Beyond Imitation: A Life-long Policy Learning Framework for Path Tracking Control of Autonomous Driving
IEEE Trans Veh Technol(2024)
摘要
Model-free learning-based control methods have recently shown significantadvantages over traditional control methods in avoiding complex vehiclecharacteristic estimation and parameter tuning. As a primary policy learningmethod, imitation learning (IL) is capable of learning control policiesdirectly from expert demonstrations. However, the performance of IL policies ishighly dependent on the data sufficiency and quality of the demonstrations. Toalleviate the above problems of IL-based policies, a lifelong policy learning(LLPL) framework is proposed in this paper, which extends the IL scheme withlifelong learning (LLL). First, a novel IL-based model-free control policylearning method for path tracking is introduced. Even with imperfectdemonstration, the optimal control policy can be learned directly fromhistorical driving data. Second, by using the LLL method, the pre-trained ILpolicy can be safely updated and fine-tuned with incremental executionknowledge. Third, a knowledge evaluation method for policy learning isintroduced to avoid learning redundant or inferior knowledge, thus ensuring theperformance improvement of online policy learning. Experiments are conductedusing a high-fidelity vehicle dynamic model in various scenarios to evaluatethe performance of the proposed method. The results show that the proposed LLPLframework can continuously improve the policy performance with collectedincremental driving data, and achieves the best accuracy and control smoothnesscompared to other baseline methods after evolving on a 7 km curved road.Through learning and evaluation with noisy real-life data collected in anoff-road environment, the proposed LLPL framework also demonstrates itsapplicability in learning and evolving in real-life scenarios.
更多查看译文
关键词
Task analysis,Vehicle dynamics,Data models,Optimal control,Adaptation models,Costs,Computational modeling,Autonomous driving,life-long learning,learning from demonstration,model-free control,path tracking
AI 理解论文
溯源树
样例
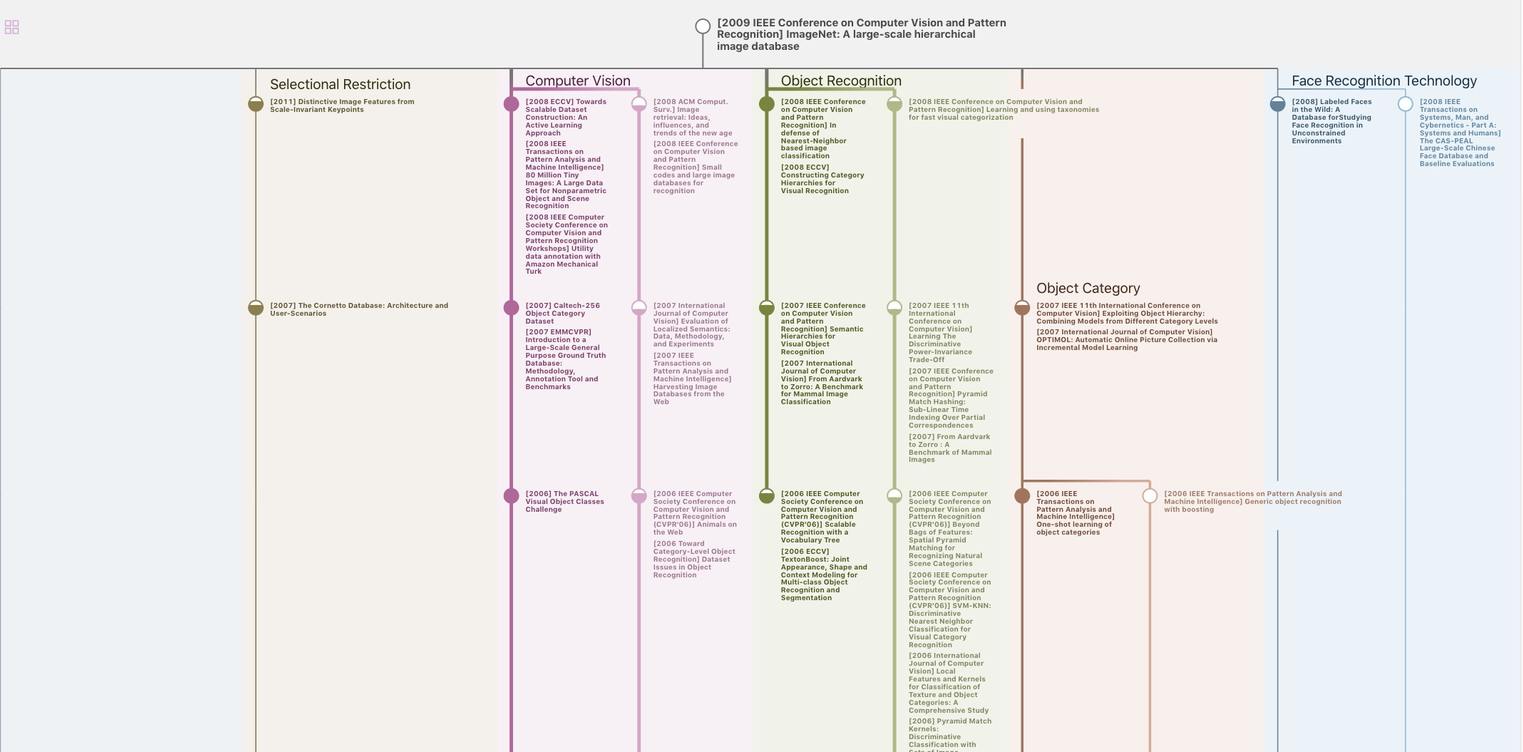
生成溯源树,研究论文发展脉络
Chat Paper
正在生成论文摘要