Enhanced Prairie Dog Optimization with Levy Flight and Dynamic Opposition-Based Learning for Global Optimization and Engineering Design Problems
Neural Computing and Applications(2024)
摘要
This study proposes a new prairie dog optimization algorithm version called EPDO. This new version aims to address the issues of premature convergence and slow convergence that were observed in the original PDO algorithm. To improve performance, several modifications are introduced in EPDO. First, a dynamic opposite learning strategy is employed to increase the diversity of the population and prevent premature convergence. This strategy helps the algorithm avoid falling into local optima and promotes global optimization. Additionally, the Lévy dynamic random walk technique is utilized in EPDO. This modified Lévy flight with random walk reduces the algorithm’s running time for the test function’s ideal value, accelerating its convergence. The proposed approach is evaluated using 33 benchmark problems from CEC 2017 and compared against seven other comparative techniques: GWO, MFO, ALO, WOA, DA, SCA, and RSA. Numerical results demonstrate that EPDO produces good outcomes and performs well in solving benchmark problems. To further validate the results and assess reliability, the authors employ average rank tests, the measurement of alternatives, and ranking according to the compromise solution (MARCOS) method, as well as a convergence report of EPDO and other algorithms. Furthermore, the effectiveness of the EPDO algorithm is demonstrated by applying it to five design problems. The results indicate that EPDO achieves impressive outcomes and proves its capability to address practical issues. The algorithm performs well in solving benchmark and practical design problems, as supported by the numerical results and validation methods used in the study.
更多查看译文
关键词
Enhanced prairie dog optimization,Lévy distribution,Dynamic opposition-based learning,Global optimization,Engineering design problem,MARCOS MCDM method,Benchmark problems
AI 理解论文
溯源树
样例
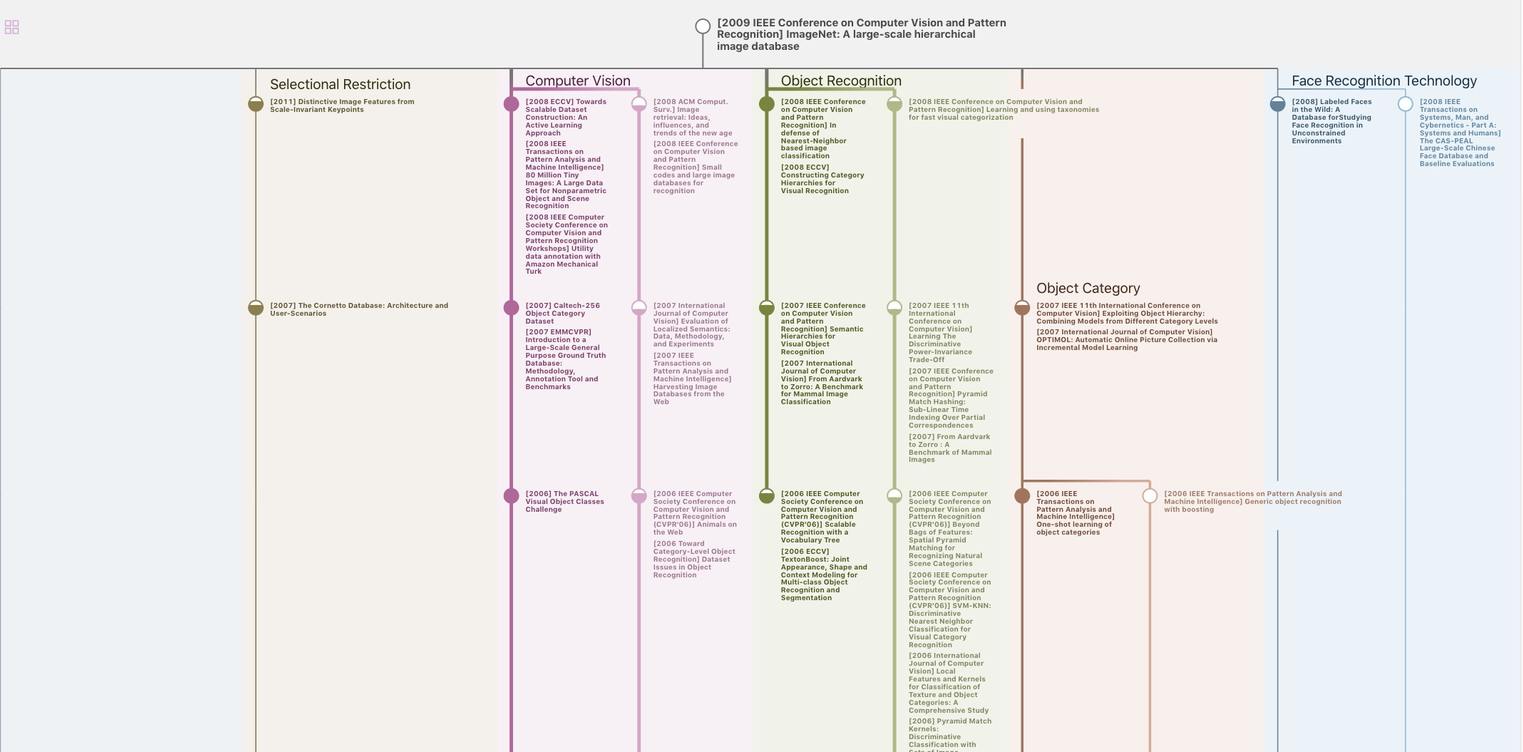
生成溯源树,研究论文发展脉络
Chat Paper
正在生成论文摘要