Assessing Image Filter Effectiveness: A Comparative Study with Noise Injection and Data Augmentation
2024 International Conference on Emerging Systems and Intelligent Computing (ESIC)(2024)
摘要
This research investigates the impact of data augmentation and noise injection on various image filtering techniques employed for static image denoising and image recognition tasks. The study utilizes the MNIST dataset, comprising 60,000 handwritten digit images for testing and 10,000 for training purposes. Several filtering methods, including mean filtering, median filtering, Laplace’s filtering, Gaussian filtering, Canny Edge filtering, spatial filtering, Normalized Box Blur filtering, Quadratic Image filtering, and Bilateral Image filtering, are evaluated. The evaluation involves visually demonstrating the effects of these filters on sample images both before and after training the model. Subsequently, accuracy metrics are computed for each filtering technique. The performance of the filters are measured using Peak Signal to Noise Ration (PSNR) and Normalized Mean Square Error (NMSE).
更多查看译文
关键词
data augmentation,noise injection,image filtering techniques,image denoising,image recognition,MNIST dataset,NMSE,PSNR,static images
AI 理解论文
溯源树
样例
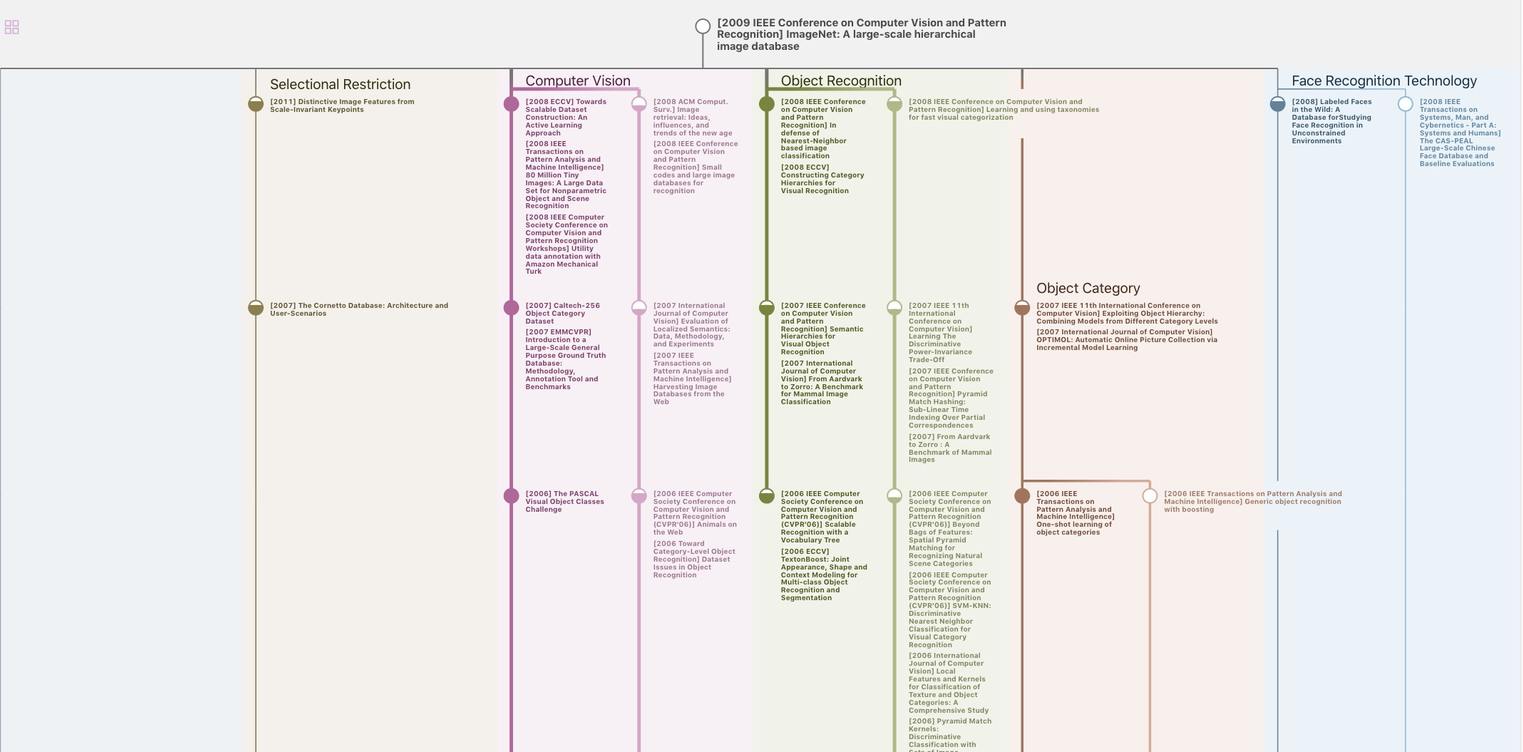
生成溯源树,研究论文发展脉络
Chat Paper
正在生成论文摘要