HairFastGAN: Realistic and Robust Hair Transfer with a Fast Encoder-Based Approach
arXiv (Cornell University)(2024)
摘要
Our paper addresses the complex task of transferring a hairstyle from areference image to an input photo for virtual hair try-on. This task ischallenging due to the need to adapt to various photo poses, the sensitivity ofhairstyles, and the lack of objective metrics. The current state of the arthairstyle transfer methods use an optimization process for different parts ofthe approach, making them inexcusably slow. At the same time, fasterencoder-based models are of very low quality because they either operate inStyleGAN's W+ space or use other low-dimensional image generators.Additionally, both approaches have a problem with hairstyle transfer when thesource pose is very different from the target pose, because they either don'tconsider the pose at all or deal with it inefficiently. In our paper, wepresent the HairFast model, which uniquely solves these problems and achieveshigh resolution, near real-time performance, and superior reconstructioncompared to optimization problem-based methods. Our solution includes a newarchitecture operating in the FS latent space of StyleGAN, an enhancedinpainting approach, and improved encoders for better alignment, colortransfer, and a new encoder for post-processing. The effectiveness of ourapproach is demonstrated on realism metrics after random hairstyle transfer andreconstruction when the original hairstyle is transferred. In the mostdifficult scenario of transferring both shape and color of a hairstyle fromdifferent images, our method performs in less than a second on the Nvidia V100.Our code is available at https://github.com/AIRI-Institute/HairFastGAN.
更多查看译文
AI 理解论文
溯源树
样例
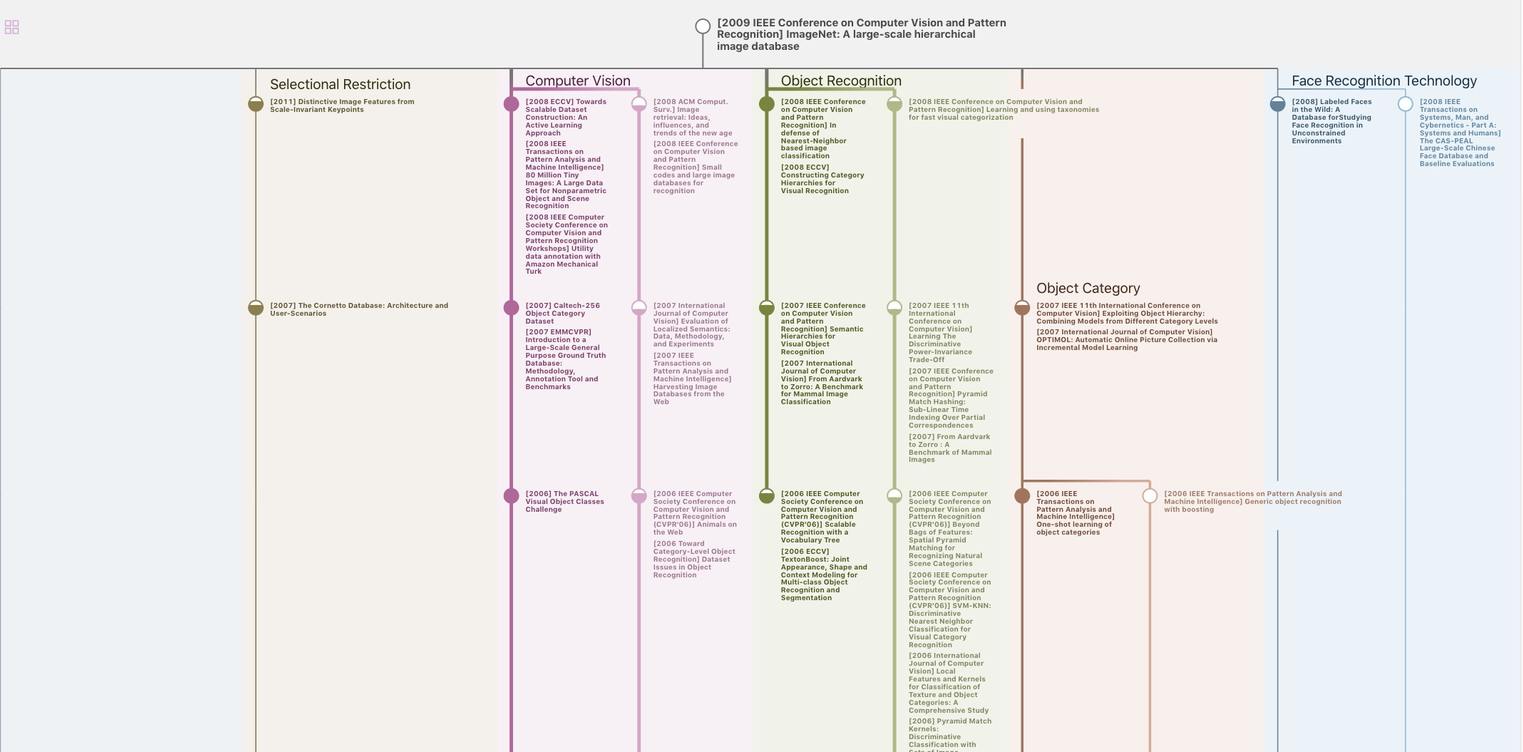
生成溯源树,研究论文发展脉络
Chat Paper
正在生成论文摘要