Pre-Processing-based Fast Design of Multiple EM Structures with One Deep Neural Network
IEEE Transactions on Antennas and Propagation(2024)
摘要
Deep learning plays a vital role in the design of electromagnetic (EM) structures. However, in current research, a single neural network typically supports only one structure design and requires a complex framework to accommodate multiple structure designs. This paper proposes using one neural-assist design for facilitating multiple EM structures. We employ two filling methods to control the vector length, an identification method to ensure accurate prediction results, and a random auxiliary vectors method to increase the data volume and reduce loss. Subsequently, we design a forward neural network (FNN) and an inverse neural network (INN) using the proposed method. The developed neural network is used to complete the dual-passband frequency selective surface (DP-FSS), space-time-coding digital metasurface element (STCDME), single/dual absorbing metasurface (SDAM), and dual-stopband frequency selective surface (DS-FSS) designs. The mean absolute error (MAE) loss values for the FNN/INN predictions and actual results for these four structures are 0.019/0.116, 0.035/0.602, 5.14/0.146, and 0.018/0.07, respectively. Finally, we design four structures with the well-training network, fabricate DP-FSS and DS-FSS, and measure them in an anechoic chamber. The measurement and simulation results are in good agreement. The proposed method significantly reduces the complexity of multiple EM structure designs, decreases the need for multiple neural networks, and simplifies the design framework, thereby contributing to the development of AI-assisted EM structures.
更多查看译文
关键词
DNN,interpolation filling,identification method,multiple EM,random auxiliary vector Method,0-vector filling
AI 理解论文
溯源树
样例
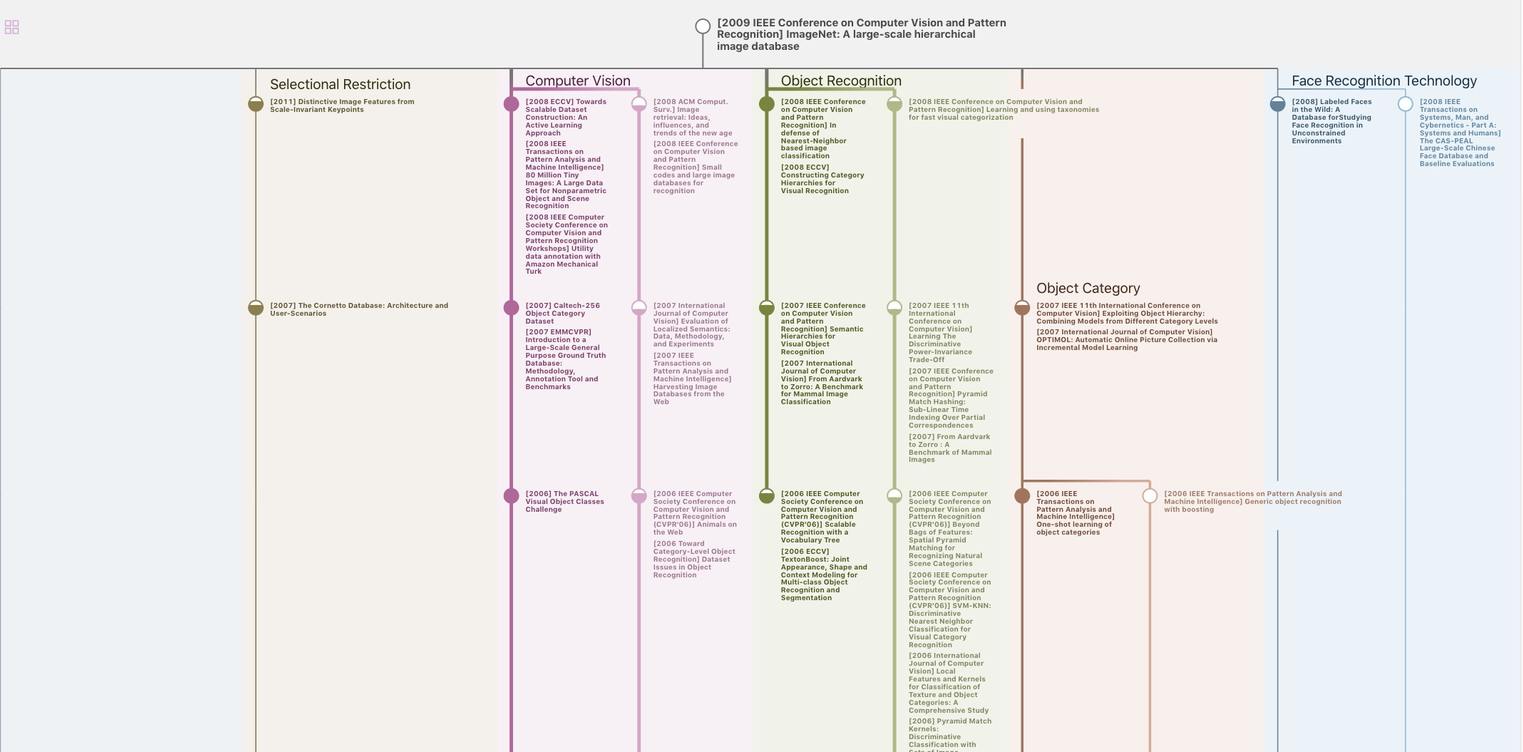
生成溯源树,研究论文发展脉络
Chat Paper
正在生成论文摘要