Emulation of Cloud Microphysics in a Climate Model
JOURNAL OF ADVANCES IN MODELING EARTH SYSTEMS(2024)
摘要
We present a machine learning based emulator of a microphysics scheme for condensation and precipitation processes (Zhao-Carr) used operationally in a global atmospheric forecast model (FV3GFS). Our tailored emulator architecture achieves high skill (>= 94%) in predicting condensate and precipitation amounts and maintains low global-average bias (<= 4%) for 1 year of continuous simulation when replacing the Fortran scheme. The stability and success of this emulator stems from key design decisions. By separating the emulation of condensation and precipitation processes, we can better enforce physical priors such as mass conservation and locality of condensation, and the vertical dependence of precipitation falling downward, using specific network architectures. An activity classifier for condensation imitates the discrete-continuous nature of the Fortran microphysics outputs (i.e., tendencies are identically zero where the scheme is inactive, and condensate is zero where clouds are fully evaporated). A temperature-scaled conditional loss function ensures accurate condensate adjustments for a high dynamic range of cloud types (e.g., cold, low-condensate cirrus clouds or warm, condensate-rich clouds). Despite excellent overall performance, the emulator exhibits some deficiencies in the uppermost model levels, leading to biases in the stratosphere. The emulator also has short episodic skill dropouts in isolated grid columns and is computationally slower than the original Fortran scheme. Nonetheless, our challenges and strategies should be applicable to the emulation of other microphysical schemes. More broadly, our work demonstrates that with suitable physically motivated architectural choices, ML techniques can accurately emulate complex human-designed parameterizations of fast physical processes central to weather and climate models. In this study, we create computer code that uses machine learning to mimic a weather model's algorithm for handling how clouds form and rain falls. When used in the weather model to replace this algorithm, our machine learning code is highly accurate in simulations for a whole year. We achieve this by making smart code design choices. We split the code into two parts: one for cloud formation and one for rain and snow. This allows us to better build important aspects of these processes into the machine learning approach. For instance, clouds form where it is moist and evaporate when it gets dry, and rain and snow fall downward. Our code learns cloud behavior based on temperature to ensure it works both for cold, thin clouds high up in the sky and warm, thick clouds closer to the ground. Our work shows a path for suitably designed machine learning code to eventually replace important parts of weather and climate models, but also that this path still requires careful human design respecting known physical principles. We build an emulator to replace the Zhao-Carr Fortran microphysics scheme in FV3GFS The integrated emulator sustains high skill throughout a 1-year simulation Tailoring the ML architecture to the structure of the underlying scheme greatly improves the online behavior of the emulator
更多查看译文
关键词
physics emulation,machine learning,microphysics,atmospheric modeling,parameterization
AI 理解论文
溯源树
样例
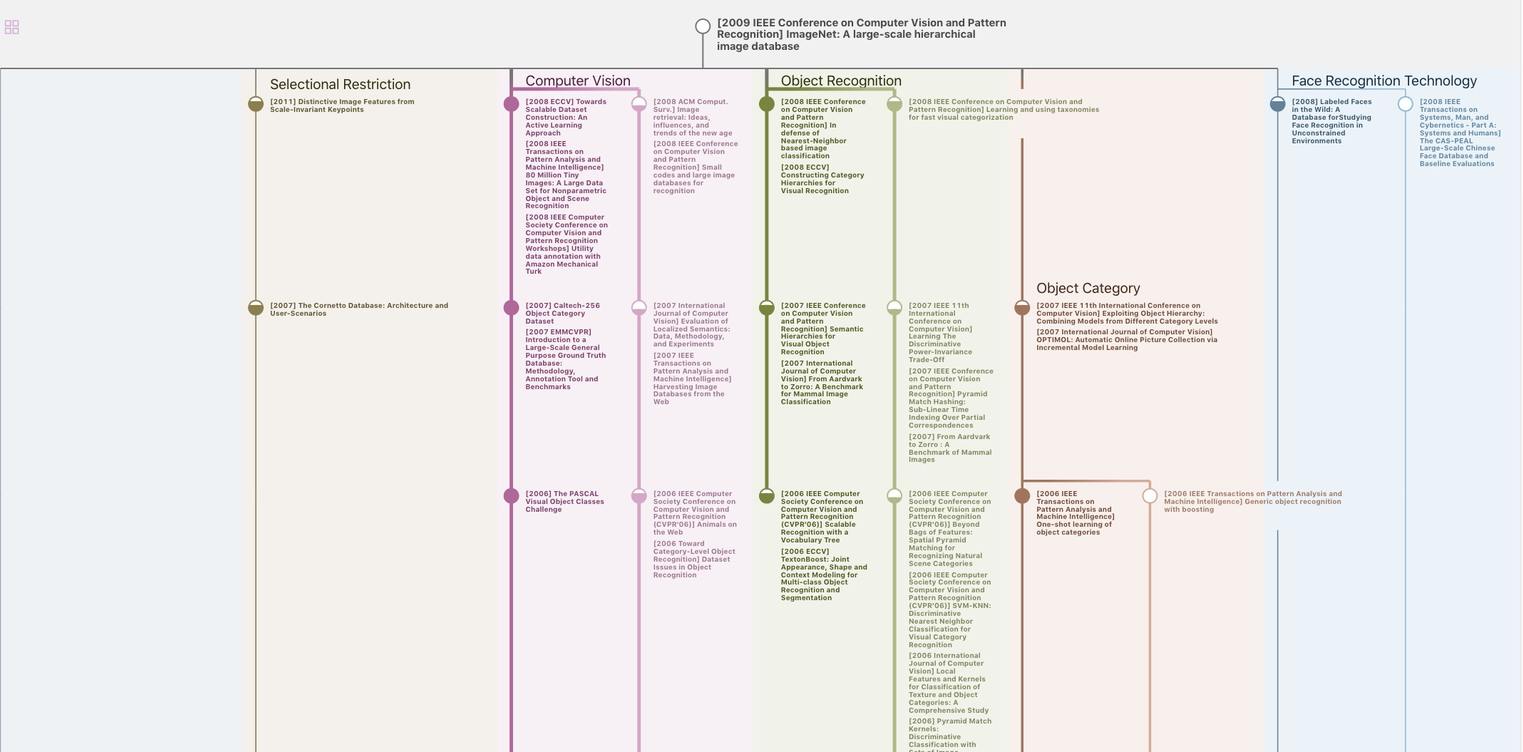
生成溯源树,研究论文发展脉络
Chat Paper
正在生成论文摘要