Machine Learning and Deep Learning Models for Nocturnal High- and Low-Glucose Prediction in Adults with Type 1 Diabetes
DIAGNOSTICS(2024)
摘要
Glucose management at night is a major challenge for people with type 1 diabetes (T1D), especially for those managed with multiple daily injections (MDIs). In this study, we developed machine learning (ML) and deep learning (DL) models to predict nocturnal glucose within the target range (3.9-10 mmol/L), above the target range, and below the target range in subjects with T1D managed with MDIs. The models were trained and tested on continuous glucose monitoring data obtained from 380 subjects with T1D. Two DL algorithms-multi-layer perceptron (MLP) and a convolutional neural network (CNN)-as well as two classic ML algorithms, random forest (RF) and gradient boosting trees (GBTs), were applied. The resulting models based on the DL and ML algorithms demonstrated high and similar accuracy in predicting target glucose (F1 metric: 96-98%) and above-target glucose (F1: 93-97%) within a 30 min prediction horizon. Model performance was poorer when predicting low glucose (F1: 80-86%). MLP provided the highest accuracy in low-glucose prediction. The results indicate that both DL (MLP, CNN) and ML (RF, GBTs) algorithms operating CGM data can be used for the simultaneous prediction of nocturnal glucose values within the target, above-target, and below-target ranges in people with T1D managed with MDIs.
更多查看译文
关键词
type 1 diabetes,continuous glucose monitoring,glucose range,prediction,machine learning,deep learning,neural networks,random forest,boosting trees
AI 理解论文
溯源树
样例
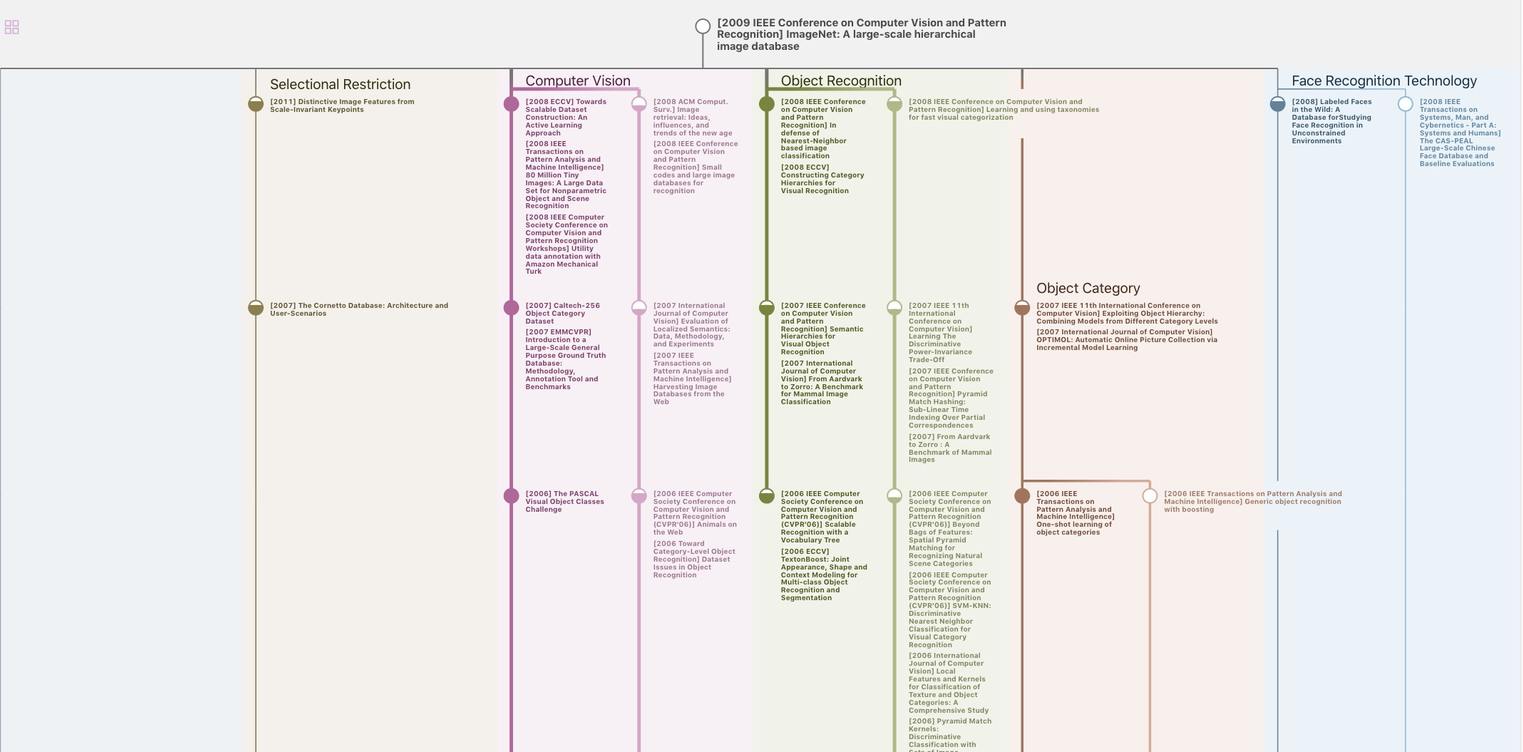
生成溯源树,研究论文发展脉络
Chat Paper
正在生成论文摘要