Optimization of Random Feature Method in the High-Precision Regime
Communications on Applied Mathematics and Computation(2024)
摘要
Machine learning has been widely used for solving partial differential equations (PDEs) in recent years, among which the random feature method (RFM) exhibits spectral accuracy and can compete with traditional solvers in terms of both accuracy and efficiency. Potentially, the optimization problem in the RFM is more difficult to solve than those that arise in traditional methods. Unlike the broader machine-learning research, which frequently targets tasks within the low-precision regime, our study focuses on the high-precision regime crucial for solving PDEs. In this work, we study this problem from the following aspects: (i) we analyze the coefficient matrix that arises in the RFM by studying the distribution of singular values; (ii) we investigate whether the continuous training causes the overfitting issue; (iii) we test direct and iterative methods as well as randomized methods for solving the optimization problem. Based on these results, we find that direct methods are superior to other methods if memory is not an issue, while iterative methods typically have low accuracy and can be improved by preconditioning to some extent.
更多查看译文
关键词
Random feature method (RFM),Partial differential equation (PDE),Least-squares problem,Direct method,Iterative method,Primary 65F30,65F20,65Y05,65-04
AI 理解论文
溯源树
样例
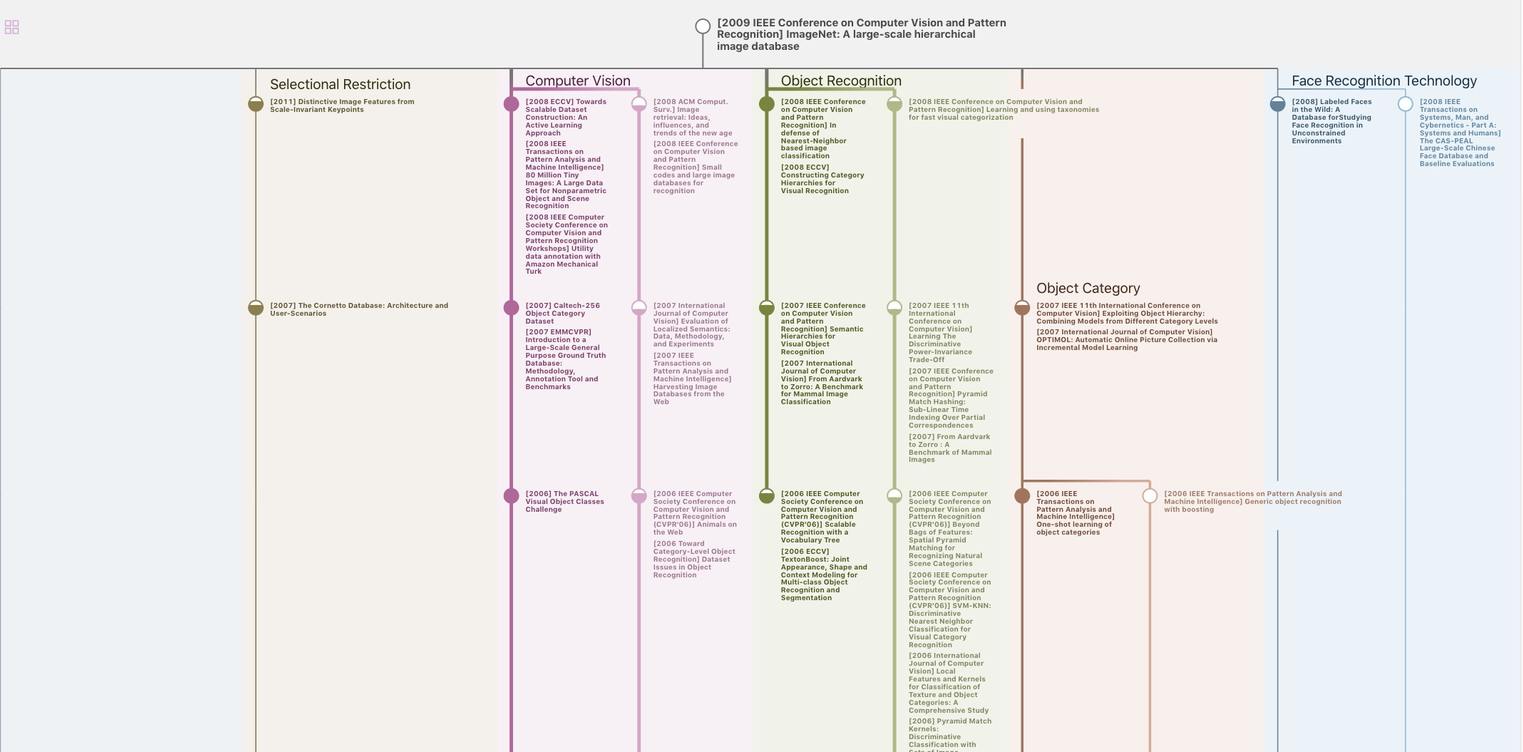
生成溯源树,研究论文发展脉络
Chat Paper
正在生成论文摘要