDDL concurrently tackles sparse and analytic dictionaries alongside sparse coefficients using Normal Equations. It optimizes electronic nose system recognition by mapping sparse coefficients with analytic dictionary learning and clustering techniques, effectively improving sparsity between classes and reducing intra-class distance. The algorithm significantly reduces SRC runtime while maintaining high accuracy. The comparative analysis demonstrates MDDL’s exceptional accuracy at 99.6078% and a remarkable up to 50-fold reduction in model training time compared to traditional algorithms. Similarly, it offers advantages of fast training and high recognition rates compared to other dictionary learning algorithms. These findings highlight MDDL as a highly effective and efficient tool for advancing electronic nose systems, demonstrating its superiority over some dictionary learning and traditional pattern recognition algorithms.
更多查看译文
关键词
Analytic dictionary,mapping discriminant dictionary learning (MDDL),electronic nose (EN),sparse representation classification (SRC)
AI 理解论文
溯源树
样例
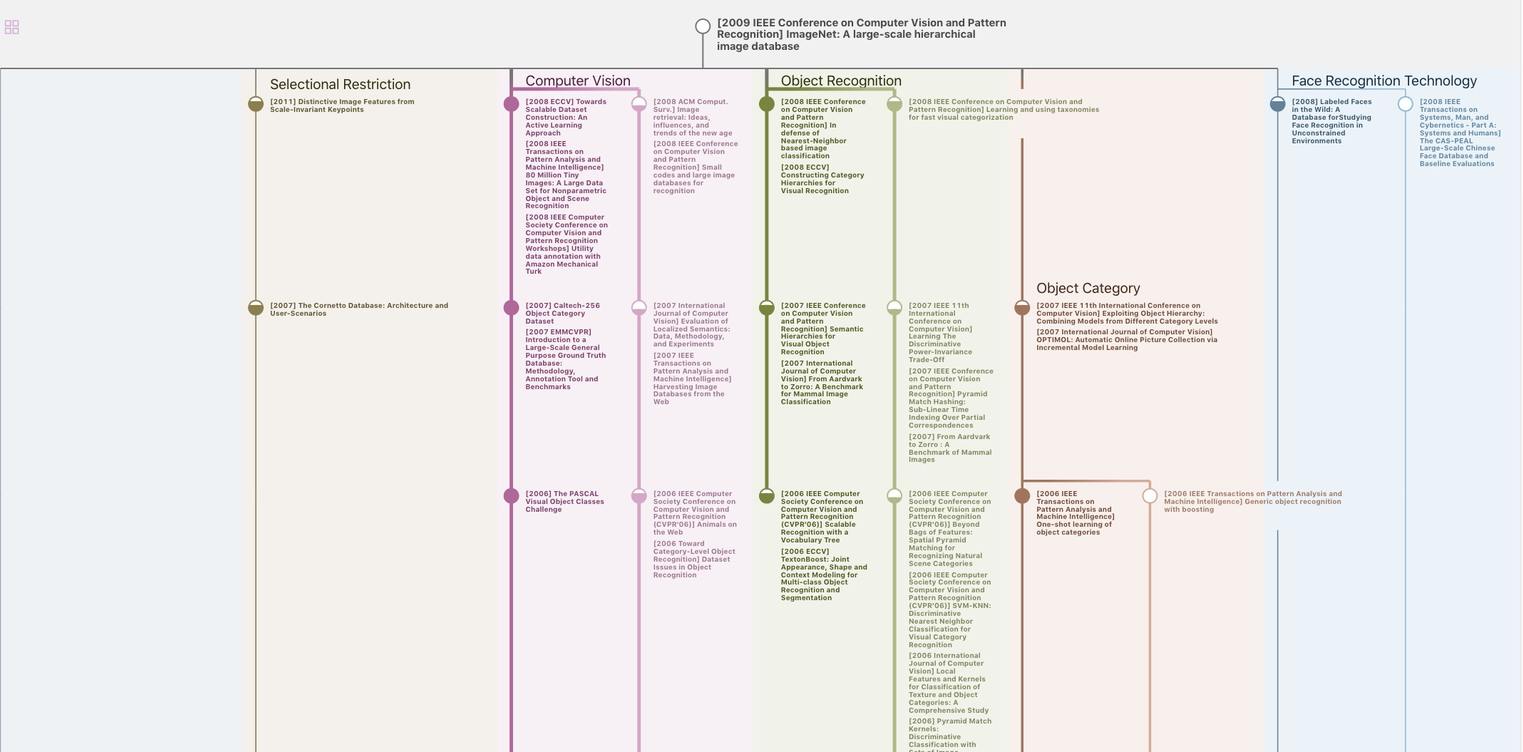
生成溯源树,研究论文发展脉络
Chat Paper
正在生成论文摘要