Toward ultra-high strength high entropy alloys via feature engineering
Journal of Materials Science & Technology(2024)
摘要
Machine learning assisted design of materials is so far based on features selected by considering the accuracy of model predictions, and those features do not necessarily ensure a high efficiency in searching for new materials. Here we estimate the efficiency of active learning loop by resampling method using available data as an alternative criterion for selection. The selected features allow an optimization of targeted property with as few new experiments as possible. Input those features into machine learning, we synthesized new high entropy alloys (HEAs) with strengths 2.8-3.0 GPa within five experimental iterations. The alloy AlVCrCoNiMo is found to possess compressive specific yield strengths of 397, 144 and 105 (MPa cm3)/g at 25, 800 and 900°C, respectively. The specific yield strength of AlVCrCoNiMo alloy at 800°C is about twice that of the commercial Inconel 718 and the typical refractory HEA of VNbMoTaW. A unique microstructure consisting of multi-scale hierarchical B2 precipitates with coherent interfaces to the BCC matrix strengthens the alloy. Our strategy of maximizing active learning efficiency provides a recipe for selecting features that accelerate the optimization of targeted property.
更多查看译文
关键词
Machine learning,Feature selection,High entropy alloys,Active learning,Precipitation
AI 理解论文
溯源树
样例
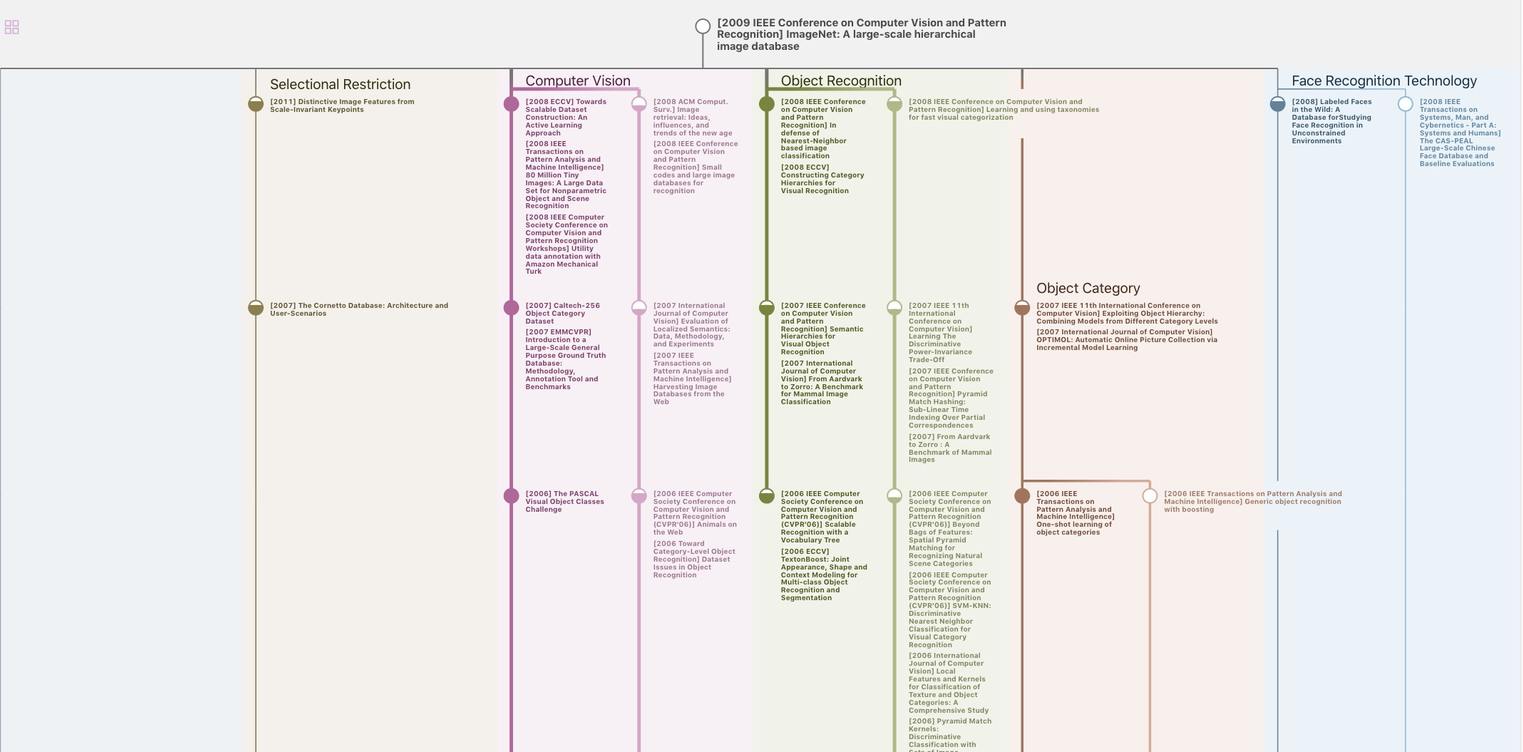
生成溯源树,研究论文发展脉络
Chat Paper
正在生成论文摘要