Comparative Analysis of Three Modeling Approaches for Predicting Pavement Conditions
TRANSPORTATION RESEARCH RECORD(2024)
摘要
States, counties, and municipalities rely on pavement performance models to forecast future pavement conditions in their jurisdictions. Accurate prediction is essential for budget planning and the identification of candidates for rehabilitation. This study compares the performance of three different approaches to predict pavement conditions: (1) a sigmoidal or S-shaped curve; (2) a grey system model (GM); and (3) Gaussian process regression (GPR). All three models are trained on the same dataset for two types of pavements, asphalt with and without overlay and composite (i.e., asphalt over concrete), with each having two types of maintenance activities frequently performed by the South Carolina Department of Transportation. The trained models are then applied to separate test datasets. The prediction results indicate that GPR is the best model in three out of four cases using mean absolute error as the performance metric; the exception is the case involving the prediction of pavement serviceability index for asphalt pavement with mill-and-replace 1-2 in. + overlay 400 pounds per square yard rehabilitation treatment. When using mean absolute percentage error and root mean squared error as the performance metrics, the GPR model is the better model for predicting conditions of composite pavements, while the GM ( 1 , 1 ) model is the better model for predicting conditions of asphalt pavements.
更多查看译文
关键词
infrastructure,infrastructure management and system preservation,pavement management systems,performance modeling
AI 理解论文
溯源树
样例
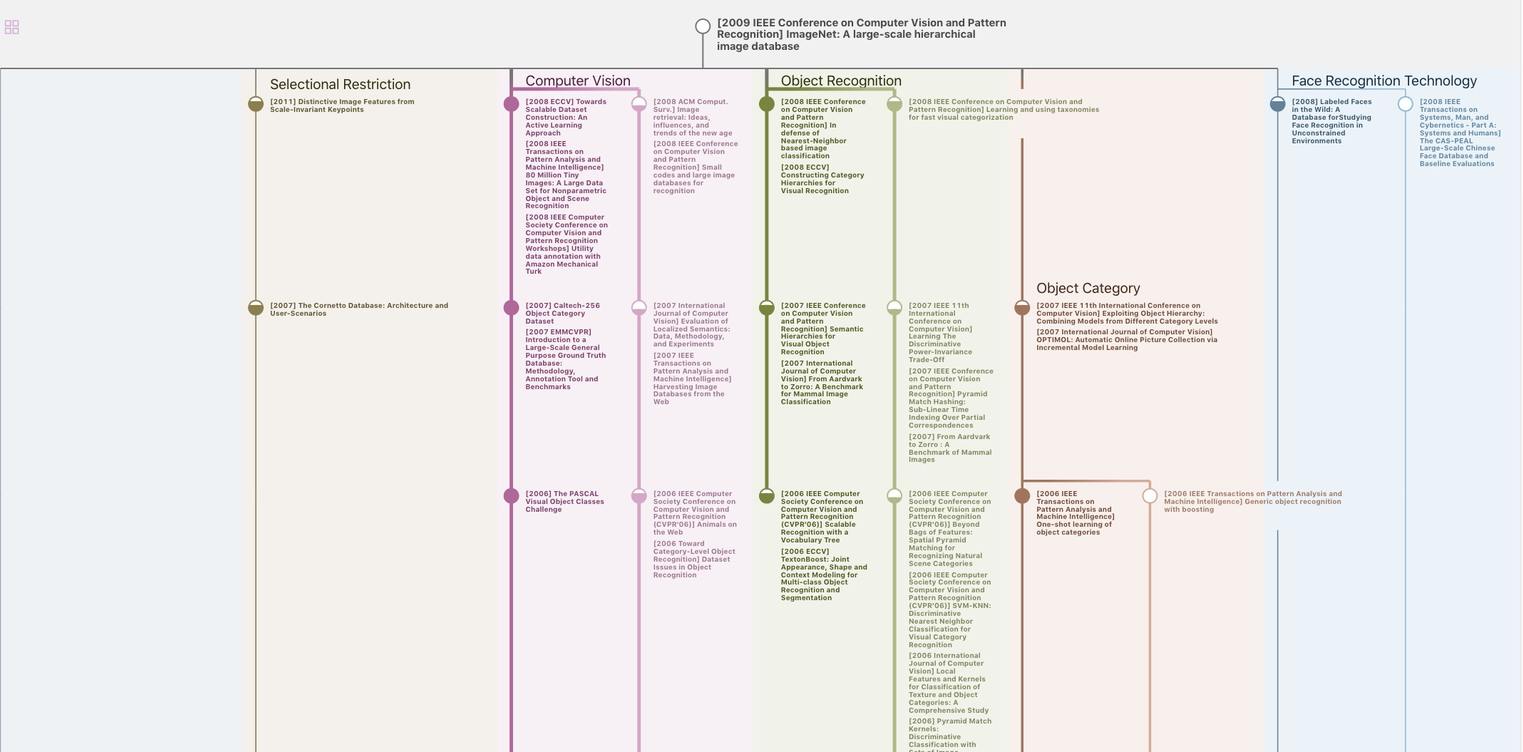
生成溯源树,研究论文发展脉络
Chat Paper
正在生成论文摘要