A deep generative model integrating single-cell time-frequency characteristics transformed from electrophysiological data with transcriptomic features
biorxiv(2024)
摘要
Patch-seq yields multi-modal data (e.g., transcriptomic and electrophysiological data) from single cells. However, currently used analytical methods are based on a few global electrophysiological features predefined from chronological potential changes and overlook the importance of time-frequency domain. In this study, we present LincSpectr, a deep neural network model that integrates transcriptomic and electrophysiological features with the latent variables of various variational autoencoders. This model combines the two modalities according to the connection between the latent variables of different modalities calculated by attention-like mechanisms and achieves cross-modal predictions and an inverse analysis. We discovered that the predicted electrophysiological features changed continuously along with their transcriptional profiles and that the neighborhood relationships between the latent states of the transcriptional profiles were consistent with those of the electrophysiological features. Inverse analysis of our model enabled the extraction of gene sets affecting specific time-frequency domains; some genes were likely to be involved in neural activity. Our approach represents a potential avenue to facilitate the discovery of molecular mechanisms underlying time-frequency activities in various cell types, enhancing our understanding of their roles in neural function.
### Competing Interest Statement
The authors have declared no competing interest.
更多查看译文
AI 理解论文
溯源树
样例
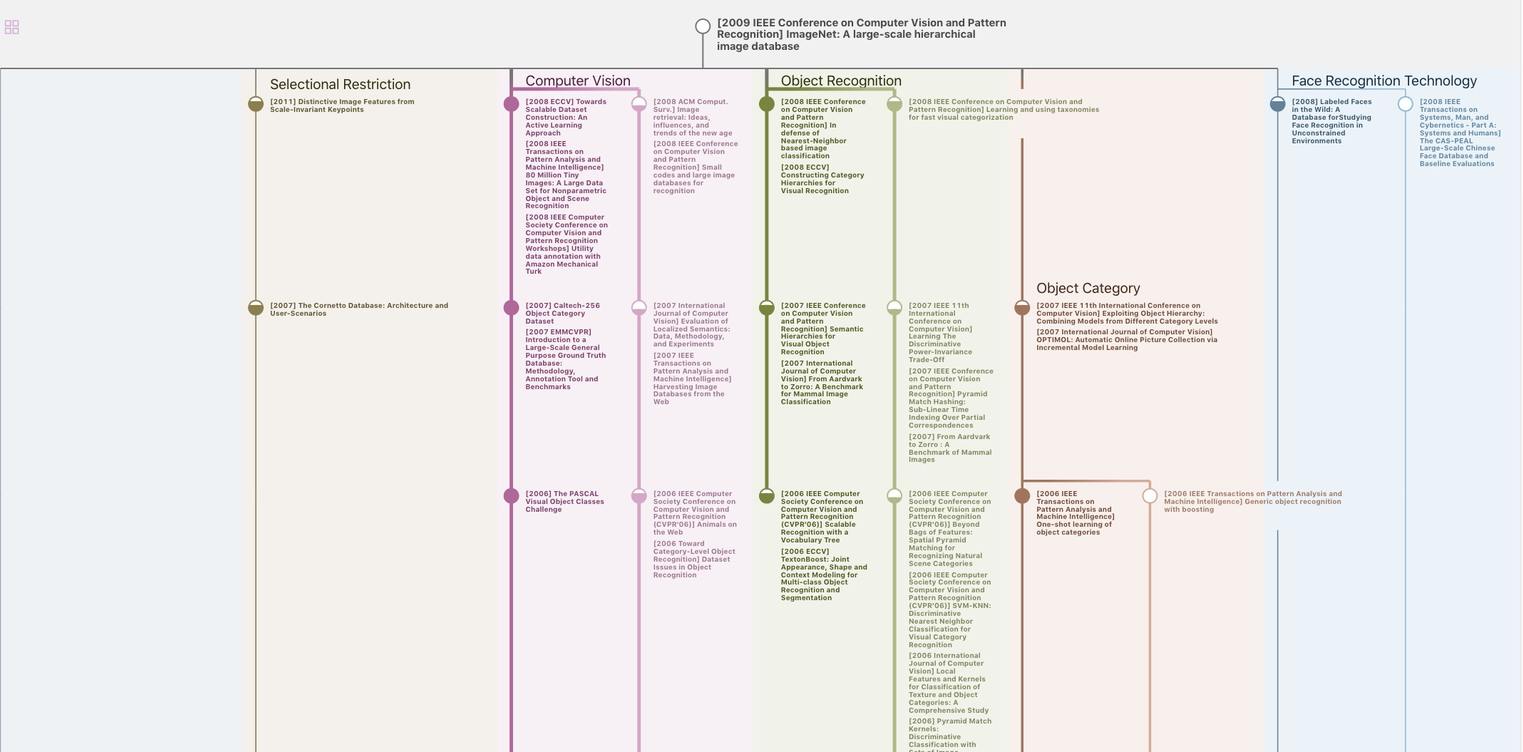
生成溯源树,研究论文发展脉络
Chat Paper
正在生成论文摘要