Deep Graph Reinforcement Learning for Mobile Edge Computing: Challenges and Solutions
IEEE Network(2024)
摘要
With the increasing Quality of Service (QoS) requirements of the Internet of Things (IoT), Mobile Edge Computing (MEC) has undoubtedly become a new paradigm for locating various resources in the proximity of User Equipment (UE) to alleviate the workload of backbone IoT networks. Deep Reinforcement Learning (DRL) has gained widespread popularity as a preferred methodology, primarily due to its capability to guide each User Equipment (UE) in making appropriate decisions within dynamic environments. However, traditional DRL algorithms cannot fully exploit the relationship between devices in the MEC graph. Here, we point out two typical IoT scenarios, i.e., task offloading decision-making when dependent tasks in resource-constrained Edge Servers (ESs) are generated in UEs and orchestration of cross-ESs distributed service, where the system cost is minimized by orchestrating hierarchical networks. To further enhance the performance of DRL, Graph Neural Networks (GNNs) and their variants provide promising generalization ability to wide IoT scenarios. We accordingly give concrete solutions for the above two typical scenarios, namely, Graph Neural Networks-Proximal Policy Optimization (GNN-PPO) and Graph Neural Networks-Meta Reinforcement Learning (GNN-MRL), which combine GNN with a popular Actor-Critic scheme and newly developed MRL. Finally, we point out four worthwhile research directions for exploring GNN and DRL for AI-empowered MEC environments.
更多查看译文
关键词
Graph Reinforcement Learning,Mobil Edge Computing,Internet of Things,Task Offloading,Resource Orchestration
AI 理解论文
溯源树
样例
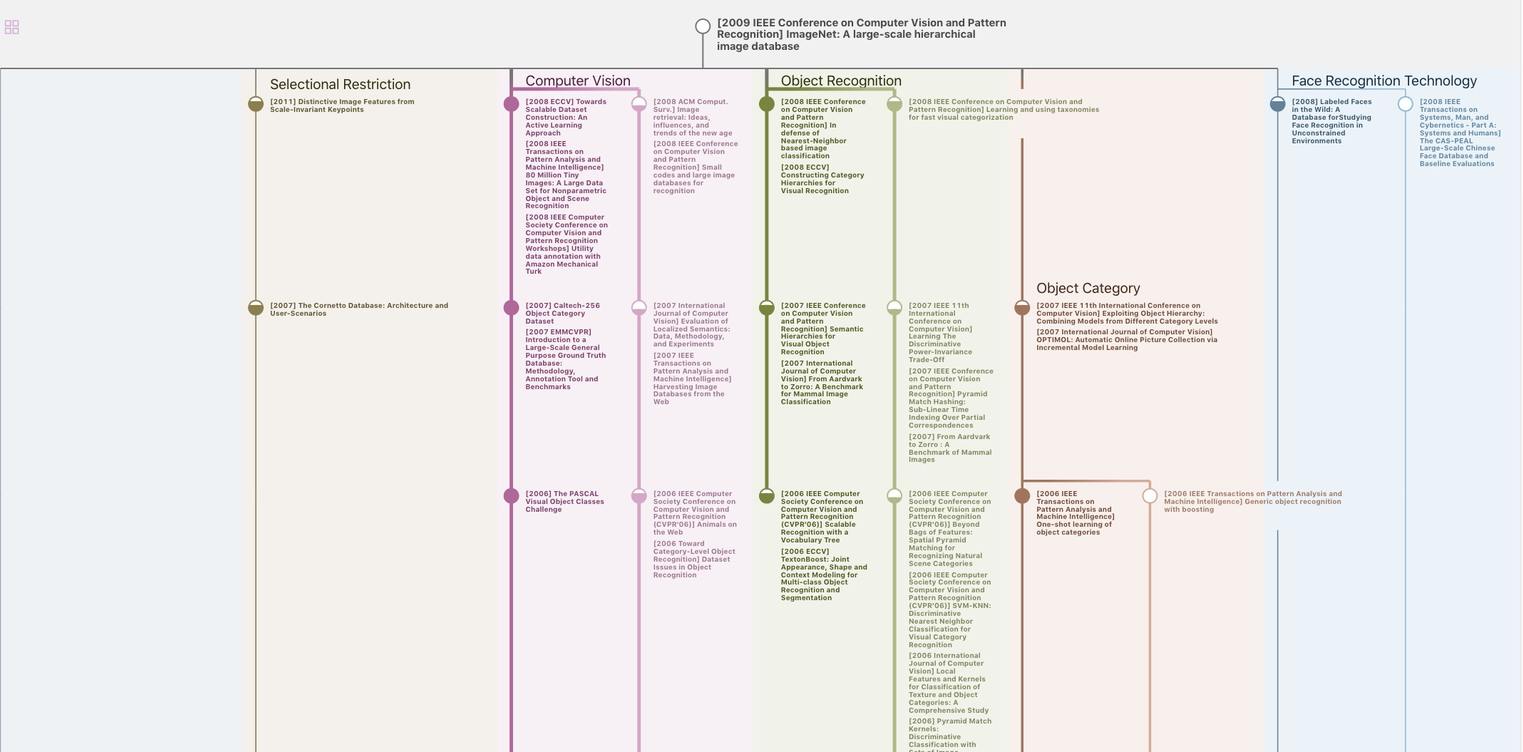
生成溯源树,研究论文发展脉络
Chat Paper
正在生成论文摘要