An Objective Function-Based Clustering Algorithm with a Closed-Form Solution and Application to Reference Interval Estimation in Laboratory Medicine
ALGORITHMS(2024)
摘要
Clustering algorithms are usually iterative procedures. In particular, when the clustering algorithm aims to optimise an objective function like in k-means clustering or Gaussian mixture models, iterative heuristics are required due to the high non-linearity of the objective function. This implies higher computational costs and the risk of finding only a local optimum and not the global optimum of the objective function. In this paper, we demonstrate that in the case of one-dimensional clustering with one main and one noise cluster, one can formulate an objective function, which permits a closed-form solution with no need for an iteration scheme and the guarantee of finding the global optimum. We demonstrate how such an algorithm can be applied in the context of laboratory medicine as a method to estimate reference intervals that represent the range of "normal" values.
更多查看译文
关键词
single-pass clustering,noise clustering,closed-form solution,reference interval
AI 理解论文
溯源树
样例
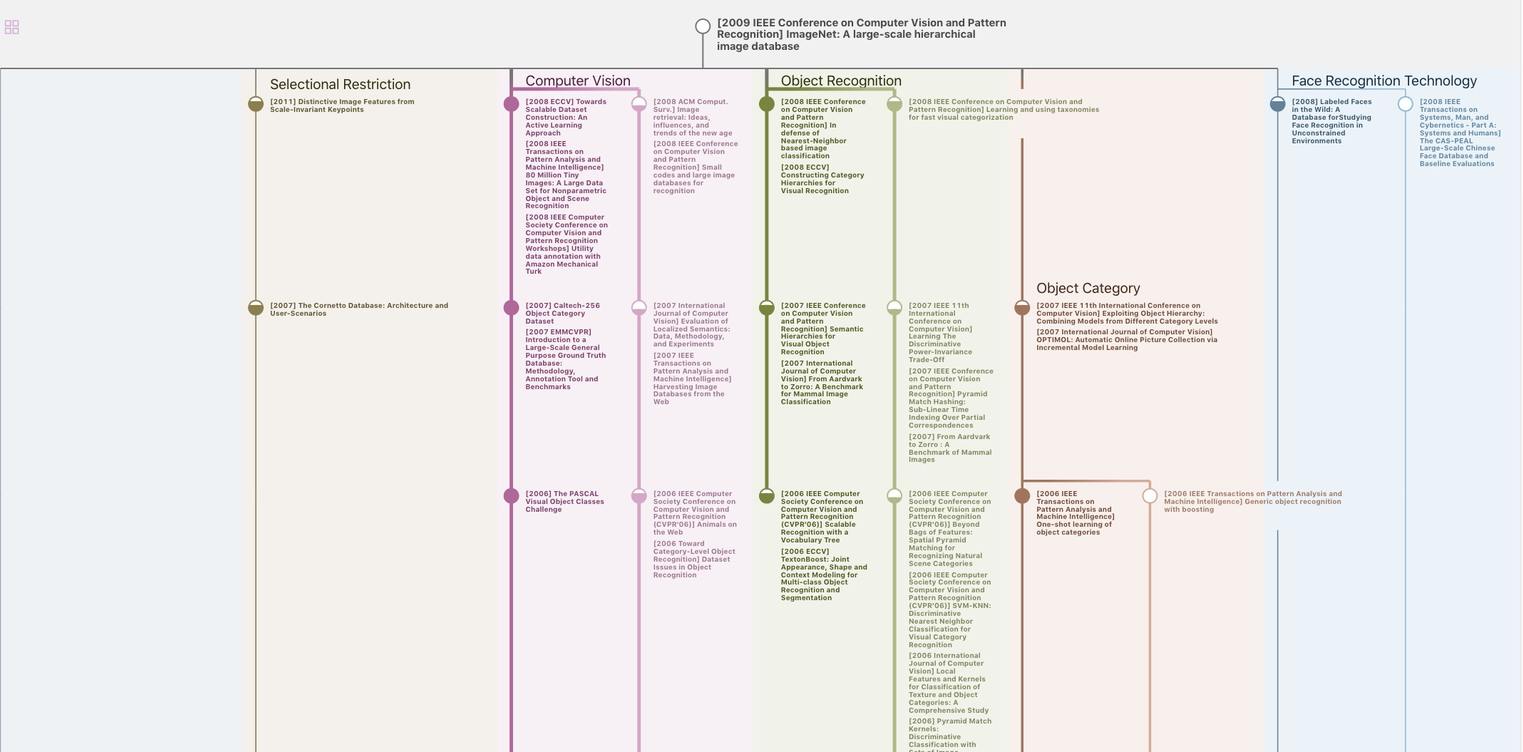
生成溯源树,研究论文发展脉络
Chat Paper
正在生成论文摘要