Predicting intensive care unit discharges of neurosurgery patients: A machine learning modeling
Journal of Critical Care(2024)
摘要
Due to the increased patient volumes within health systems, it has become increasingly important to free up available beds across all departments of hospitals including ED and ICU. Especially during pandemics like COVID-19, the ICUs typically operate at near full capacity levels which makes effective management of ICU beds more critical [1]. An effective capacity management can only be possible by accurately predicting patient discharge times and synchronizing patient flows. The objective of this study to use data extracted from a hospital's electronic health records (EHR) to predict whether a patient will be discharged from the ICU within 24 h.
Methods
The research uses an open access dataset (MIMIC) obtained from the EHR system of Beth Israel Deaconess Medical Center (BIDMC), where patient information and encounter dates are masked to protect confidentiality. The dataset used in this study was obtained by filtering for postoperative neurosurgery intensive care patients who had undergone a craniotomy procedure during their hospitalization. For those patients, several explanatory features that potentially impact ICU discharges are generated. Using these features as input to a feed-forward neural network architecture, we predict the likelihood that a patient will be discharged within the next 24 h.
Results
After filtering for patients who had undergone a craniotomy procedure, 2781 unique patients and 3020 hospital admissions of these patients were included in our dataset. For this patient cohort, the mean length of ICU stay is 10.3 days (median: 6 days). Each patient's data is recorded at 6-h intervals resulting in 133,792 observations, in total. From the clinical and demographic data, 54 features are generated for each observation. Clinical features (47) include indicators of the patient's clinical conditions such as infection and pulmonary embolism, which may affect the intensive care hospitalization process (fever, pulse, intubation, etc.). Remaining 7 features include the demographic characteristics of the patient such as age, gender, and the time since ICU admission. The neural network model trained predicts whether a neurosurgery intensive care patients will be discharged within 24 h with an AUC of 82%.
Conclusion
The study demonstrated that a machine learning model can predict whether a patient will be discharged in next 24 h with high accuracy. Machine learning models developed with big data sets can assist clinicians and hospital management units in predicting inpatient movements and thus in planning hospital resources more accurately.
更多查看译文
关键词
Neurosurgery intensive care,Machine learning,Big data,Mathematical modeling
AI 理解论文
溯源树
样例
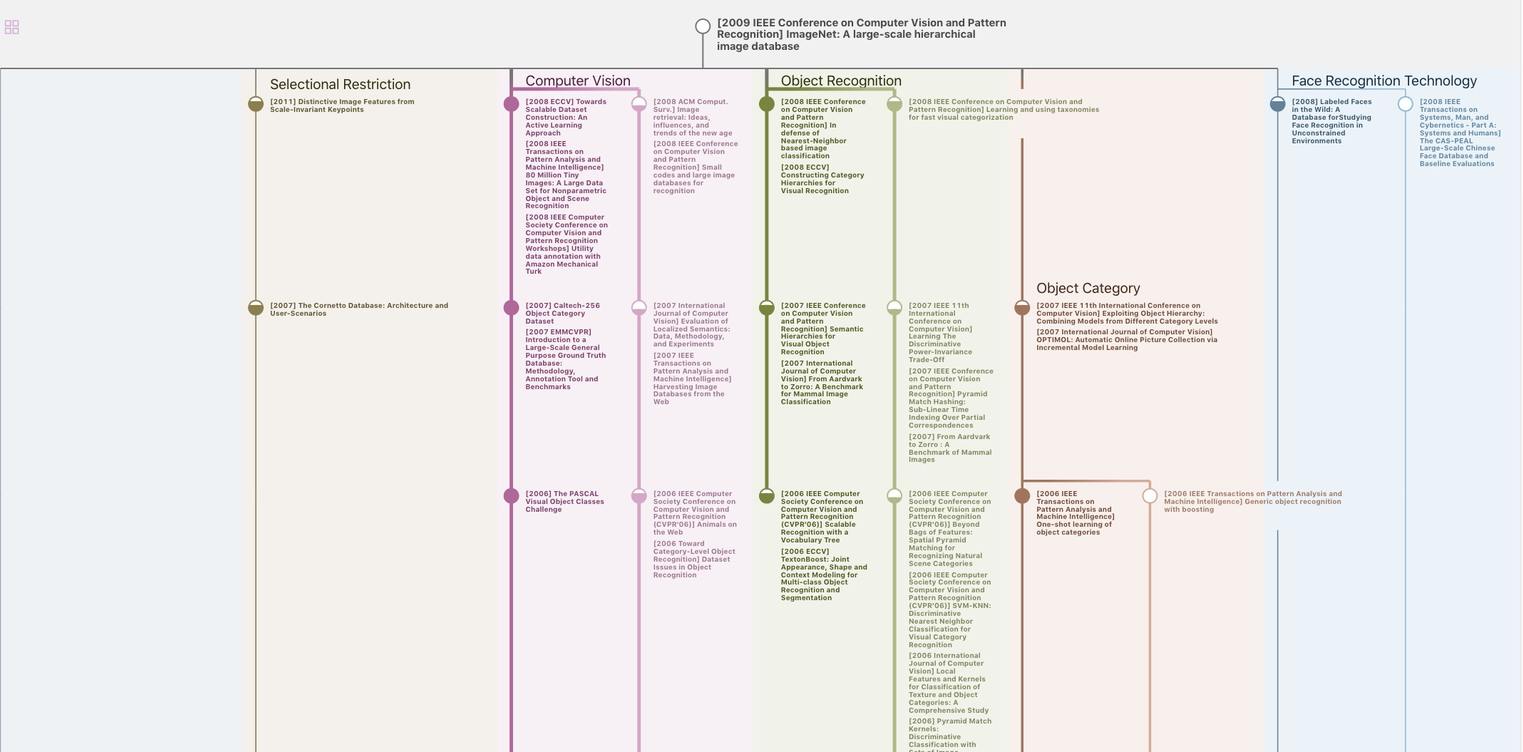
生成溯源树,研究论文发展脉络
Chat Paper
正在生成论文摘要