PyDTS: A Python Toolkit for Deep Learning Time Series Modelling.
Entropy (Basel, Switzerland)(2024)
摘要
In this article, the topic of time series modelling is discussed. It highlights the criticality of analysing and forecasting time series data across various sectors, identifying five primary application areas: denoising, forecasting, nonlinear transient modelling, anomaly detection, and degradation modelling. It further outlines the mathematical frameworks employed in a time series modelling task, categorizing them into statistical, linear algebra, and machine- or deep-learning-based approaches, with each category serving distinct dimensions and complexities of time series problems. Additionally, the article reviews the extensive literature on time series modelling, covering statistical processes, state space representations, and machine and deep learning applications in various fields. The unique contribution of this work lies in its presentation of a Python-based toolkit for time series modelling (PyDTS) that integrates popular methodologies and offers practical examples and benchmarking across diverse datasets.
更多查看译文
关键词
time series modelling,forecasting,nonlinear modelling,denoising,anomaly detection,degradation modelling
AI 理解论文
溯源树
样例
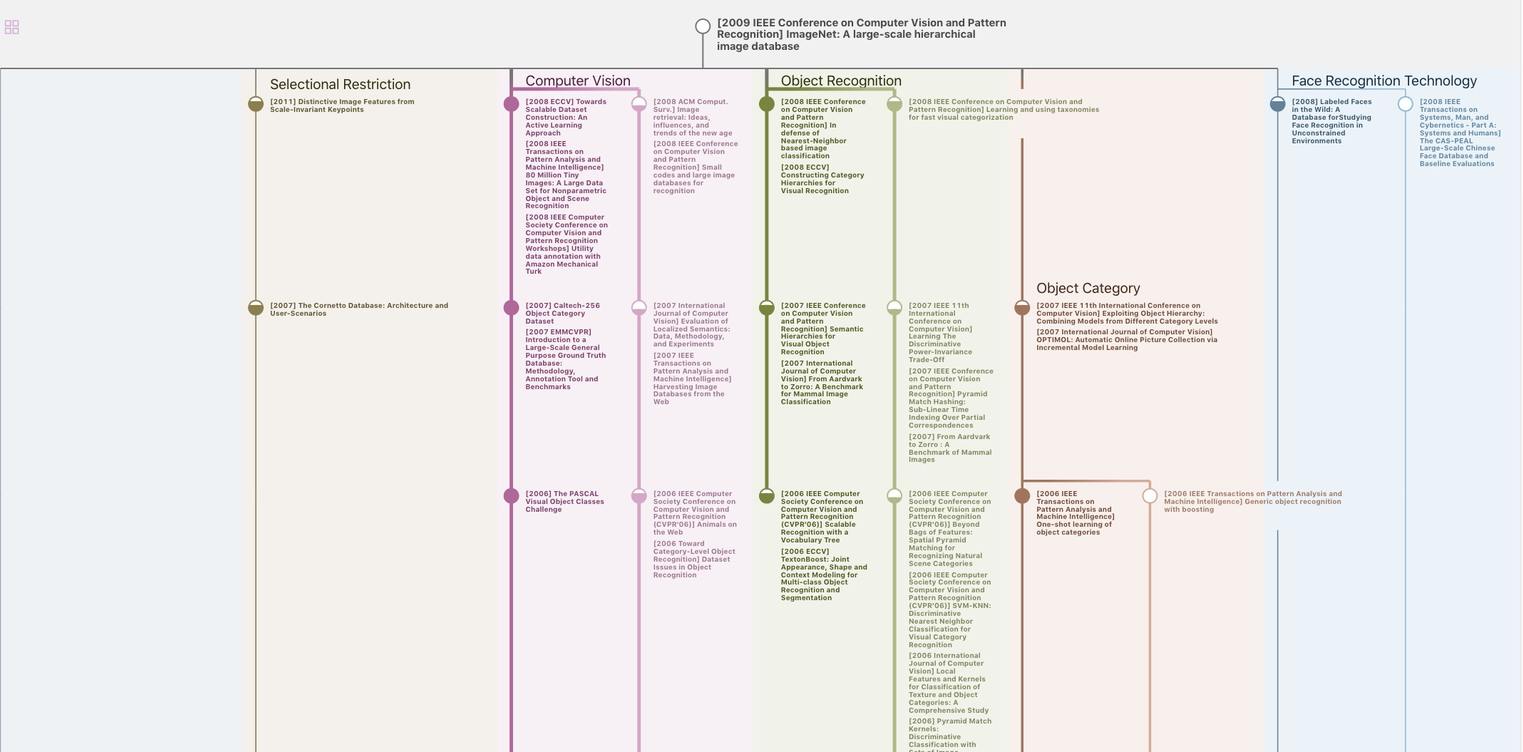
生成溯源树,研究论文发展脉络
Chat Paper
正在生成论文摘要