Multi-performance optimization of low-carbon geopolymer considering mechanical, cost, and CO2 emission based on experiment and interpretable learning
Construction and Building Materials(2024)
摘要
This study proposed a procedure to optimize the mixture proportion of geopolymer using machine learning (ML) and multi-objective optimization (MOO) model, which enhances the compressive strength and minimizes the carbon emission and costs. The experiment was preliminary carried out to analyze the workability, compressive strength, microstructure, and pore characteristics of fly ash/slag-based geopolymer. The results showed that the higher replacement ratio of slag established positive effects on the hardening as well as porosity improvement due to the coexistence of C-S-H and NASH-type binders, especially for a ratio was no more than 60%. Considering the performance of geopolymers is often influenced by multiple factors such as material composition and curing conditions, which could not be solved by traditional methods. A database containing 836 samples was established to analyze the prediction performance of 7 ML models by using Bayesian optimization. The GPR model has good generalization ability, with R2 being 0.99 (0.99) on the testing set (training set). Furthermore, the reliability of the ML models was evaluated by the tests conducted in this paper. The feature importance analysis utilizing SHAP method indicates that Age, HM, and SS are key parameters influencing the compressive strength of geopolymer. The proposed MOO model can effectively evaluate the trade-offs between compressive strength, carbon emission, and costs, and all Pareto solutions for bi-objective and three-objective provided the optimal mixture schemes. On this basis, the design procedure proposed in this paper can give the scheme of geopolymer materials with different requirements (given mixture scheme and given design strength).
更多查看译文
关键词
Low-carbon geopolymer,Experiment,Machine learning,Multi-objective optimization,Design procedure
AI 理解论文
溯源树
样例
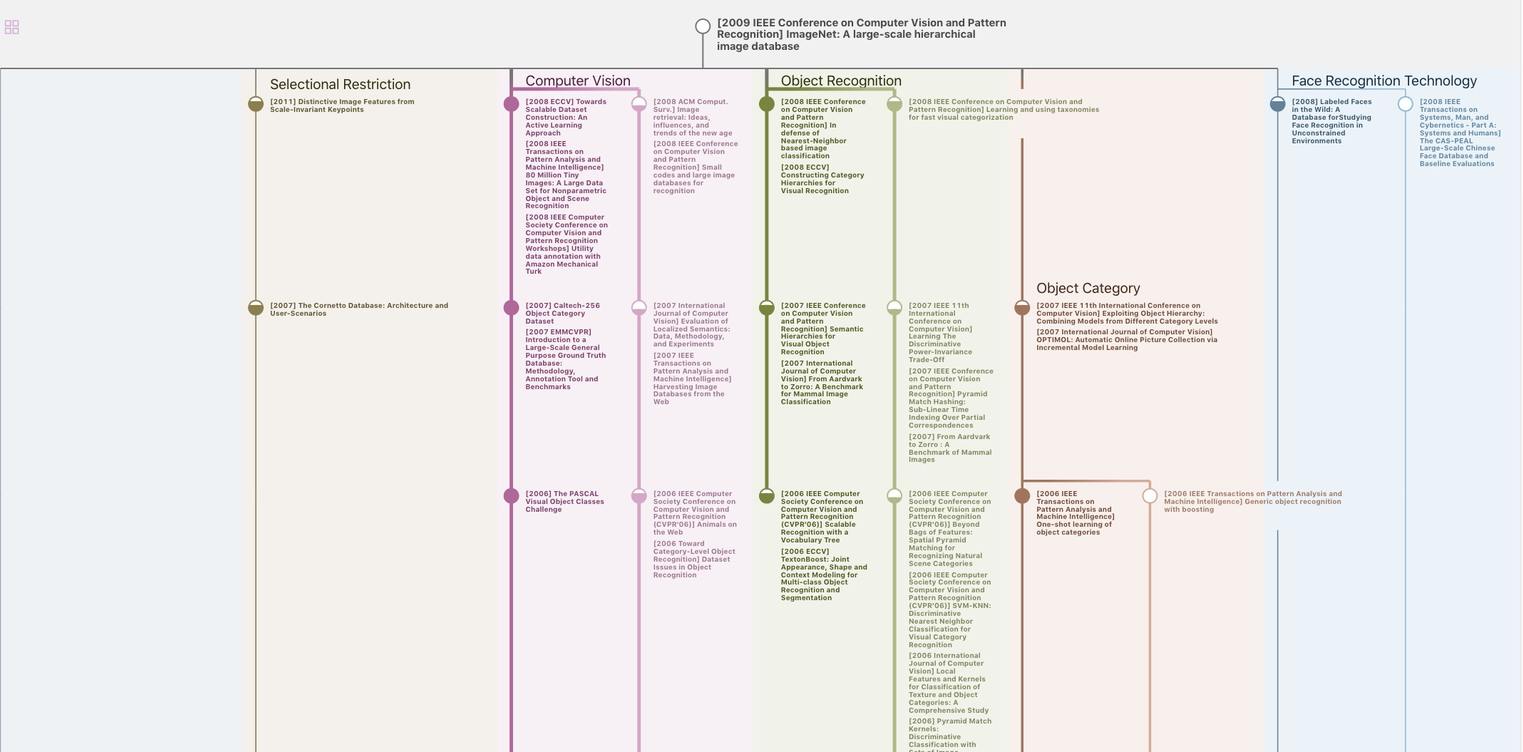
生成溯源树,研究论文发展脉络
Chat Paper
正在生成论文摘要