An Active Perception Game for Robust Autonomous Exploration
CoRR(2024)
Abstract
We formulate active perception for an autonomous agent that explores an
unknown environment as a two-player zero-sum game: the agent aims to maximize
information gained from the environment while the environment aims to minimize
the information gained by the agent. In each episode, the environment reveals a
set of actions with their potentially erroneous information gain. In order to
select the best action, the robot needs to recover the true information gain
from the erroneous one. The robot does so by minimizing the discrepancy between
its estimate of information gain and the true information gain it observes
after taking the action. We propose an online convex optimization algorithm
that achieves sub-linear expected regret O(T^3/4) for estimating the
information gain. We also provide a bound on the regret of active perception
performed by any (near-)optimal prediction and trajectory selection algorithms.
We evaluate this approach using semantic neural radiance fields (NeRFs) in
simulated realistic 3D environments to show that the robot can discover up to
12
M3ED dataset, the proposed algorithm reduced the error of information gain
prediction in occupancy map by over 67
occupancy maps on a Jackal ground robot, we show that this approach can
calculate complicated trajectories that efficiently explore all occluded
regions.
MoreTranslated text
AI Read Science
Must-Reading Tree
Example
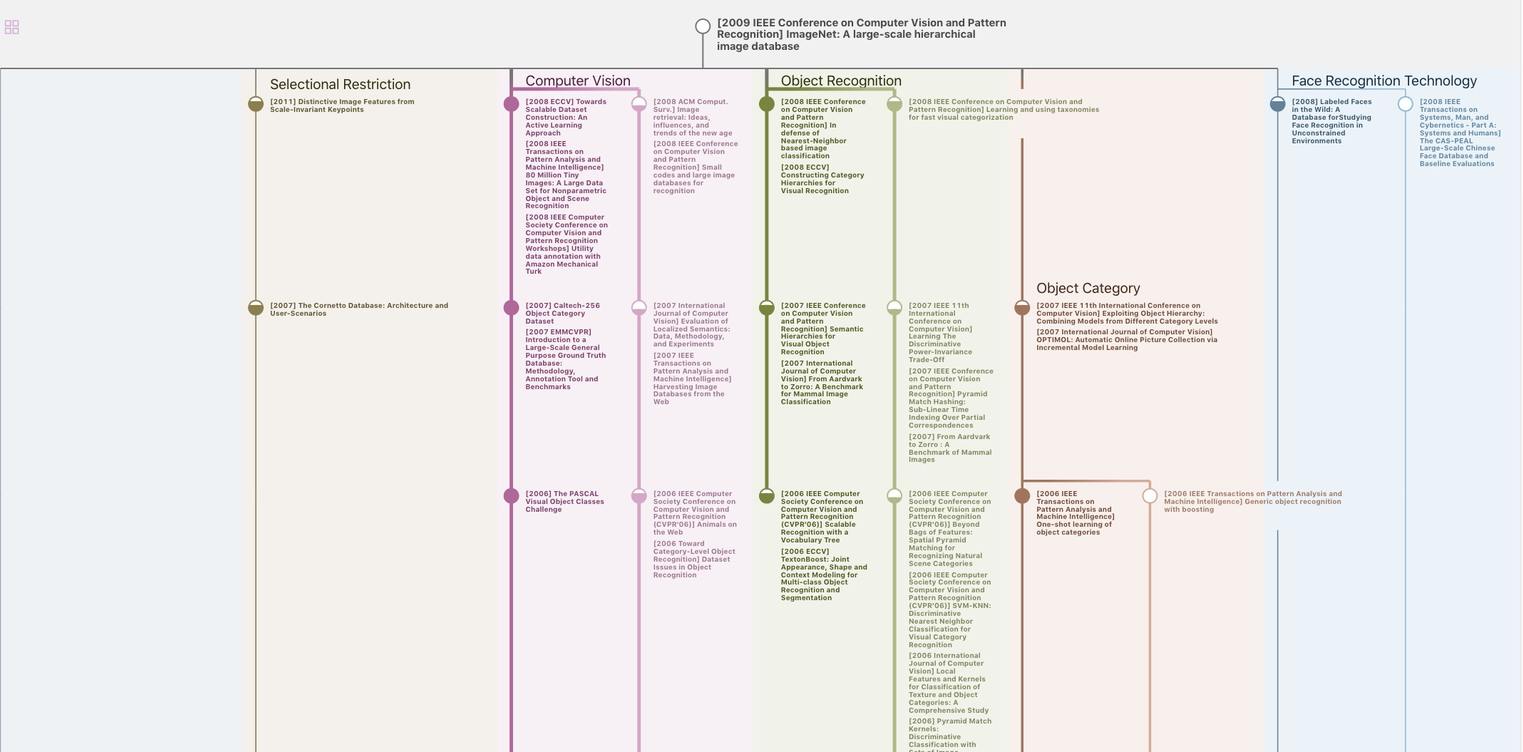
Generate MRT to find the research sequence of this paper
Chat Paper
Summary is being generated by the instructions you defined