Self-supervised air quality estimation with graph neural network assistance and attention enhancement
Neural Computing and Applications(2024)
摘要
The rapid progress of industrial development, urbanization, and traffic has caused air quality degradation that negatively affects human health and environmental sustainability, especially in developed countries. However, due to the limited number of sensors available, the air quality index at many locations is not monitored. Therefore, many research, including statistical and machine learning approaches, have been proposed to tackle the problem of estimating air quality value at an arbitrary location. Most of the existing research perform interpolation process based on traditional techniques that leverage distance information. In this work, we propose a novel deep-learning-based model for air quality value estimation. This approach follows the encoder–decoder paradigm, with the encoder and decoder trained separately using different training mechanisms. In the encoder component, we proposed a new self-supervised graph representation learning approach for spatio-temporal data. For the decoder component, we designed a deep interpolation layer that employs two attention mechanisms and a fully connected layer using air quality data at known stations, distance information, and meteorology information at the target point to predict air quality at arbitrary locations. The experimental results demonstrate significant improvements in estimation accuracy achieved by our proposed model compared to state-of-the-art approaches. For the MAE indicator, our model enhances the estimation accuracy from 4.93 R_2 score attained by our proposed model also shows considerable improvement, with increases of 5.39 https://github.com/duclong1009/Unsupervised-Air-Quality-Estimation .
更多查看译文
关键词
Air quality interpolation,Graph neural network,Time-series prediction
AI 理解论文
溯源树
样例
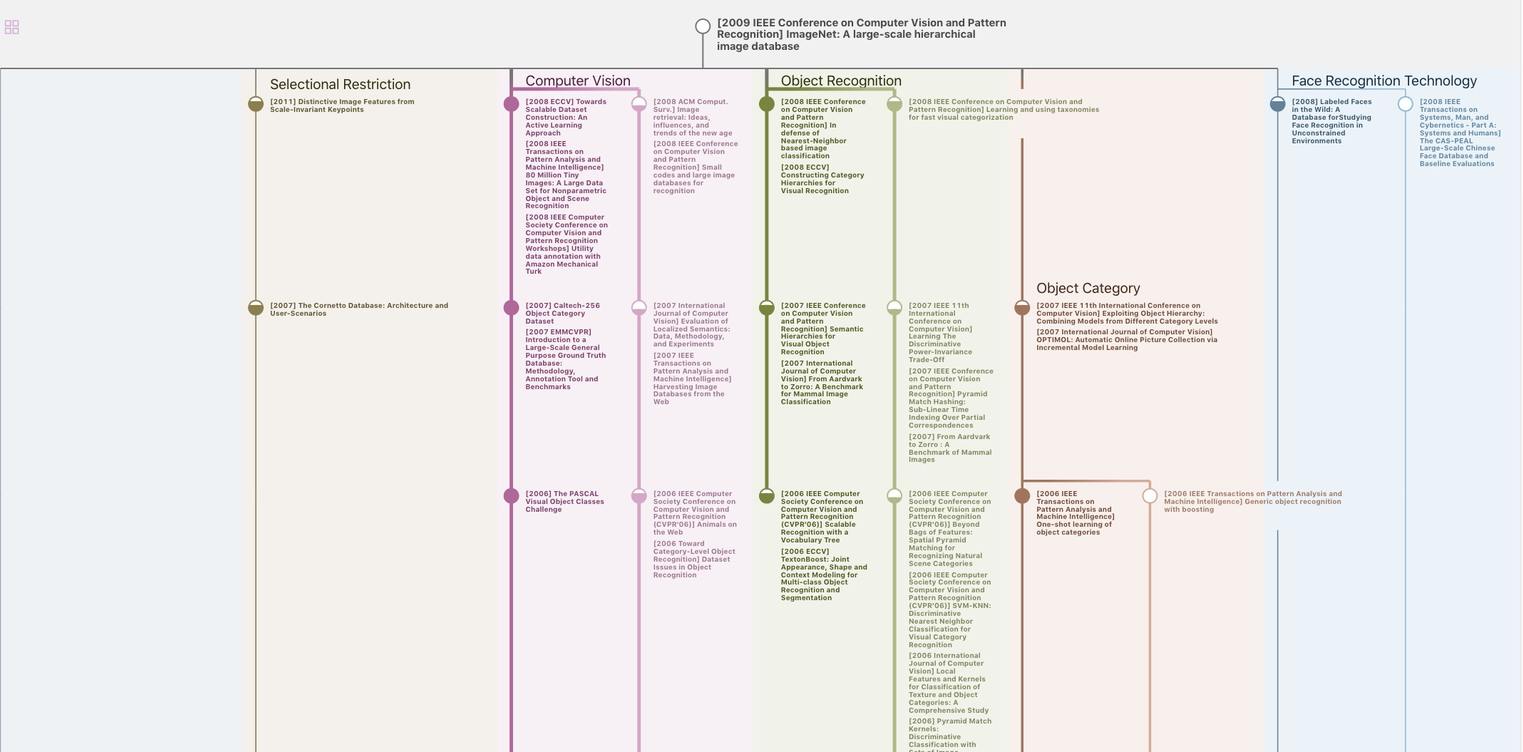
生成溯源树,研究论文发展脉络
Chat Paper
正在生成论文摘要