conteNXt: A Graph-Based Approach to Assimilate Content and Context for Event Detection in OSN
IEEE TRANSACTIONS ON COMPUTATIONAL SOCIAL SYSTEMS(2024)
摘要
Social networks are rapidly expanding due to their imperative role in disseminating information in a split second, emerging as the primary source for breaking news. As a result, the rich, user-generated information entices researchers to delve deeper and extract valuable insights. Event detection in online social networks (OSNs) is a research problem that has shifted researchers attention from traditional news media to online social media data. Event detection in OSNs is an automated process, addressing the impractical task of manually filtering potential events from vast amounts of online data. Unfortunately, the informality and semantic sparsity of online social networking text pose significant challenges to the event detection task. To this end, we present an approach named conteNXt for detecting events from Twitter (currently "X") posts (also known as Tweets). To handle large amounts of data, the proposed method divides tweets into bins and uses postprocessing methods to extract bursty keyphrases. These keyphrases are then used to generate a weighted keyphrase graph using the Word2Vec model. Finally, Markov clustering is employed to cluster and detect events in the bursty keyphrase graph. conteNXt is evaluated on the Event-Corpus2012 benchmark dataset and two additional datasets extracted from the archive, Archive2020 and Archive2021, using performance evaluation metrics: #events, precision, recall, and F1-score. The proposed approach outperforms state-of-the-art methods, including SEDTWik, Twevent, Sentence-BERT, MABED, EDED, CommunityINDICATOR, and EventX. Additionally, the proposed approach is capable of detecting vital events that are not identified by the aforementioned state-of-the-art methods.
更多查看译文
关键词
Event detection,Social networking (online),Blogs,Semantics,Feature extraction,Media,Clustering algorithms,Bursty keyphrases,event detection,Markov clustering (MCL),online social network (OSN),social network analysis
AI 理解论文
溯源树
样例
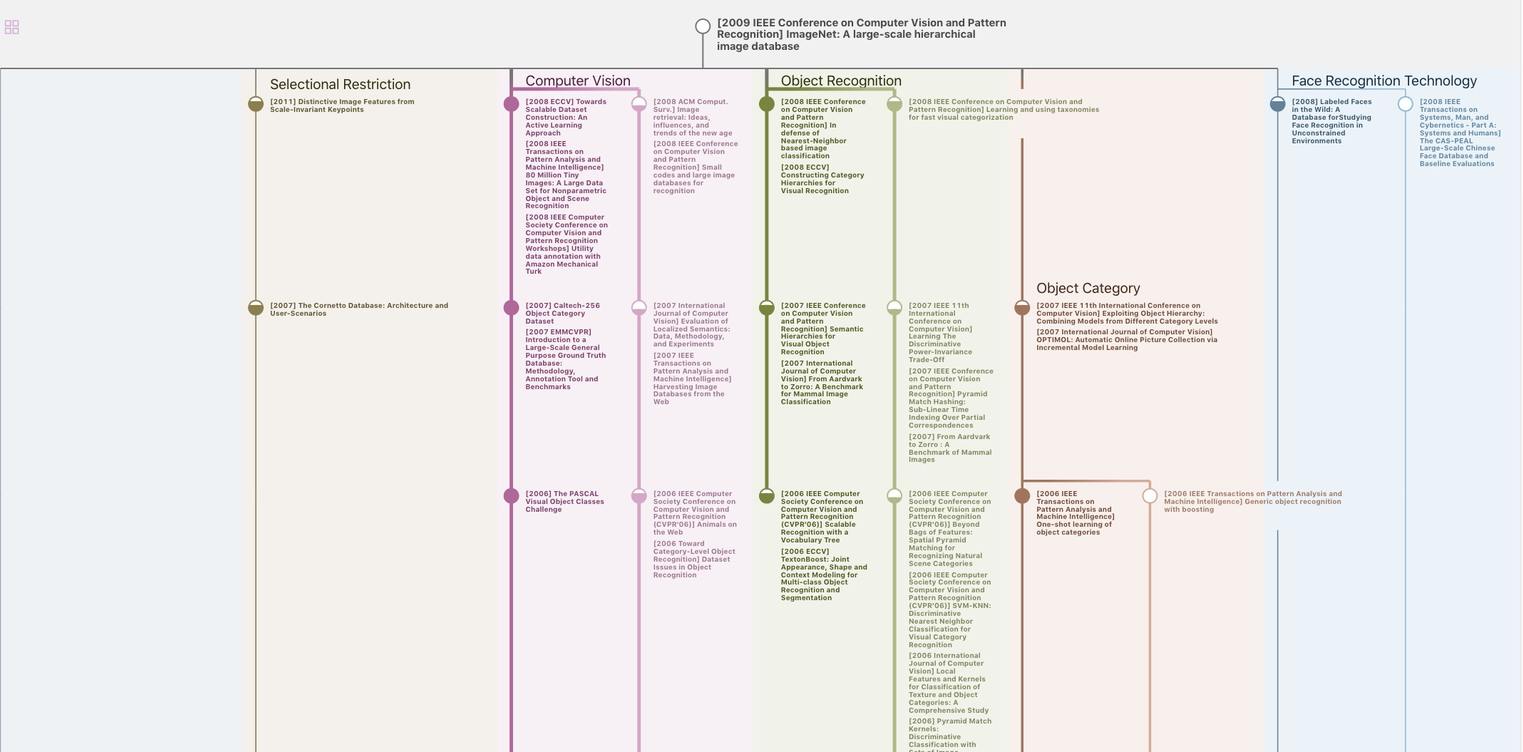
生成溯源树,研究论文发展脉络
Chat Paper
正在生成论文摘要