Harnessing Chemical Space Neural Networks to Systematically Annotate GPCR ligands
biorxiv(2024)
摘要
Drug-target interaction databases comprise millions of manually curated data points, yet there are missed opportunities for repurposing established interaction networks to infer novel drug-target interactions by interpolating on chemical neighborhoods. To address this gap, we collect drug-target interactions on 128 unique GPCRs across 187K molecules and establish an all-vs-all chemical space network, which can be efficiently calculated and parameterised as a graph with 32.4 billion potential edges. Beyond testing state-of-the-art machine learning approaches, we develop a chemical space neural network (CSNN) to infer drug activity classes with up to 98% accuracy, by leveraging the graph of chemical neighbourhoods. We combine this virtual library screen with a fast and cheap experimental platform to validate our predictions and to discover 14 novel drug-GPCR interactions. Altogether, our platform integrates virtual library screening and experimental validation to facilitate fast and efficient coverage of missing drug-target interactions.
### Competing Interest Statement
The authors have declared no competing interest.
更多查看译文
AI 理解论文
溯源树
样例
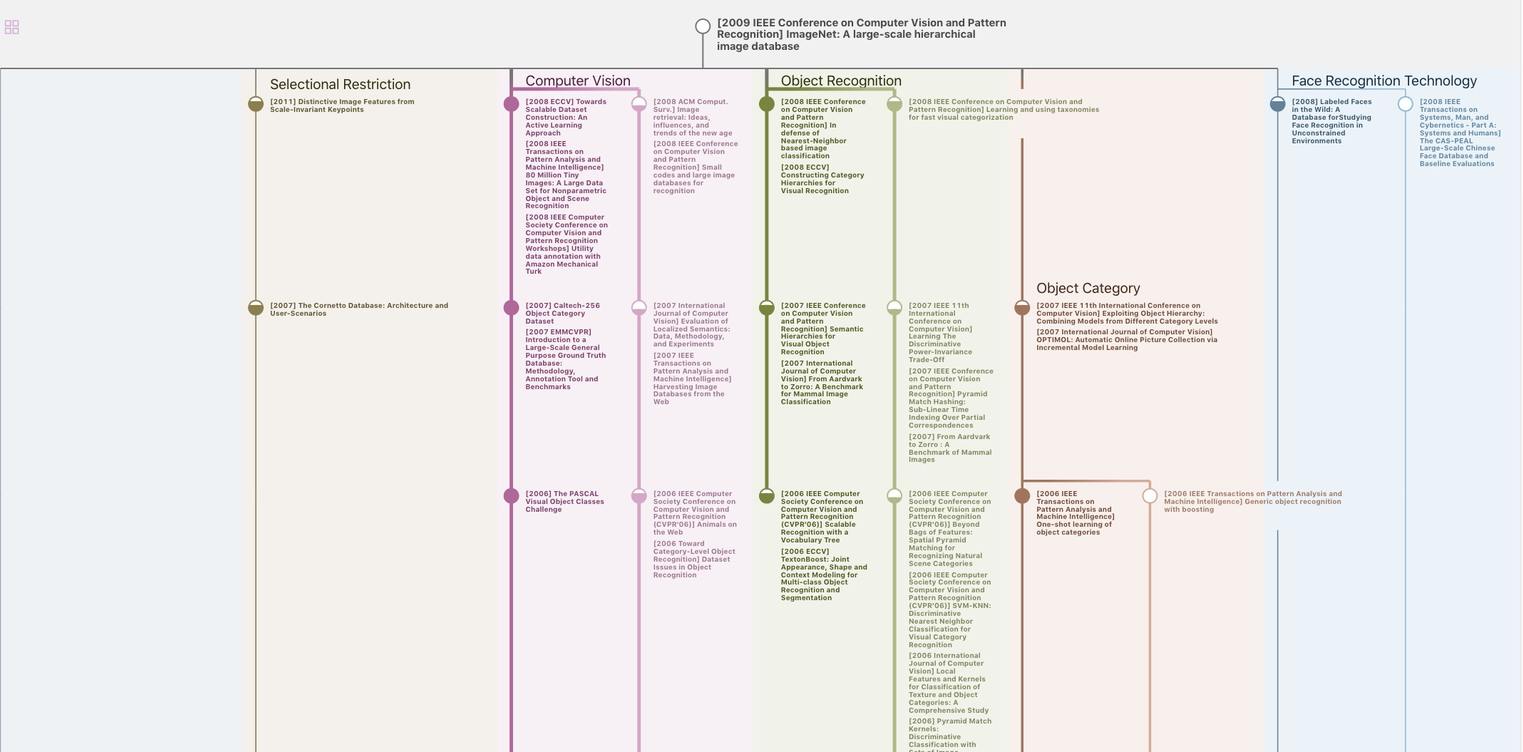
生成溯源树,研究论文发展脉络
Chat Paper
正在生成论文摘要