S2RC-GCN: A Spatial-Spectral Reliable Contrastive Graph Convolutional Network for Complex Land Cover Classification Using Hyperspectral Images
2024 International Joint Conference on Neural Networks (IJCNN)(2024)
摘要
Spatial correlations between different ground objects are an importantfeature of mining land cover research. Graph Convolutional Networks (GCNs) caneffectively capture such spatial feature representations and have demonstratedpromising results in performing hyperspectral imagery (HSI) classificationtasks of complex land. However, the existing GCN-based HSI classificationmethods are prone to interference from redundant information when extractingcomplex features. To classify complex scenes more effectively, this studyproposes a novel spatial-spectral reliable contrastive graph convolutionalclassification framework named S2RC-GCN. Specifically, we fused the spectraland spatial features extracted by the 1D- and 2D-encoder, and the 2D-encoderincludes an attention model to automatically extract important information. Wethen leveraged the fused high-level features to construct graphs and fed theresulting graphs into the GCNs to determine more effective graphrepresentations. Furthermore, a novel reliable contrastive graph convolutionwas proposed for reliable contrastive learning to learn and fuse robustfeatures. Finally, to test the performance of the model on complex objectclassification, we used imagery taken by Gaofen-5 in the Jiang Xia area toconstruct complex land cover datasets. The test results show that compared withother models, our model achieved the best results and effectively improved theclassification performance of complex remote sensing imagery.
更多查看译文
AI 理解论文
溯源树
样例
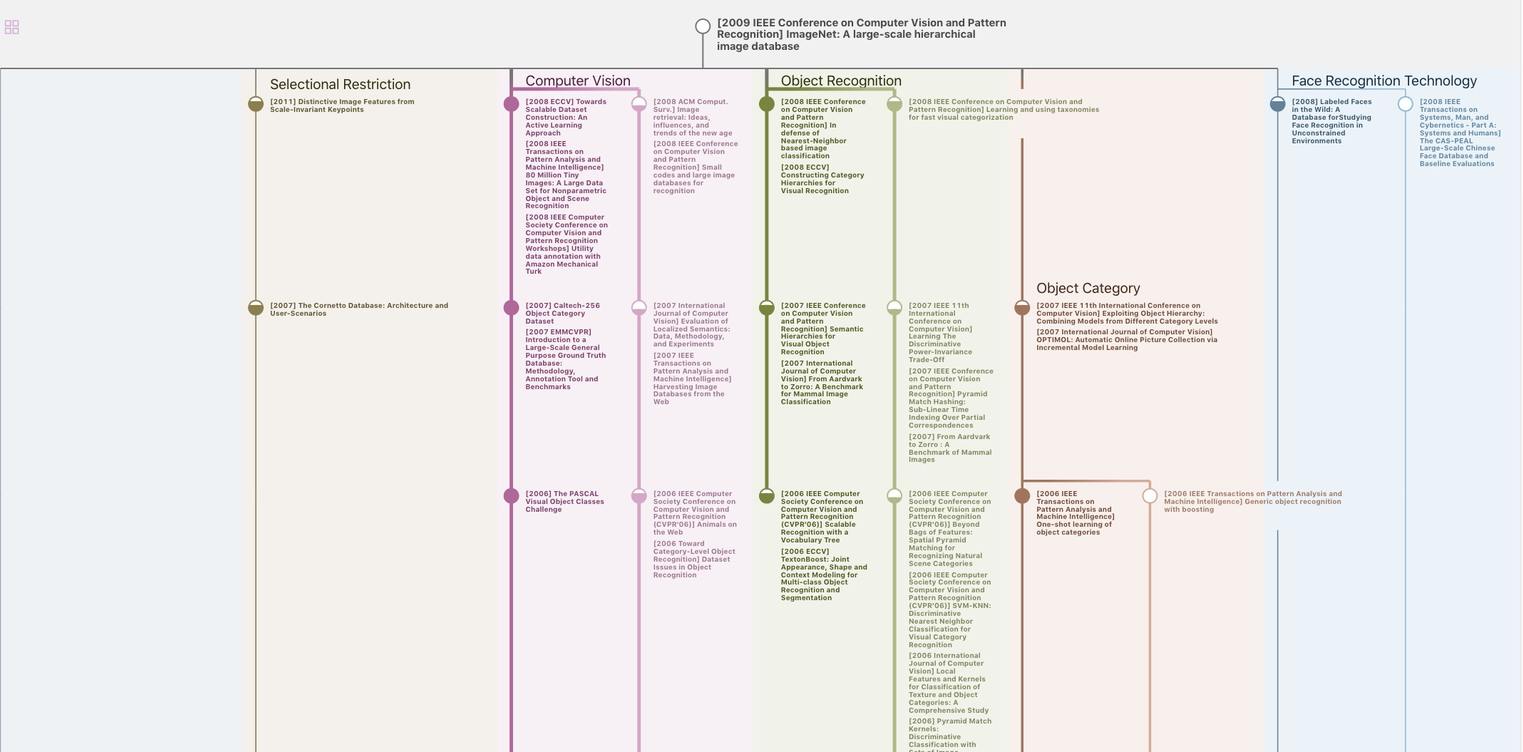
生成溯源树,研究论文发展脉络
Chat Paper
正在生成论文摘要