DFSNet: A 3D Point Cloud Segmentation Network toward Trees Detection in an Orchard Scene
SENSORS(2024)
摘要
In order to guide orchard management robots to realize some tasks in orchard production such as autonomic navigation and precision spraying, this research proposed a deep-learning network called dynamic fusion segmentation network (DFSNet). The network contains a local feature aggregation (LFA) layer and a dynamic fusion segmentation architecture. The LFA layer uses the positional encoders for initial transforming embedding, and progressively aggregates local patterns via the multi-stage hierarchy. The fusion segmentation module (Fus-Seg) can format point tags by learning a multi-embedding space, and the generated tags can further mine the point cloud features. At the experimental stage, significant segmentation results of the DFSNet were demonstrated on the dataset of orchard fields, achieving an accuracy rate of 89.43% and an mIoU rate of 74.05%. DFSNet outperforms other semantic segmentation networks, such as PointNet, PointNet++, D-PointNet++, DGCNN, and Point-NN, with improved accuracies over them by 11.73%, 3.76%, 2.36%, and 2.74%, respectively, and improved mIoUs over the these networks by 28.19%, 9.89%, 6.33%, 9.89, and 24.69%, respectively, on the all-scale dataset (simple-scale dataset + complex-scale dataset). The proposed DFSNet can capture more information from orchard scene point clouds and provide more accurate point cloud segmentation results, which are beneficial to the management of orchards.
更多查看译文
关键词
semantic segmentation,point clouds,deep learning,tree protection robot
AI 理解论文
溯源树
样例
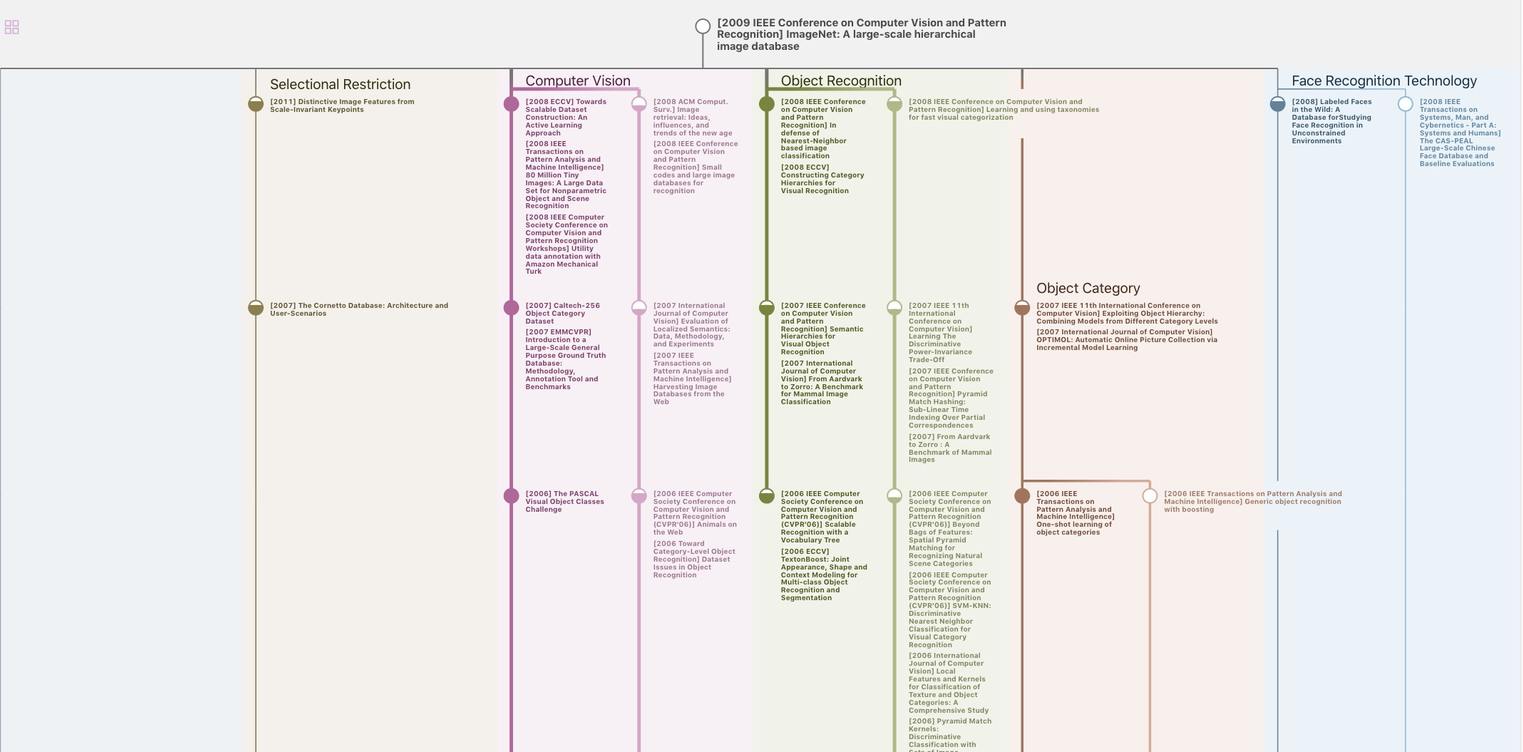
生成溯源树,研究论文发展脉络
Chat Paper
正在生成论文摘要