Constrained Independent Vector Analysis with References: Algorithms and Performance Evaluation.
Asilomar Conference on Signals, Systems and Computers(2023)
摘要
Independent vector analysis (IVA) is an effective approach to joint blind source separation that fully leverages the statistical dependence across multiple datasets. Nonetheless, its performance might suffer when the number of datasets increases. Constrained IVA is an effective way to bypass computational issues and improve the separation quality by incorporating prior information. This paper introduces a class of approaches to constrained IVA. First, besides the existing augmented Lagrange method, we introduce two novel approaches: the alternating direction method of multipliers and multi-objective optimization. Second, by exploiting the non-orthogonal decoupIing of the IVA cost, we derive gradient descent and Newton's method to minimize the objective function. Finally, we demonstrate the effectiveness of algorithms for constrained IVA over unconstrained IVA with simulations.
更多查看译文
关键词
joint blind source separation,independent vector analysis,constrained optimization
AI 理解论文
溯源树
样例
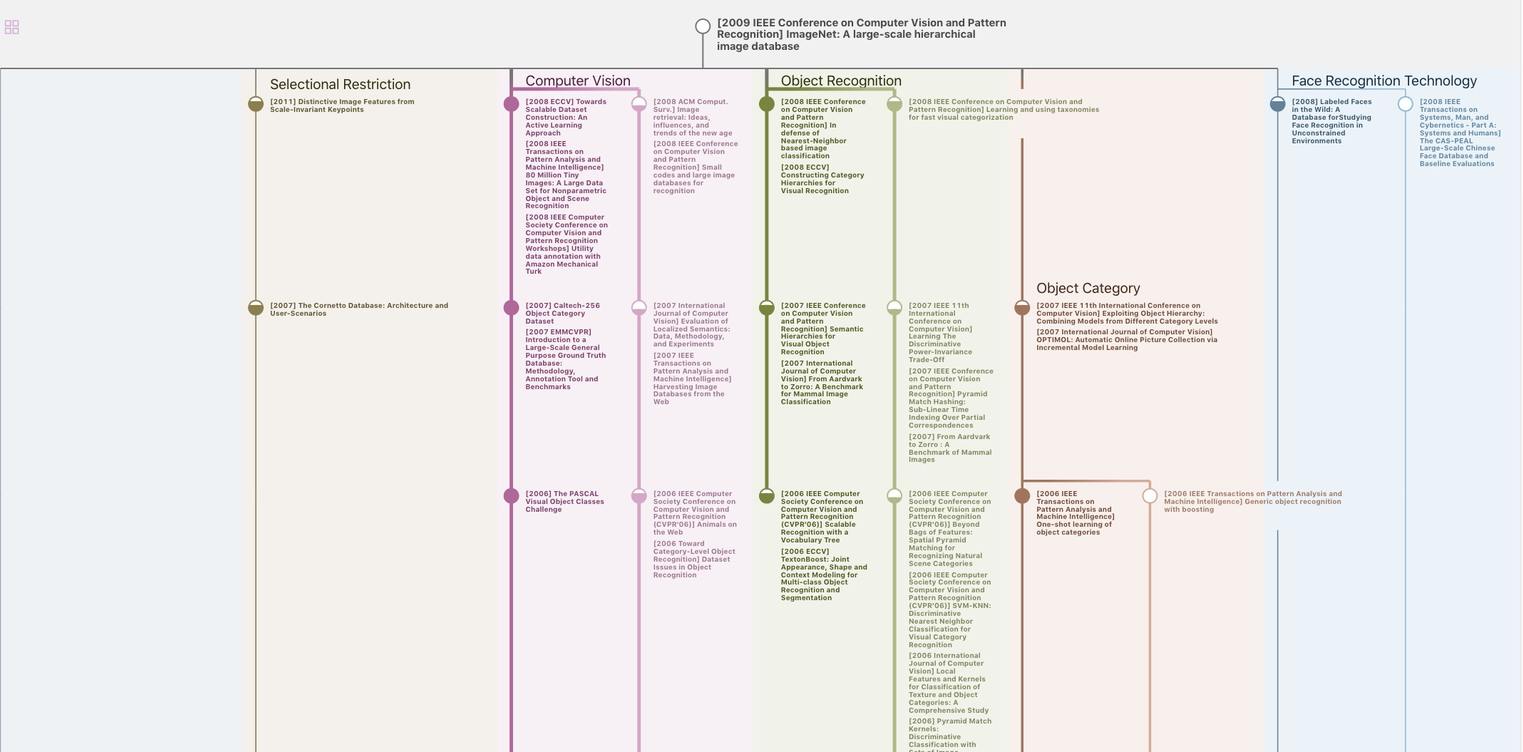
生成溯源树,研究论文发展脉络
Chat Paper
正在生成论文摘要