DMM: A Deep Reinforcement Learning based Map Matching Framework for Cellular Data
IEEE Transactions on Knowledge and Data Engineering(2024)
摘要
This paper presents a novel map matching framework that adopts deep learning techniques to map a sequence of cell tower locations to a trajectory on a road network. Map matching is an essential pre-processing step for many applications, such as traffic optimization and human mobility analysis. However, most recent approaches are based on hidden Markov models (HMMs) or neural networks that are hard to consider high-order location information or heuristics observed from real driving scenarios. In this paper, we develop a deep reinforcement learning based map matching framework for cellular data, named as DMM, which adopts a recurrent neural network (RNN) coupled with a reinforcement learning scheme to identify the most-likely trajectory of roads given a sequence of cell towers. To transform DMM into a practical system, several challenges are addressed by developing a set of techniques, including spatial-aware representation of input cell tower sequences, an encoder-decoder based RNN network for map matching model with variable-length input and output, and a global heuristics-driven reinforcement learning based scheme for optimizing the parameters of the encoder-decoder map matching model. Extensive experiments on a large-scale anonymized cellular dataset reveal that DMM provides high map matching accuracy and fast inference time.
更多查看译文
关键词
Map matching,Deep reinforcement learning,Location-based services
AI 理解论文
溯源树
样例
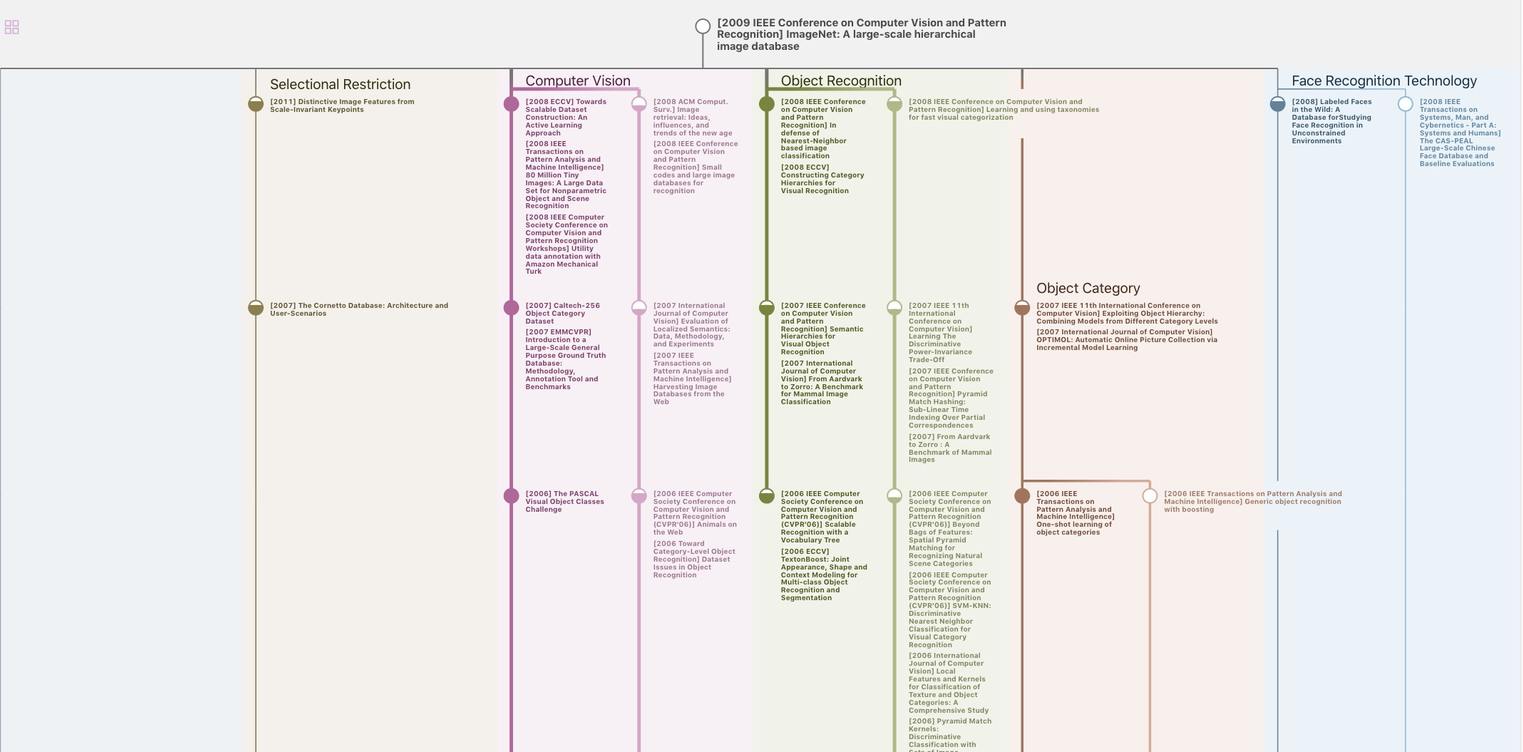
生成溯源树,研究论文发展脉络
Chat Paper
正在生成论文摘要