DRA: A Data Reconstruction Attack on Vertical Federated K-Means Clustering
EXPERT SYSTEMS WITH APPLICATIONS(2024)
Abstract
Vertical federated learning has become a novel approach to address the challenges of sharing training data and preserving data privacy in machine learning. However, there still exist privacy leakage risks in parameter transmission process while directly running vertical federated k-means clustering. To address these issues, this paper proposes a data reconstruction attack based on the distance matrices. The attack model does not affect the convergence speed and results of the model, nor does it disrupt the training process. The attacker only needs to receive, record, and analyze the transmitted distance matrices in the training process, and through steps, as establishment of new coordinate, possible location inference, target location extraction, coordinate alignment, and attribute filtering, can reconstruct the original training data set. Extensive experiments were conducted on three public datasets, the average relative Euclidean distances between the reconstructed dataset samples and the training dataset samples are 0.489%, 0.610%, and 0.887% for small-sample datasets, datasets with diverse data distributions, and high-dimensional datasets, respectively. Showing that the attack model is effective in the VFk-means clustering algorithm.
MoreTranslated text
Key words
Vertical federated learning,Federated k-means clustering,Attack model
AI Read Science
Must-Reading Tree
Example
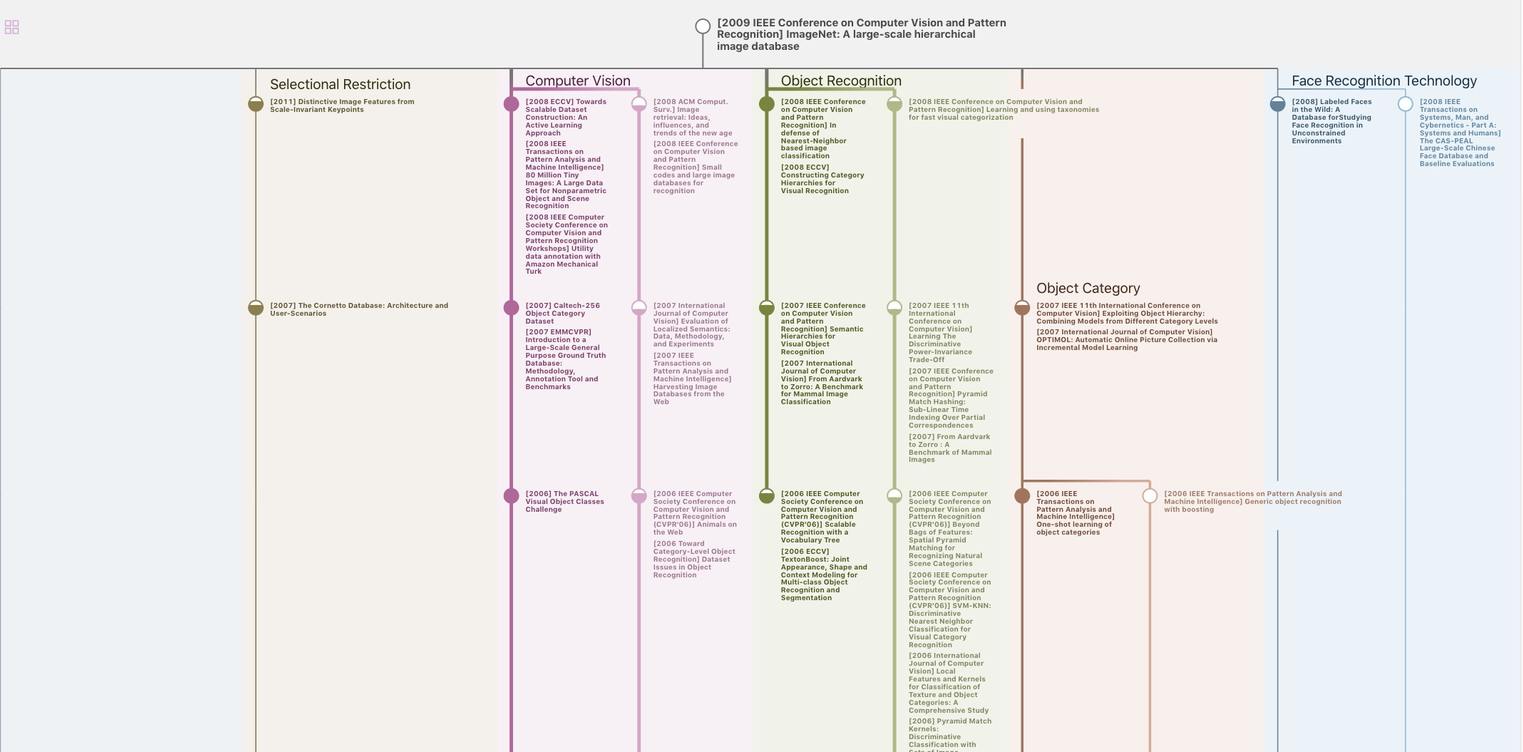
Generate MRT to find the research sequence of this paper
Chat Paper
Summary is being generated by the instructions you defined