Federated Learning for Massive MIMO Power Allocation.
Asilomar Conference on Signals, Systems and Computers(2023)
摘要
This work proposes the use of federated learning (FL) for deep learning-based power allocation in massive MIMO systems. The conventional approach for learning-based power allocation builds on centralized training, where training data is collected at a single node which performs training. On the other hand, doing so requires extensive data exchange and processing at the central node, leading to high communication and computational overhead. In contrast, FL enables distributed training without the need to centralize training data, allowing for parallelization of the training load (hence speeding up training), as well as efficient use of the available communication resources. In this work we study FL for massive MIMO power allocation with heterogeneity-aware user sampling, and show that FL-based power allocation can achieve performance comparable to that of the centralized approach while significantly reducing communication overhead. Our experiments demonstrate the effectiveness of the proposed distributed learning strategies in terms of both convergence rate and spectral efficiency.
更多查看译文
关键词
Federated Learning,Massive MIMO,Training Center,Spectral Efficiency,Communication Overhead,Convolutional Layers,Global Model,Real-world Setting,Local Setting,Selection Mechanism,Training Loss,Heterogeneous Network,Channel Gain,Minimum Mean Square Error,Validation Loss,Local Dataset,Local Training,User Equipment,Central Party,Pilot Signals,Federated Learning Framework,Label Noise,Power Allocation Scheme,Multi-armed Bandit,Minimum Efficiency,Neural Network,Optimal Power Allocation,Party-state,Mean Square Error,Learning Rate
AI 理解论文
溯源树
样例
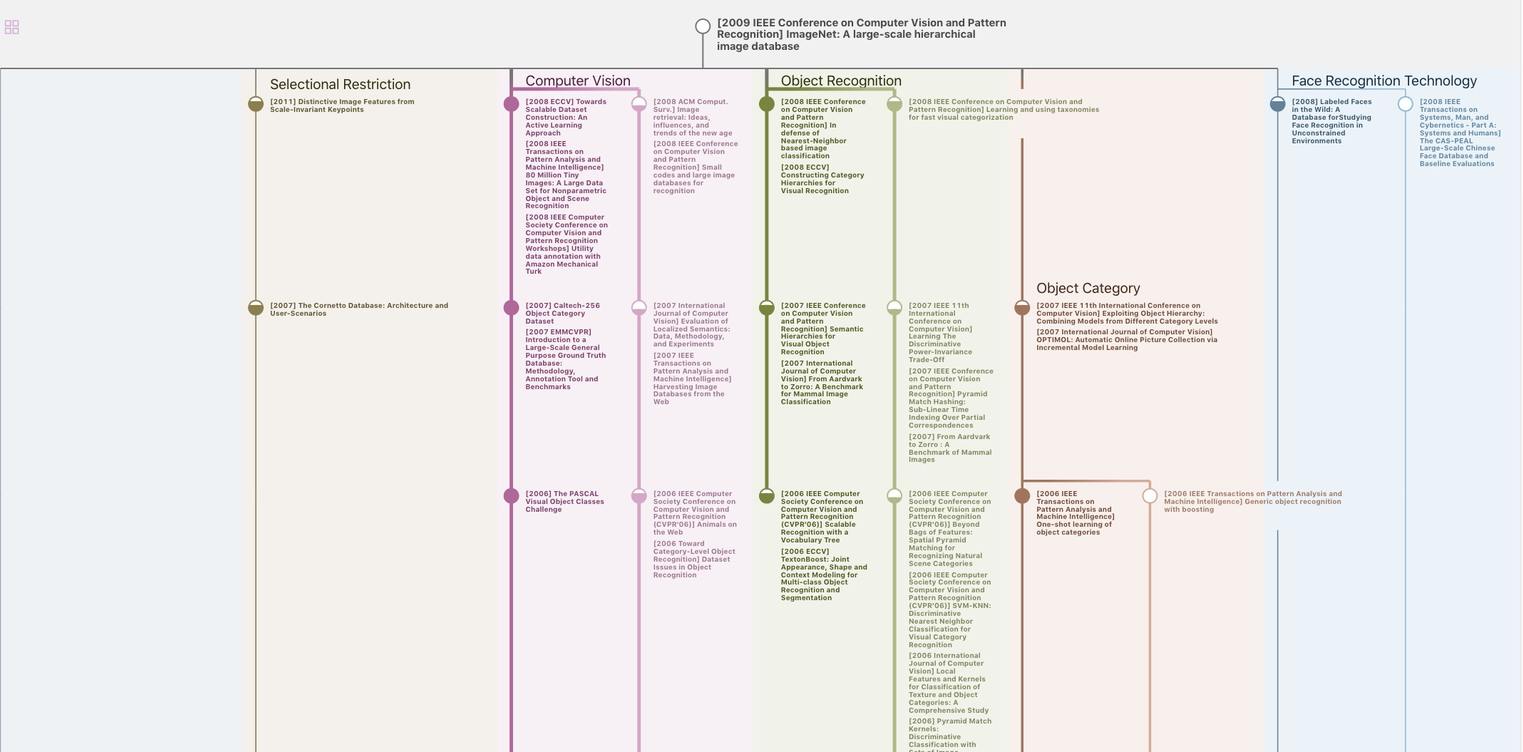
生成溯源树,研究论文发展脉络
Chat Paper
正在生成论文摘要