UPetu: A Unified Parameter-efficient Fine-tuning Framework for Remote Sensing Foundation Model
IEEE Transactions on Geoscience and Remote Sensing(2024)
摘要
Recent advancements in remote sensing foundation models have unveiled their tremendous potential in addressing earth observation tasks. Presently, when large-scale foundation models are transferred to downstream tasks, the prevalent approach is to adopt the full-tuning strategy, resulting in significant increases in storage demands and computational costs. Although the introduction of parameter-efficient fine-tuning (PEFT) has mitigated this issue to some extent, mainstream PEFT methods are primarily designed for classification tasks and often prove insufficient to meet the demands of dense prediction tasks. In order to overcome the aforementioned limitations, we propose a unified PEFT framework UPetu, encompassing two essential and complementary modules: the efficient quantization adapter module (EQAM) and the context-aware prompt module (CAPM). EQAM is specifically designed to enhance the correlation between fine-grained feature information and task-specific knowledge through the introduction of quantization linear layers and non-linear activation functions. Additionally, CAPM is introduced to acquire rich contextual features by incorporating trainable prompts into multi-scale features. The synergistic integration of both modules enhances the representation learning capability and generalization transferability of the foundation model. Extensive experiments on three remote sensing scene classification datasets demonstrate the superiority of UPetu over other fine-tuning methods. With the update of only 0.73% of ConvNeXt-B parameters, our UPetu achieves superior performance compared to full-tuning on the UCM-55, AID-28, and AID-55 datasets. Furthermore, experiments conducted on semantic segmentation and change detection tasks provide additional evidence of the effectiveness and generalization capabilities of the proposed UPetu.
更多查看译文
关键词
Remote Sensing,Foundation Model,Parameter-efficient Fine-tuning
AI 理解论文
溯源树
样例
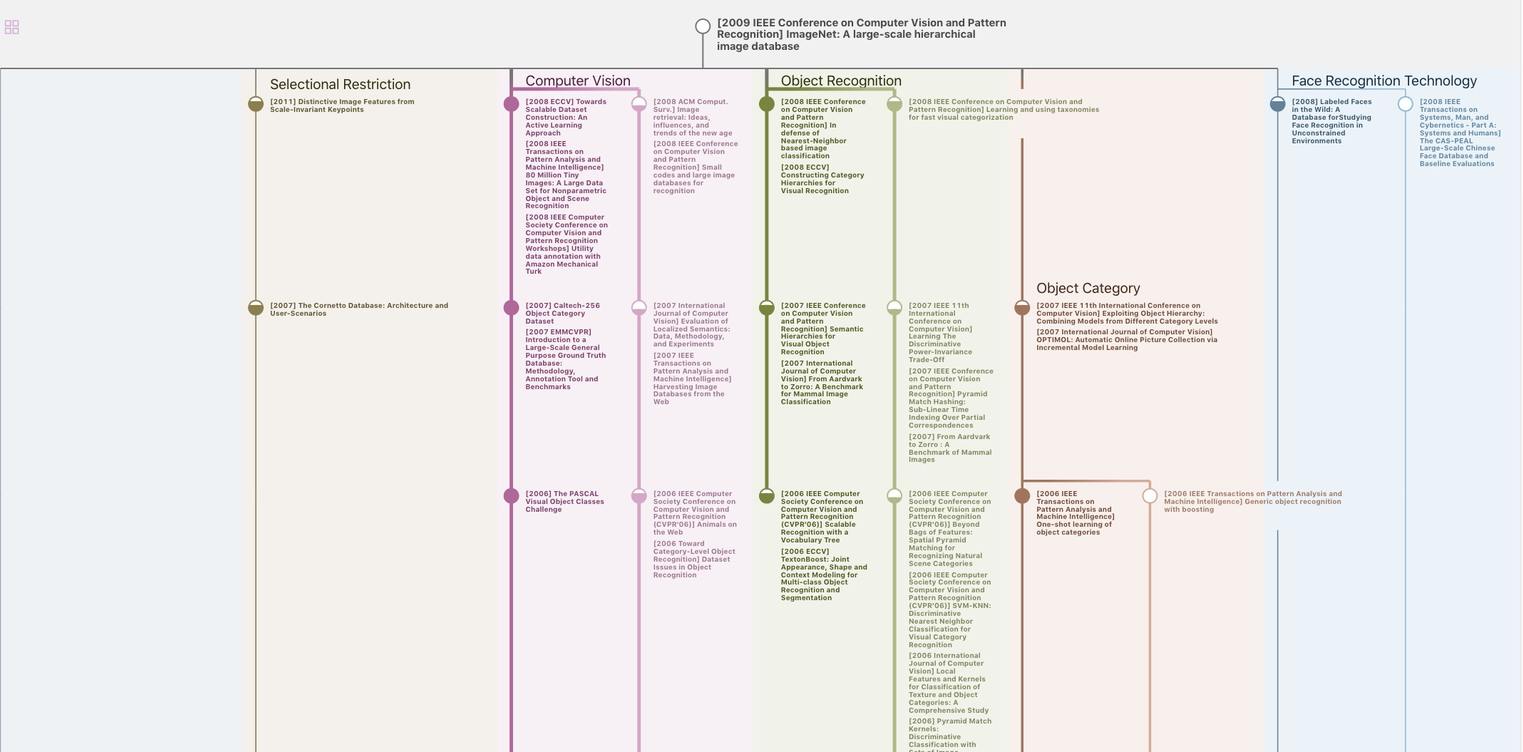
生成溯源树,研究论文发展脉络
Chat Paper
正在生成论文摘要