Optimizing Contingency Management with Reinforcement Learning.
medRxiv : the preprint server for health sciences(2024)
摘要
Contingency Management (CM) is a psychological treatment that aims to change behavior with financial incentives. In substance use disorders (SUDs), deployment of CM has been enriched by longstanding discussions around the cost-effectiveness of prized-based and voucher-based approaches. In prize-based CM, participants earn draws to win prizes, including small incentives to reduce costs, and the number of draws escalates depending on the duration of maintenance of abstinence. In voucher-based CM, participants receive a predetermined voucher amount based on specific substance test results. While both types have enhanced treatment outcomes, there is room for improvement in their cost-effectiveness: the voucher-based system requires enduring financial investment; the prize-based system might sacrifice efficacy. Previous work in computational psychiatry of SUDs typically employs frameworks wherein participants make decisions to maximize their expected compensation. In contrast, we developed new frameworks that clinical decision-makers choose actions, CM structures, to reinforce the substance abstinence behavior of participants. We consider the choice of the voucher or prize to be a sequential decision, where there are two pivotal parameters: the prize probability for each draw and the escalation rule determining the number of draws. Recent advancements in Reinforcement Learning, more specifically, in off-policy evaluation, afforded techniques to estimate outcomes for different CM decision scenarios from observed clinical trial data. We searched CM schemas that maximized treatment outcomes with budget constraints. Using this framework, we analyzed data from the Clinical Trials Network to construct unbiased estimators on the effects of new CM schemas. Our results indicated that the optimal CM schema would be to strengthen reinforcement rapidly in the middle of the treatment course. Our estimated optimal CM policy improved treatment outcomes by 32% while maintaining costs. Our methods and results have broad applications in future clinical trial planning and translational investigations on the neurobiological basis of SUDs.
更多查看译文
AI 理解论文
溯源树
样例
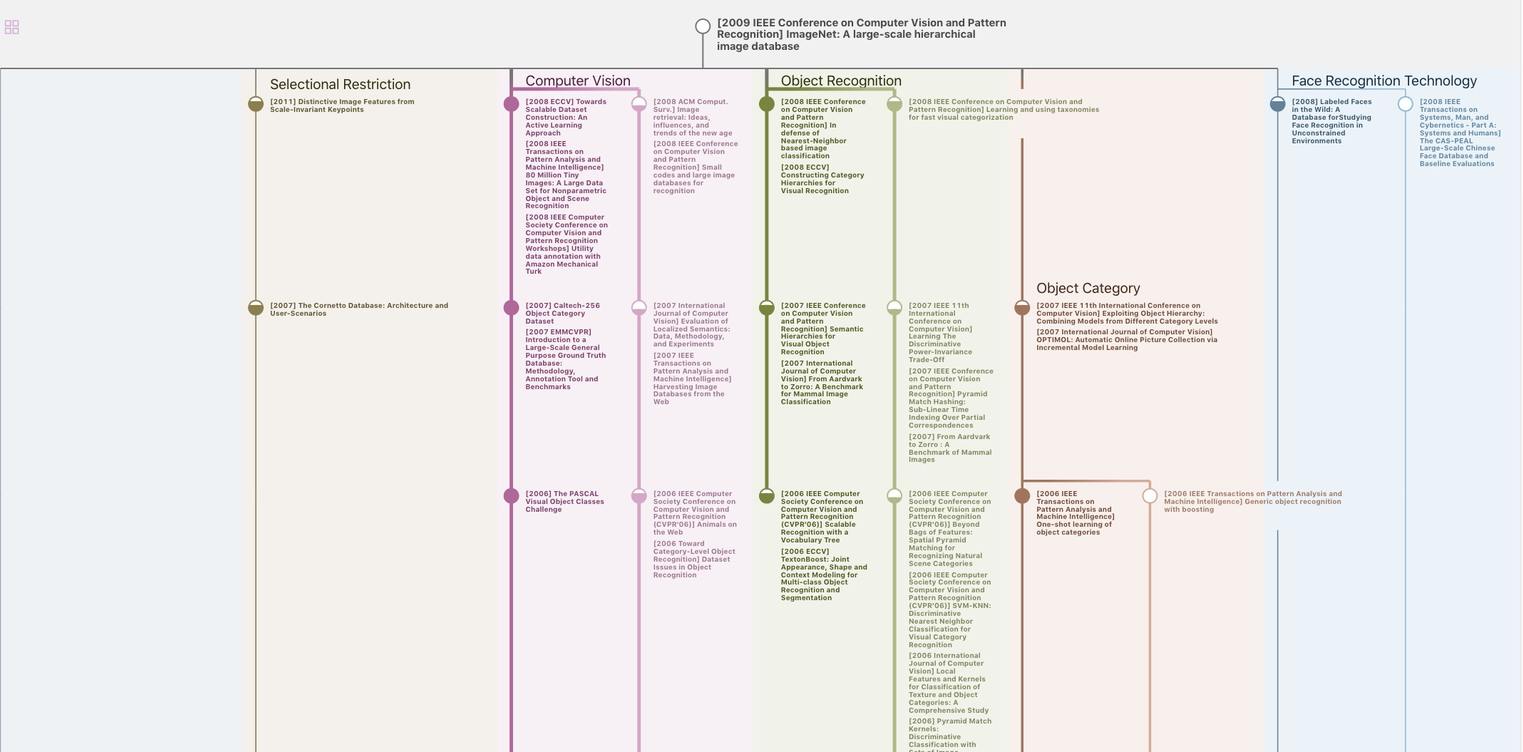
生成溯源树,研究论文发展脉络
Chat Paper
正在生成论文摘要