On the Hartree-Fock Ground State Manifold in Magic Angle Twisted Graphene Systems
arXiv · (2024)
Abstract
Recent experiments have shown that magic angle twisted bilayer graphene
(MATBG) can exhibit correlated insulator behavior at half-filling. Seminal
theoretical results towards understanding this phase in MATBG has shown that
Hartree-Fock ground states (with a positive charge gap) can be exact many-body
ground states of an idealized flat band interacting (FBI) Hamiltonian. We prove
that in the absence of spin and valley degrees of freedom, the only
Hartree-Fock ground states of the FBI Hamiltonian for MATBG are two
ferromagnetic Slater determinants. Incorporating spin and valley degrees of
freedom, we provide a complete characterization of the Hartree-Fock ground
state manifold, which is generated by a U(4) × U(4) hidden
symmetry group acting on five elements. We also introduce new tools for ruling
out translation symmetry breaking in the Hartree-Fock ground state manifold,
which may be of independent interest.
MoreTranslated text
PDF
View via Publisher
AI Read Science
Must-Reading Tree
Example
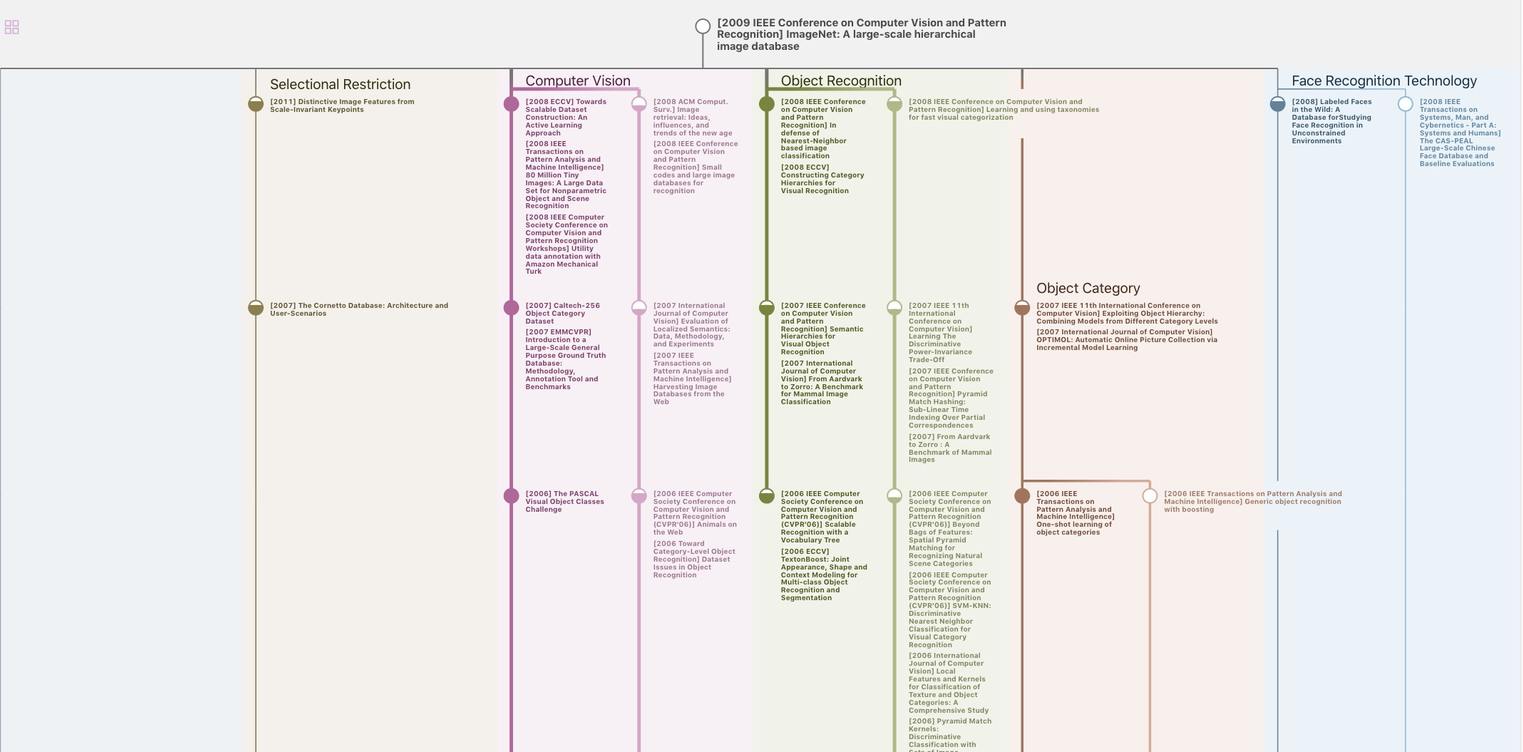
Generate MRT to find the research sequence of this paper
Related Papers
2020
被引用537 | 浏览
2020
被引用116 | 浏览
2021
被引用191 | 浏览
2020
被引用87 | 浏览
Data Disclaimer
The page data are from open Internet sources, cooperative publishers and automatic analysis results through AI technology. We do not make any commitments and guarantees for the validity, accuracy, correctness, reliability, completeness and timeliness of the page data. If you have any questions, please contact us by email: report@aminer.cn
Chat Paper
去 AI 文献库 对话