VFL3D: A Single-Stage Fine-Grained Lightweight Point Cloud 3D Object Detection Algorithm Based on Voxels
IEEE TRANSACTIONS ON INTELLIGENT TRANSPORTATION SYSTEMS(2024)
摘要
In this work, we propose a voxel-based single-stage fine-grained and efficient point cloud 3D object detection algorithm to address the inadequate granularity in point cloud feature extraction tasks and the imbalance between efficiency and accuracy in single-stage point cloud 3D object detection scenarios. We develop a lightweight multibranch cross-sparse convolution network (LMCCN) that is designed to preserve the feature granularity of the original point cloud while achieving enhanced extraction efficiency. Additionally, we introduce a compact fine-grained self-attention augmented bird's eye view (BEV) feature extraction module (CFSAM). This module aims to further refine BEV features, enabling the acquisition of both locally and globally enhanced features and thereby augmentingthe perceptual capabilities of the constructed model. Without bells and whistles, the proposed method attains excellent performance on many autonomous driving benchmarks, with detection accuracies of up to 81.67% on KITTI, 72.74% on ONCE, and 84.00% on nuScenes. Moreover, it reaches a peak detection speed of 46.08 FPS, effectively balancing accuracy with speed.
更多查看译文
关键词
Feature extraction,Point cloud compression,Three-dimensional displays,Object detection,Convolution,Data mining,Computational efficiency,Single-stage,fine-grained,lightweight,multibranch cross-sparse convolution network,compact fine-grained self-attention augmented module
AI 理解论文
溯源树
样例
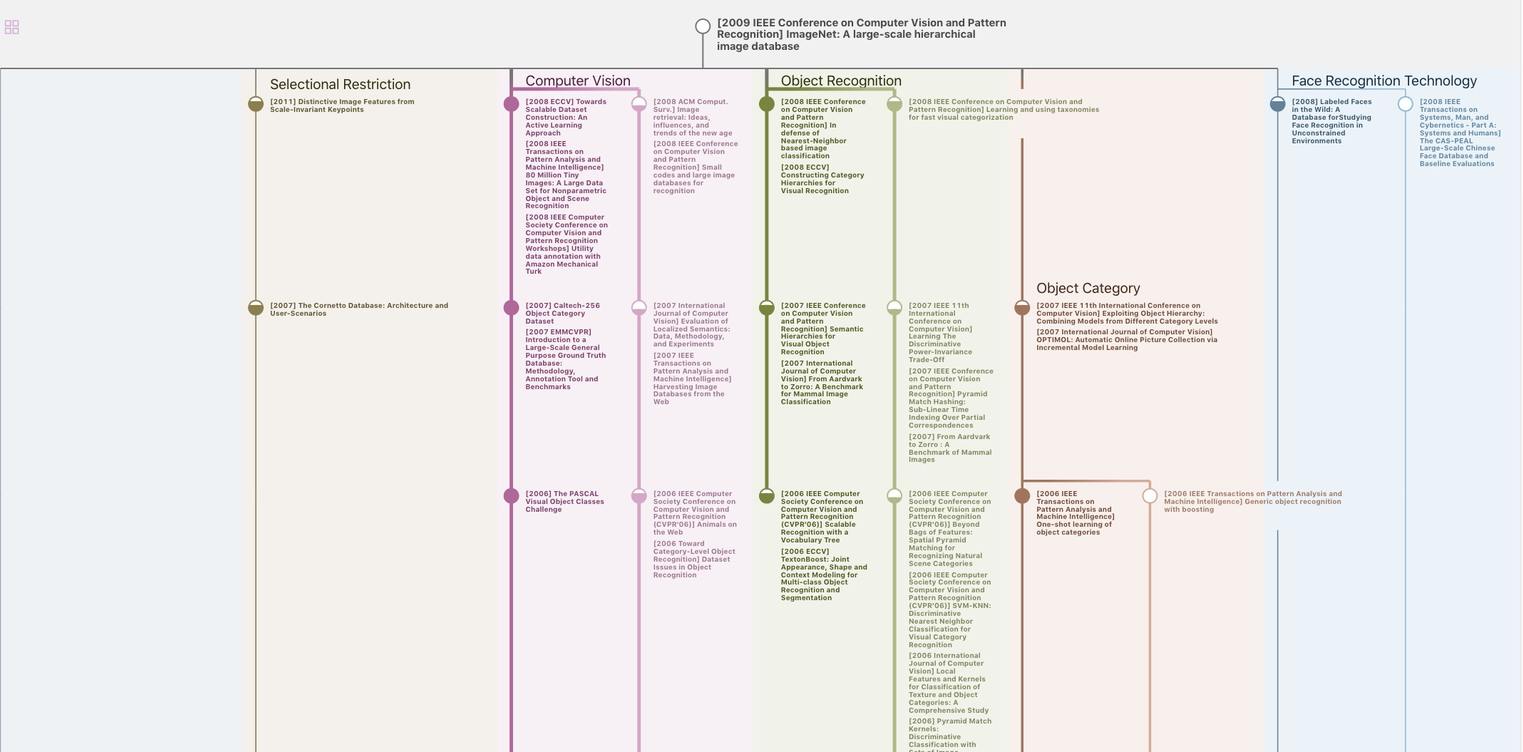
生成溯源树,研究论文发展脉络
Chat Paper
正在生成论文摘要