Prediction of Severe Obstructive Coronary Artery Disease Using Radiomic Features from Resting Cardiac Ultrasound Images: A Pilot Study
crossref(2024)
摘要
Background: Although cardiac ultrasound is frequently performed in patients with chest pain, the probability of obstructive coronary artery disease (CAD) cannot be quantified. We investigated the potential of cardiac ultrasound radiomics (ultrasomics) to identify obstructive CAD using limited echocardiography frames, suitable for cardiac point-of-care ultrasound evaluation. Methods: In total, 333 patients who were either healthy controls (n=30), undergoing invasive coronary procedures (n=113), or coronary CT angiography (n=190) were divided into two temporally distinct training (n=271) and testing (n=62) cohorts. Machine learning models were developed using ultrasomics for predicting severe CAD (stenosis >70%) and compared with regional LV wall motion abnormalities (RWMA). Results: In total, 94 (28.2%) patients had severe CAD with 50 (15.0%) having high-risk CAD defined as left main stenosis >50% (n=11), multivessel CAD (n=43), or 100% occlusion (n=20). The ultrasomics model was superior to RWMA for predicting severe CAD [area under the receiver operating curve (AUC) of 0.80 (95% confidence interval [CI]: 0.74 to 0.86) vs. 0.67 (95% CI: 0.61-0.72), p=0.0014] in the training set and [0.77 (95% CI: 0.64-0.90) vs. 0.70 (95% CI: 0.56-0.81), p=0.24] in the test set, respectively. The model also predicted high-risk CAD with an AUC of 0.84 (95% CI: 0.77-0.90) in the training set and 0.70 (95% CI: 0.48-0.88) in the test set. A combination of ultrasomics with RWMA showed incremental value over RWMA alone for predicting severe CAD. Conclusions: Cardiac ultrasomic features extracted from limited echocardiography views can aid the development of machine learning models to predict the presence of severe obstructive CAD.
### Competing Interest Statement
Dr. Sengupta is a consultant to Heart Sciences and RCE Technologies. Dr. Yanamala is a consultant to Research Spark Hub, Turnkey Learning LLC & TurnKey Insights (I) Pvt. Ltd. All other authors have no relationships relevant to the contents of this poster to disclose.
### Funding Statement
This work was supported by a National Science Foundation grant (Grant Number: 2125872).
### Author Declarations
I confirm all relevant ethical guidelines have been followed, and any necessary IRB and/or ethics committee approvals have been obtained.
Yes
The details of the IRB/oversight body that provided approval or exemption for the research described are given below:
Human Research Protection Program (HRPP), Institutional Review Board (IRB) of Robert Wood Johnson University Hospital (RWJBH), New Brunswick, NJ, USA. Waived or exempt with category 4(iii).
I confirm that all necessary patient/participant consent has been obtained and the appropriate institutional forms have been archived, and that any patient/participant/sample identifiers included were not known to anyone (e.g., hospital staff, patients or participants themselves) outside the research group so cannot be used to identify individuals.
Yes
I understand that all clinical trials and any other prospective interventional studies must be registered with an ICMJE-approved registry, such as ClinicalTrials.gov. I confirm that any such study reported in the manuscript has been registered and the trial registration ID is provided (note: if posting a prospective study registered retrospectively, please provide a statement in the trial ID field explaining why the study was not registered in advance).
Yes
I have followed all appropriate research reporting guidelines, such as any relevant EQUATOR Network research reporting checklist(s) and other pertinent material, if applicable.
Yes
All data produced in the present study are available upon reasonable request to the authors.
更多查看译文
AI 理解论文
溯源树
样例
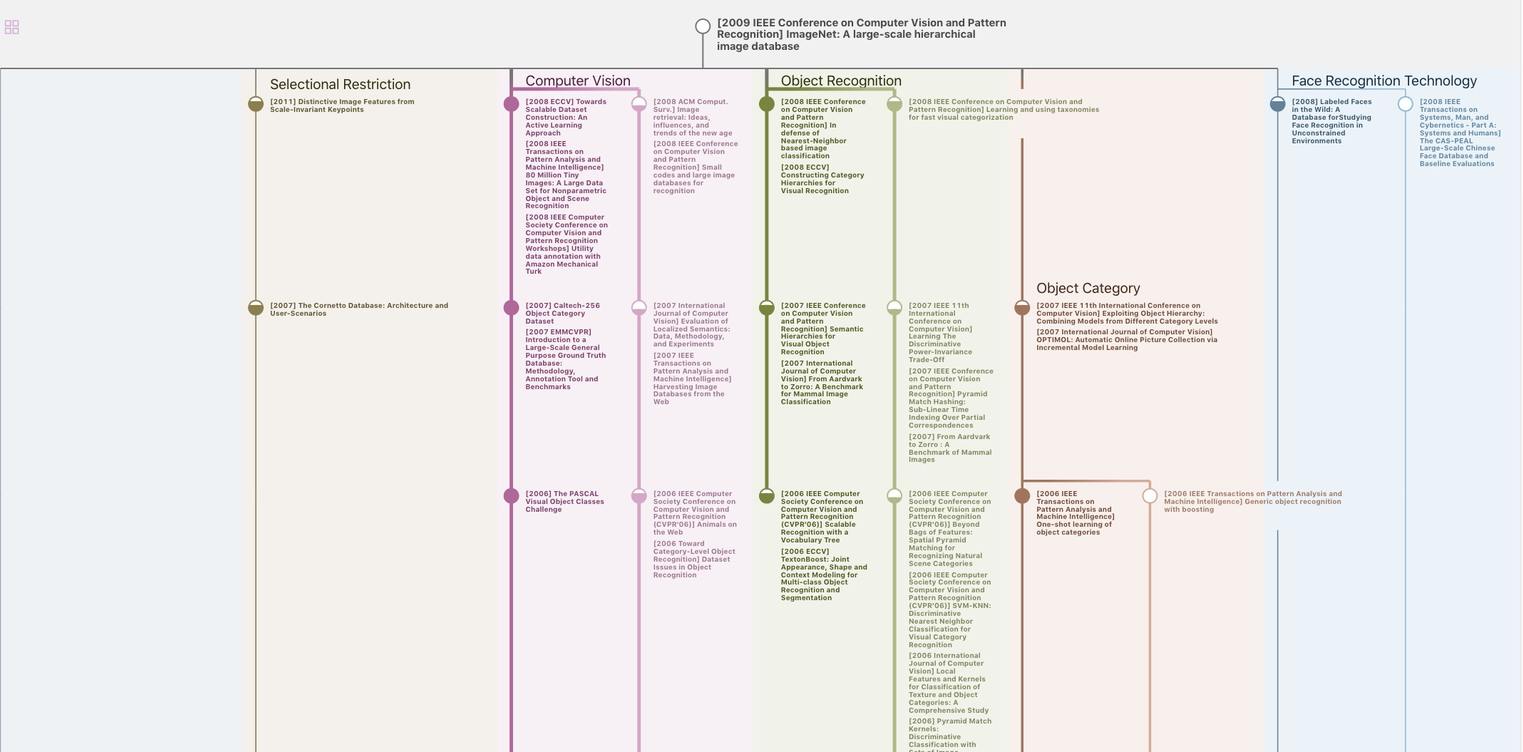
生成溯源树,研究论文发展脉络
Chat Paper
正在生成论文摘要