Synchrotron radiation sparse-view CT artifact correction through deep learning neural networks
NONDESTRUCTIVE TESTING AND EVALUATION(2024)
摘要
Synchrotron radiation X-ray Computed Tomography(CT) imaging is an effective means of non-destructively revealing the internal structure of samples. To obtain high-quality reconstructed slices, the number of projections should be at least ${\pi \over 2}{N_{detector}}$pi 2Ndetector (${N_{detector}}$Ndetector is the number of detector pixels occupied by the sample diameter). Acquiring excessive projections leads to high radiation doses and long scanning time. Insufficient projections, falling below the Nyquist sampling criterion, result in significant streaking artifacts. This paper proposes a method that fuses the structural similarity index and the peak signal-to-noise ratio as a loss function of the Attention U-Net network to correct streaking artifacts. Moreover, a new deep learning framework based on the Transformer and convolutional neural network is also proposed to solve the artifact problem. At 200 and 400 views, the two proposed methods significantly improve the reconstructed slices' quality, qualitatively and quantitatively outperforming the classical streaking artifact correction method. The optimal sparsity ratio for sparse-view CT imaging is investigated: the Trans-attunet method corrects streaking artifacts at a 1/12 sparsity ratio to visualize large sample features, whereas ratios of 1/3 or 1/2 more effectively recover fine structures. The optimal sparsity ratio is contingent upon the trade-off between desired image quality and imaging efficiency.
更多查看译文
关键词
Synchrotron radiation X-ray imaging,Computed tomography,Sparse-view CT,Deep learning,Nondestructive testing
AI 理解论文
溯源树
样例
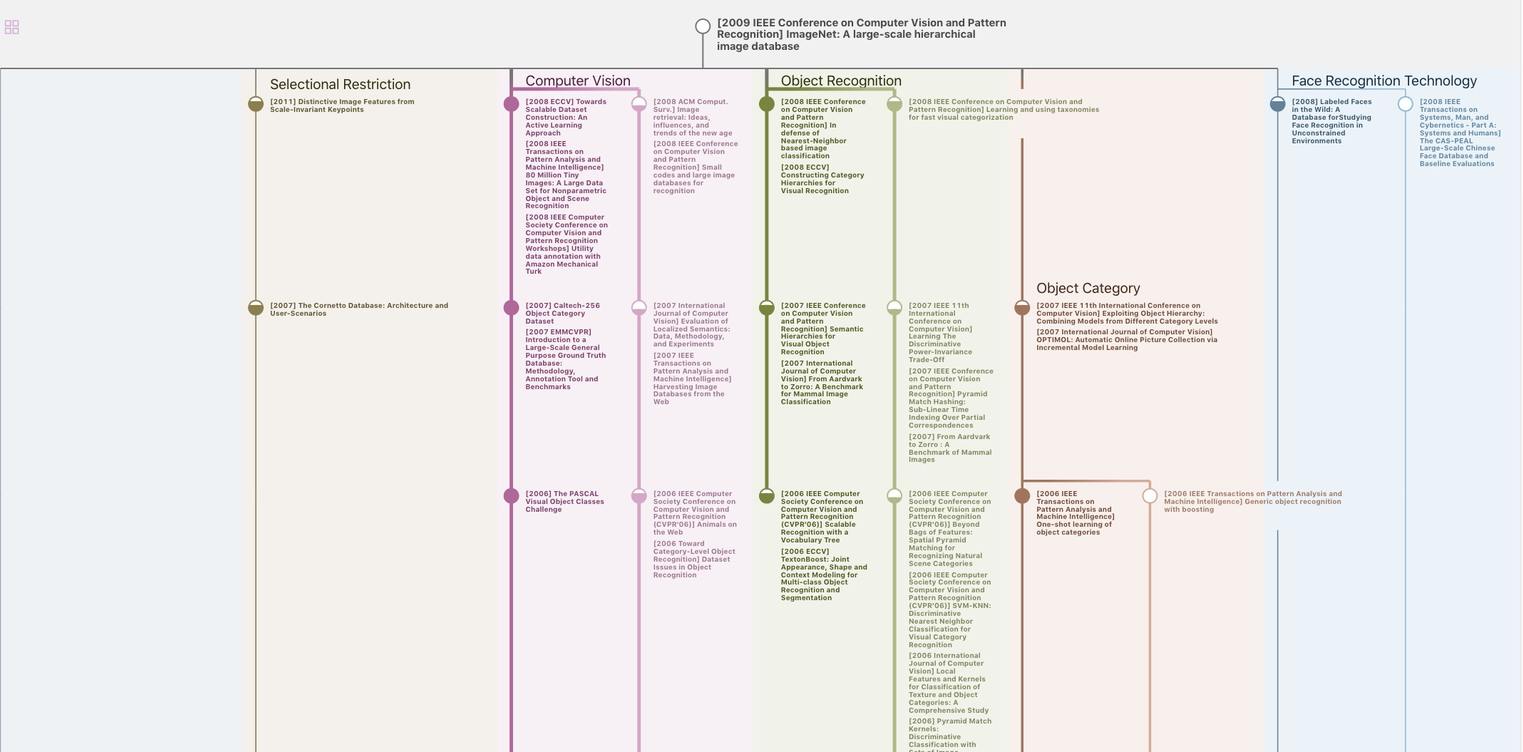
生成溯源树,研究论文发展脉络
Chat Paper
正在生成论文摘要