Machine Learning Based Source Number Estimation under Unknown Spatially Correlated Noise
IEEE Sensors Journal(2024)
摘要
The existing model-driven methods for source number estimation (SNE) under spatially correlated noise are limited by the inherent shortcomings of model assumptions and subjective parameter settings, and have high requirements for signal-to-noise ratio and sample size. Although machine learning (ML) has begun to emerge in SNE due to its powerful learning ability, existing ML-based methods mainly focus on Gaussian white noise, and there are few works on spatially correlated noise. In view of this, an ML-based SNE scheme for spatially correlated noise is designed including feature extraction, data preprocessing, and neural network design and training. Specifically, we first propose a new feature vector F
WGDE-1
based on weighted Gerschgorin disk estimator (WGDE), and utilize different preprocessing methods to preprocess it before inputting it into the designed fully-connected neural network. Due to the inherent data-driven advantage of ML and the effectiveness of the extracted feature vector combined with logarithm preprocessing, the proposed method can perform well. Moreover, considering the complexity and uncertainty of the actual propagation environment, the model-based noise modeling method will deteriorate the estimation performance due to model mismatch, online learning is introduced to further improve the robustness of the proposed method, which can cope with the impact of the change of noise conditions to a certain extent. Simulation results verify the effectiveness of the proposed method in dealing with the unknown spatially correlated noise.
更多查看译文
关键词
Source number estimation,spatially correlated noise,feature extraction,machine learning,online learning
AI 理解论文
溯源树
样例
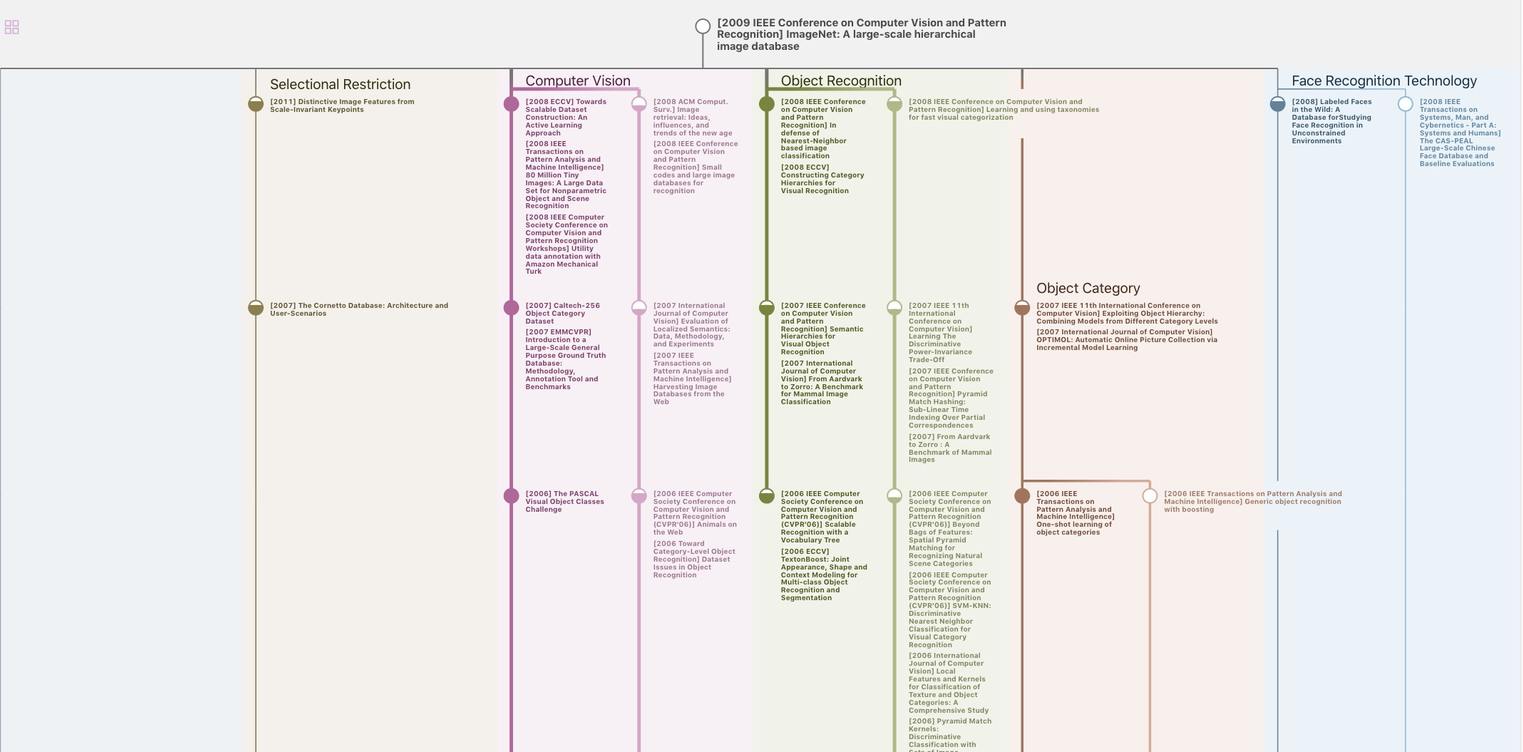
生成溯源树,研究论文发展脉络
Chat Paper
正在生成论文摘要