On the size generalizibility of graph neural networks for learning resource allocation
Science China Information Sciences(2024)
摘要
Size generalization is important for learning resource allocation policies in wireless systems with time-varying scales. If a neural network for learning a wireless policy is not generalizable to the size of its input, it has to be re-trained whenever the system scale changes, which hinders its practical use due to the unaffordable training costs. Graph neural networks (GNNs) have been shown with size generalization ability empirically when optimizing resource allocation. Yet, are GNNs naturally size generalizable? In this paper, we argue that GNNs are not always size generalizable for resource allocation. We find that the aggregation and activation functions of the GNNs for learning a class of wireless policies play a key role in their size generalization ability. We take the GNN with the mean aggregator, called mean-GNN, as an example to reveal a size generalization condition. To demonstrate how to satisfy the condition, we learn power and bandwidth allocation policies for ultra-reliable low-latency communications and show that selecting or pre-training the activation function in the output layer of mean-GNN can make the GNN size generalizable. Simulation results validate our analysis and evaluate the performance of the learned policies.
更多查看译文
关键词
size generalization,graph neural networks,resource allocation,permutation equivariance
AI 理解论文
溯源树
样例
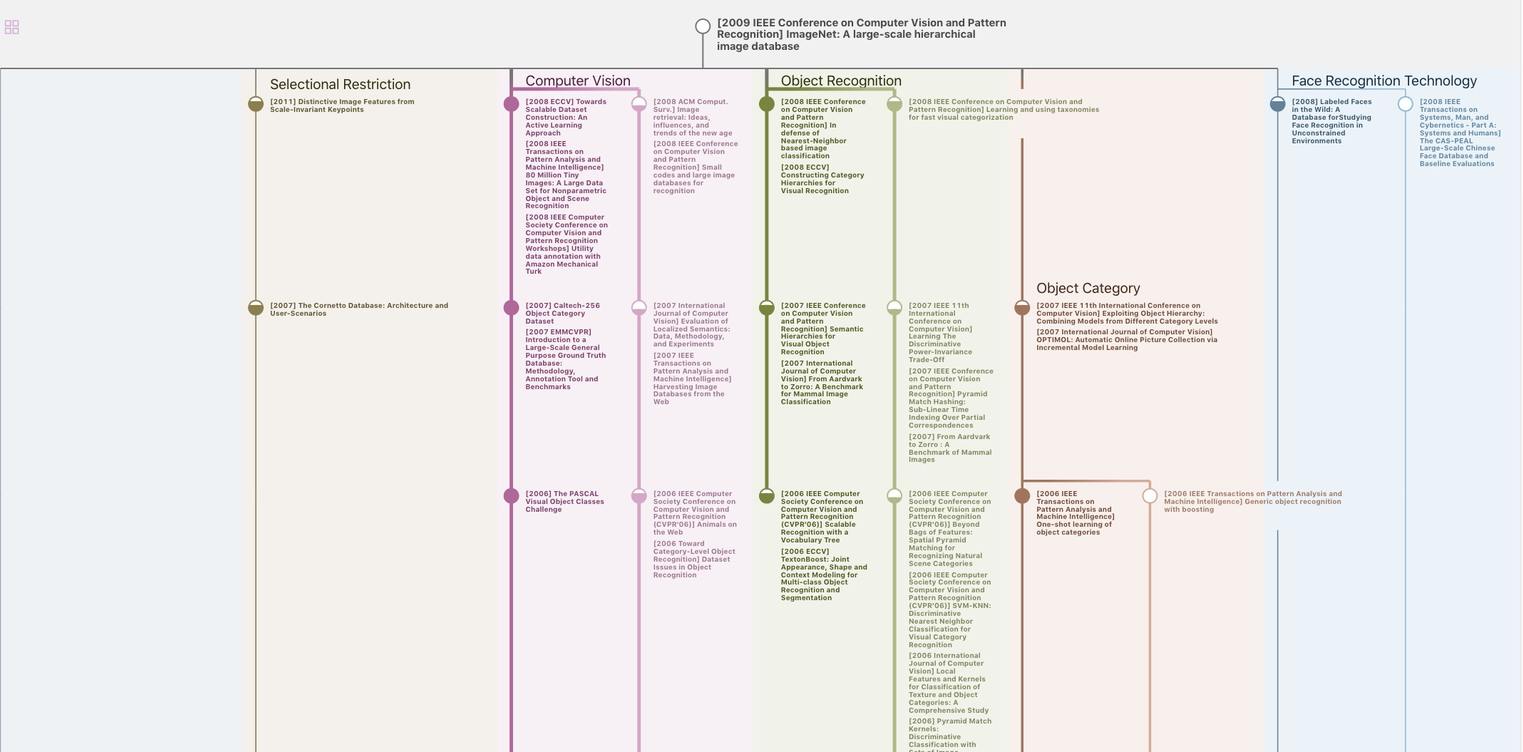
生成溯源树,研究论文发展脉络
Chat Paper
正在生成论文摘要