Leveraging the Strengths of Long Short-Term Memory and Gated Recurrent Unit Architectures for Indian Stock Market Prediction
2024 International Conference on Emerging Systems and Intelligent Computing (ESIC)(2024)
摘要
The study recognizes the multifaceted nature of the stock market and the challenges inherent in predicting market movements accurately. Focused on the Indian stock market, the research harnesses the capabilities of deep learning, specifically the long short-term memory (LSTM) and gated recurrent unit (GRU) architectures, known for their prowess in processing sequential data with predictions over a 10-day horizon. The models are systematically evaluated, considering parameters such as root mean square error (RMSE), mean absolute error (MAE), regression variance, and R2-score, with a comparative analysis highlighting the effectiveness of the proposed hybrid models—LSTM-GRU and GRU-LSTM. The experimental analysis reveals that the GRU-LSTM model demonstrates superior performance on the HDFC bank Ltd. dataset, while the LSTM-GRU model excels on the TATA Motors Ltd. dataset, aligning with the predictive performance and accuracy metrics observed in earlier experiments.
更多查看译文
关键词
Stock market prediction,long short-term memory (LSTM),gated recurrent unit (GRU).
AI 理解论文
溯源树
样例
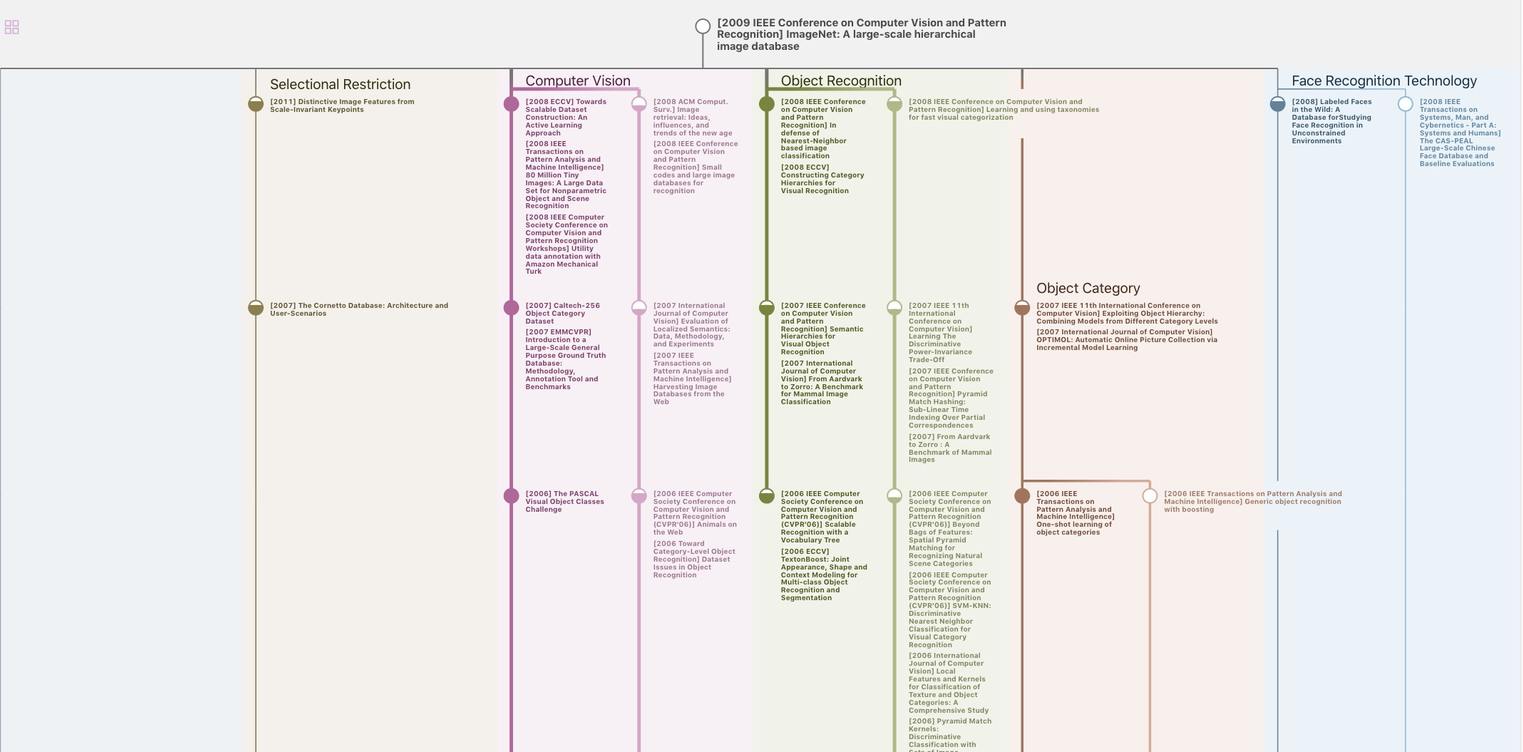
生成溯源树,研究论文发展脉络
Chat Paper
正在生成论文摘要