Policy Optimization finds Nash Equilibrium in Regularized General-Sum LQ Games
arxiv(2024)
摘要
In this paper, we investigate the impact of introducing relative entropy
regularization on the Nash Equilibria (NE) of General-Sum N-agent games,
revealing the fact that the NE of such games conform to linear Gaussian
policies. Moreover, it delineates sufficient conditions, contingent upon the
adequacy of entropy regularization, for the uniqueness of the NE within the
game. As Policy Optimization serves as a foundational approach for
Reinforcement Learning (RL) techniques aimed at finding the NE, in this work we
prove the linear convergence of a policy optimization algorithm which (subject
to the adequacy of entropy regularization) is capable of provably attaining the
NE. Furthermore, in scenarios where the entropy regularization proves
insufficient, we present a δ-augmentation technique, which facilitates
the achievement of an ϵ-NE within the game.
更多查看译文
AI 理解论文
溯源树
样例
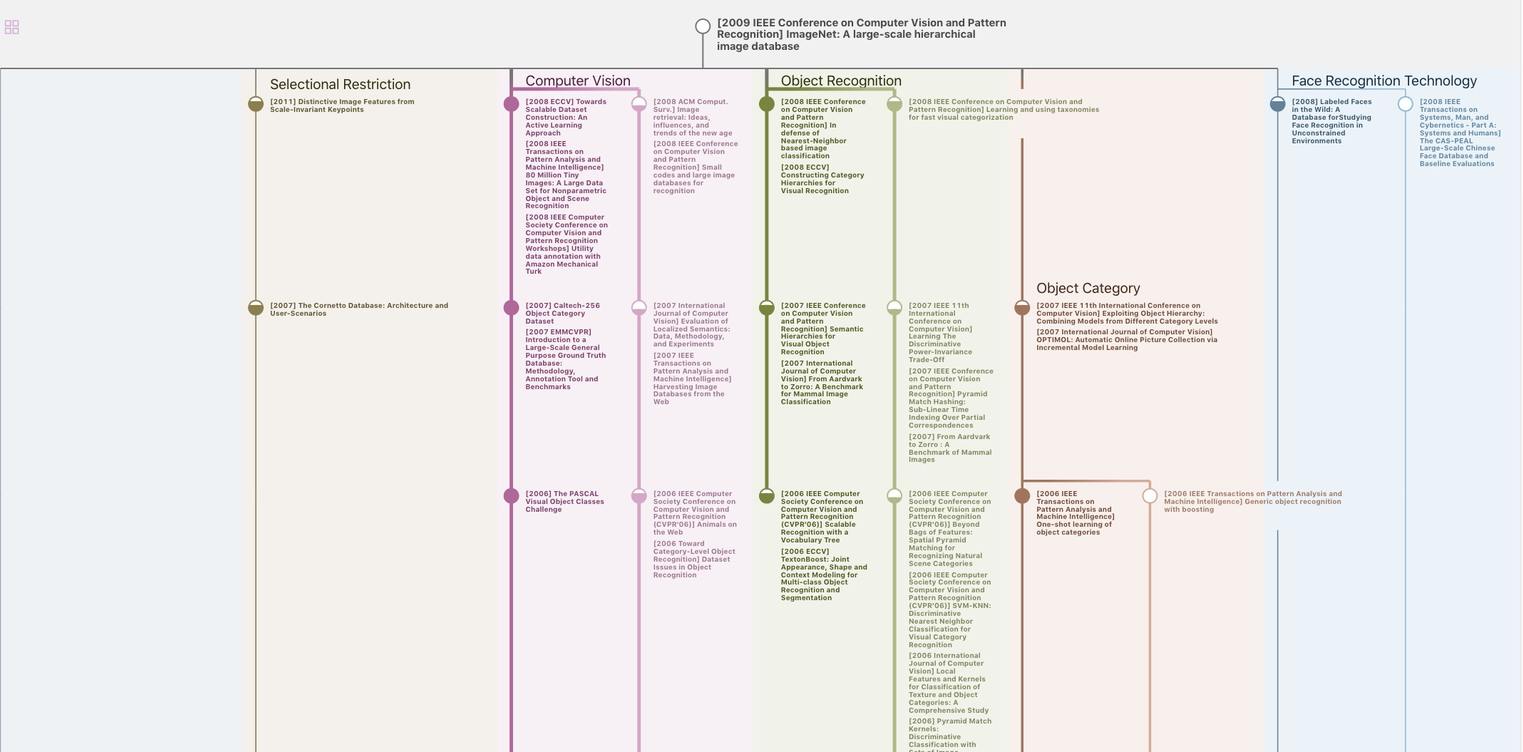
生成溯源树,研究论文发展脉络
Chat Paper
正在生成论文摘要