Precision Prediction for Dengue Fever in Singapore: A Machine Learning Approach Incorporating Meteorological Data.
Tropical medicine and infectious disease(2024)
摘要
OBJECTIVE:This study aimed to improve dengue fever predictions in Singapore using a machine learning model that incorporates meteorological data, addressing the current methodological limitations by examining the intricate relationships between weather changes and dengue transmission.
METHOD:Using weekly dengue case and meteorological data from 2012 to 2022, the data was preprocessed and analyzed using various machine learning algorithms, including General Linear Model (GLM), Support Vector Machine (SVM), Gradient Boosting Machine (GBM), Decision Tree (DT), Random Forest (RF), and eXtreme Gradient Boosting (XGBoost) algorithms. Performance metrics such as Mean Absolute Error (MAE), Root Mean Square Error (RMSE), and R-squared (R2) were employed.
RESULTS:From 2012 to 2022, there was a total of 164,333 cases of dengue fever. Singapore witnessed a fluctuating number of dengue cases, peaking notably in 2020 and revealing a strong seasonality between March and July. An analysis of meteorological data points highlighted connections between certain climate variables and dengue fever outbreaks. The correlation analyses suggested significant associations between dengue cases and specific weather factors such as solar radiation, solar energy, and UV index. For disease predictions, the XGBoost model showed the best performance with an MAE = 89.12, RMSE = 156.07, and R2 = 0.83, identifying time as the primary factor, while 19 key predictors showed non-linear associations with dengue transmission. This underscores the significant role of environmental conditions, including cloud cover and rainfall, in dengue propagation.
CONCLUSION:In the last decade, meteorological factors have significantly influenced dengue transmission in Singapore. This research, using the XGBoost model, highlights the key predictors like time and cloud cover in understanding dengue's complex dynamics. By employing advanced algorithms, our study offers insights into dengue predictive models and the importance of careful model selection. These results can inform public health strategies, aiming to improve dengue control in Singapore and comparable regions.
更多查看译文
关键词
prediction,dengue fever,machine learning,meteorological data
AI 理解论文
溯源树
样例
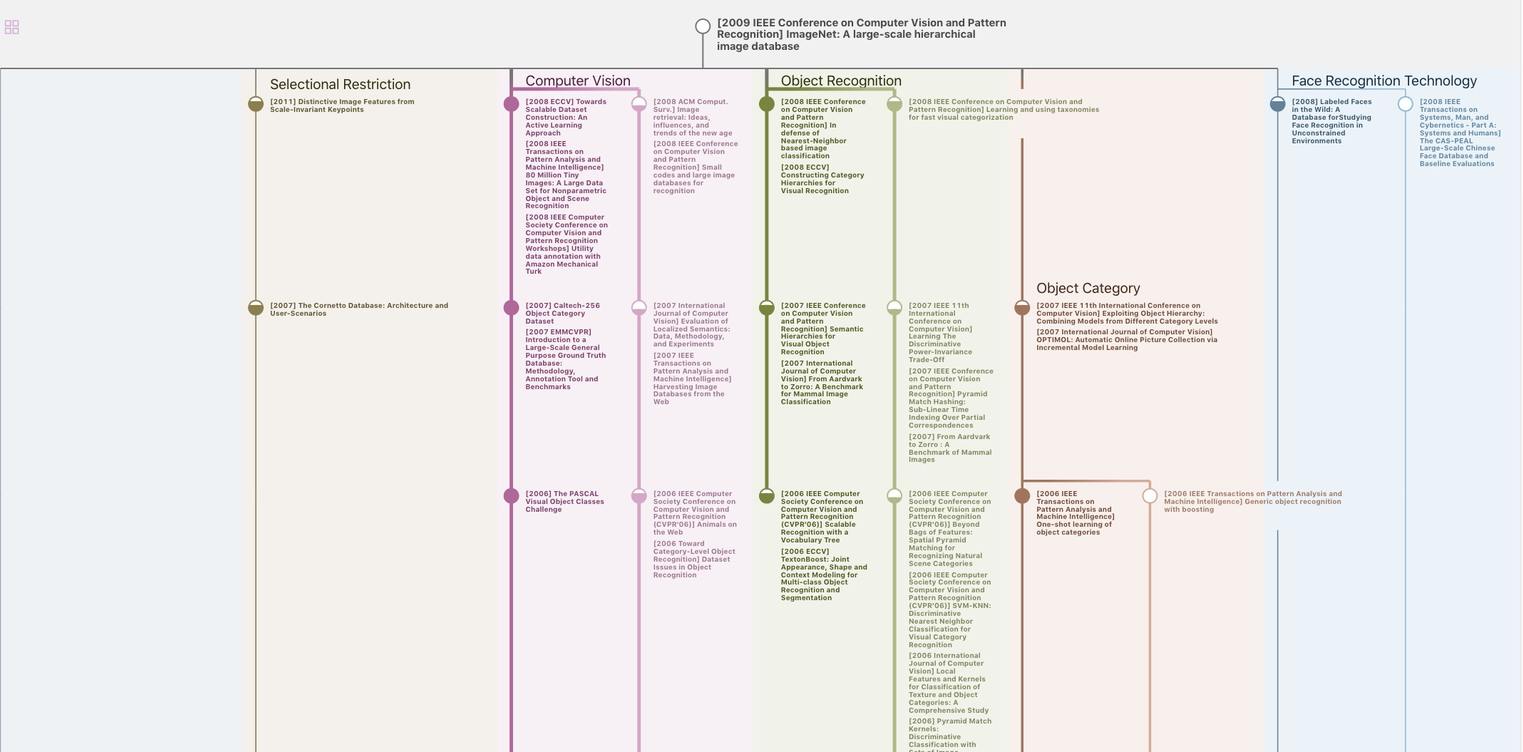
生成溯源树,研究论文发展脉络
Chat Paper
正在生成论文摘要