Learning optimal spatial subsampling for single-channel ultrasound imaging
Science Talks(2024)
摘要
Traditional ultrasound synthetic aperture imaging relies on closely spaced measurement positions, where the pitch size is smaller than half the ultrasound wavelength. While this approach achieves high-quality images, it necessitates the storage of large data sets and an extended measurement time. To address these issues, there is a burgeoning interest in exploring effective subsampling techniques. Recently, Deep Probabilistic Subsampling (DPS) has emerged as a feasible approach for designing selection matrices for multi-channel systems. In this paper, we address spatial subsampling in single-channel ultrasound imaging for Nondestructive Testing (NDT) applications. To accomplish a model-based data-driven spatial subsampling approach within the DPS framework that allows for the optimal selection of sensing positions on a discretized grid, it is crucial to build an adequate signal model and design an adapted network architecture with a reasonable cost function. The reconstructed image quality is then evaluated through simulations, showing that the presented subsampling pattern approaches the performance of fully sampling and substantially outperforms uniformly spatial subsampling in terms of signal recovery quality.
更多查看译文
关键词
Machine learning for signal processing,Signal subsampling,Sparse signal recovery,Ultrasound nondestructive testing
AI 理解论文
溯源树
样例
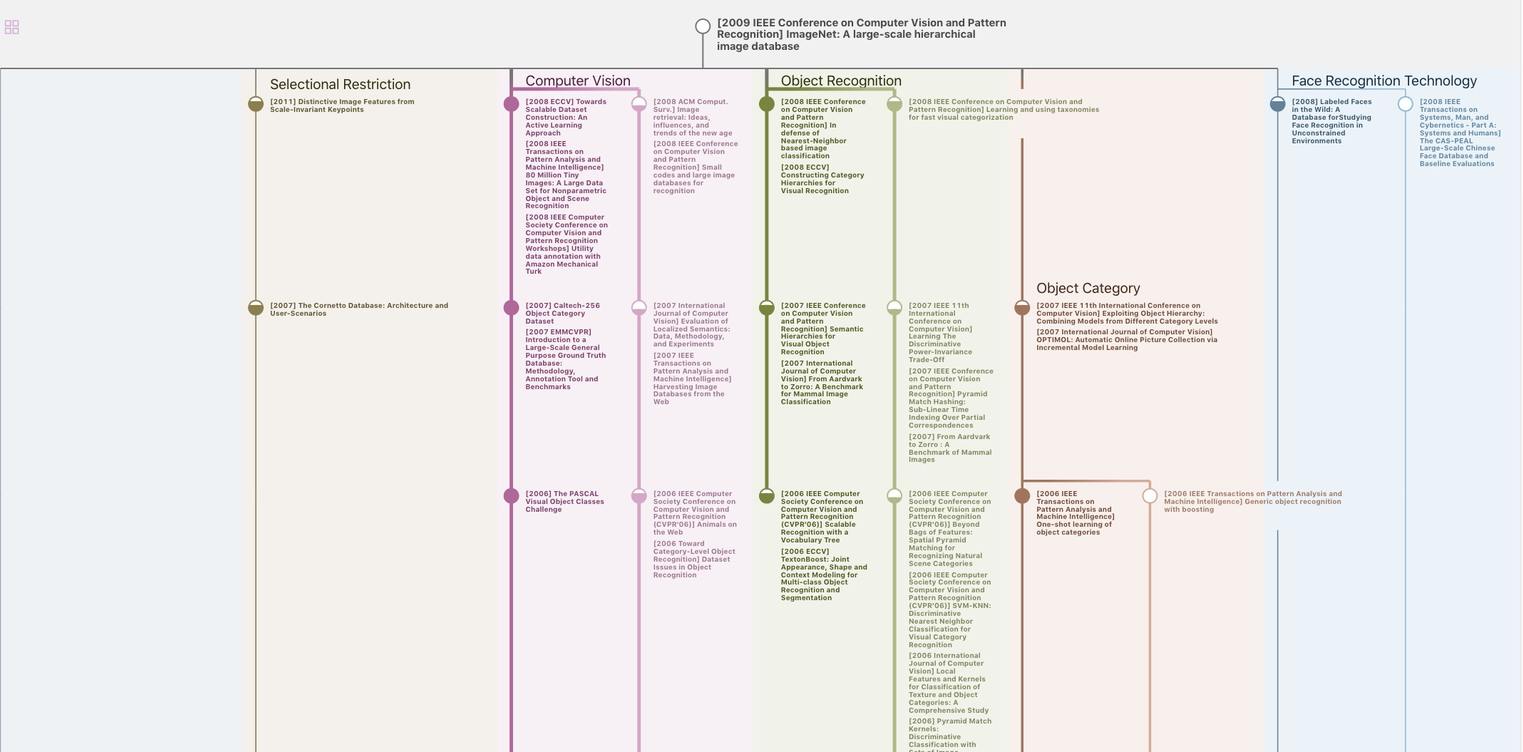
生成溯源树,研究论文发展脉络
Chat Paper
正在生成论文摘要