Gradient Methods for Scalable Multi-value Electricity Network Expansion Planning
arxiv(2024)
摘要
We consider multi-value expansion planning (MEP), a general bilevel
optimization model in which a planner optimizes arbitrary functions of the
dispatch outcome in the presence of a partially controllable, competitive
electricity market. The MEP problem can be used to jointly plan various grid
assets, such as transmission, generation, and battery storage capacities;
examples include identifying grid investments that minimize emissions in the
absence of a carbon tax, maximizing the profit of a portfolio of renewable
investments and long-term energy contracts, or reducing price inequities
between different grid stakeholders. The MEP problem, however, is in general
nonconvex, making it difficult to solve exactly for large real-world systems.
Therefore, we propose a fast stochastic implicit gradient-based heuristic
method that scales well to large networks with many scenarios. We use a strong
duality reformulation and the McCormick envelope to provide a lower bound on
the performance of our algorithm via convex relaxation. We test the performance
of our method on a large model of the U.S. Western Interconnect and demonstrate
that it scales linearly with network size and number of scenarios and can be
efficiently parallelized on large machines. We find that for medium-sized 16
hour cases, gradient descent on average finds a 5.3x lower objective value in
16.5x less time compared to a traditional reformulation-based approach solved
with an interior point method. We conclude with a large example in which we
jointly plan transmission, generation, and storage for a 768 hour case on 100
node system, showing that emissions penalization leads to additional 40.0%
reduction in carbon intensity at an additional cost of $17.1/MWh.
更多查看译文
AI 理解论文
溯源树
样例
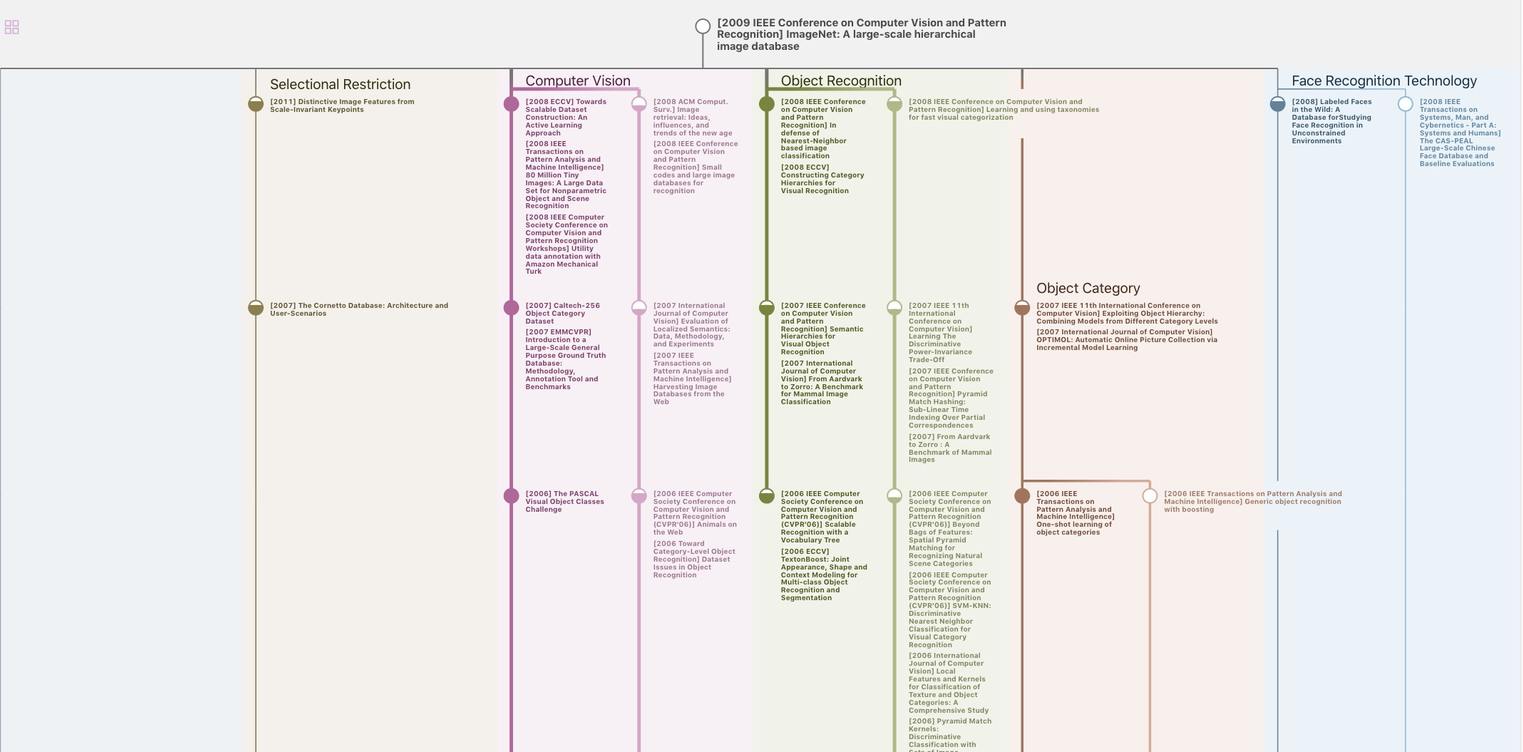
生成溯源树,研究论文发展脉络
Chat Paper
正在生成论文摘要