Statistical analysis, regression, and neural network modeling of the tensile strength of thermoplastic unidirectional carbon fiber-polysulfone composites
Carbon Trends(2024)
摘要
High-strength and high-modulus carbon fibers are the basis of many composite materials used in power and automotive engineering as well as other mechanical engineering fields. Superstructural thermoplastic binders—like PPS, PSU, PES, and PEEK—are emerging quickly as a binder material. The mechanical properties of composite materials, especially tensile strength, are improved when high-strength and high-modulus fibers are combined with superstructural thermoplastic binders. However, the type of carbon fiber used, the concentration of thermoplastic binder, and the specifics of the production process all have a significant impact on the final mechanical properties of the composite material. As such, predicting these properties requires both a thorough analysis and a trustworthy mathematical model that predicts mechanical properties (tensile strength).The study that is being presented takes a thorough approach to statistical analysis and model building that anticipates the tensile strength of composite material samples made of carbon filaments that have been impregnated with polysulfone (PSU), a thermoplastic polymer.PSU thermoplastic polymer was used as a binder, and 817 samples of composite material with high-strength and high-modulus carbon fibers of four different grades were subjected to a thorough statistical analysis of the tensile test findings.Nine distinct regression models and four CNN-based models with three distinct neuron activation functions were constructed based on the statistical analysis. The built-in models forecast the composite material's ultimate strength based on the specimen loading circumstances, filler qualities, and composition.Significant differences were found in the mechanical properties of carbon fibers of different grades and types (high-strength and high-modulus) based on statistical analysis of the results of tensile tests. The results of Spearman's correlation study indicated a medium positive correlation between ultimate strength and polymer concentration and a weak negative association between ultimate strength and the density of the carbon fiber contained in the composite material. The strain corresponding to the ultimate strength and fiber density were found to have a medium negative correlation, whereas the polymer concentration showed a medium positive correlation. In the composite material, a very slight negative association was discovered between the concentration of polymers and the density of carbon fibers.Test results were split into two categories while creating CNN and regression models: 75% were used for model testing and 25% were used for training. The CNN model with three layers of hidden parameters produced the best prediction results; the RMSE was 142.948 MPa and the Spearman correlation coefficient between the test strength and the anticipated values was 0.988.Regression models' sensitivity analysis revealed that, up to a response variable (tensile strength) value of 0.75, fiber density has a greater influence than polymer density, with sample loading rate having the least impact. Beyond this point, fiber density essentially has no effect on tensile strength; polymer concentration and sample loading rate have the greatest effects. A minimal tensile strength at fiber density in the region of 0.1 of the maximum density and an abnormal decrease in tensile strength with increasing polymer concentration at the response variable value of 0.75 were discovered during the CNN model's sensitivity analysis. Additionally, it was discovered that, in contrast to other regression models, such as neural networks, the partial least squares model and LASSO are sensitive to the existence of groups in the data.To accomplish the specified structural features of tensile strength, the carbon fiber-PSU composite material production process' technological parameters can be optimized using the modeling and statistical analysis results that were acquired.
更多查看译文
关键词
Composite material,thermoplastic polymer,polysulfone,carbon fiber,polymer-matrix composites,Carbon fiber composites,fiber reinforced thermoplastics,mechanical properties,neural network,regression model,statistical analysis,robust regression
AI 理解论文
溯源树
样例
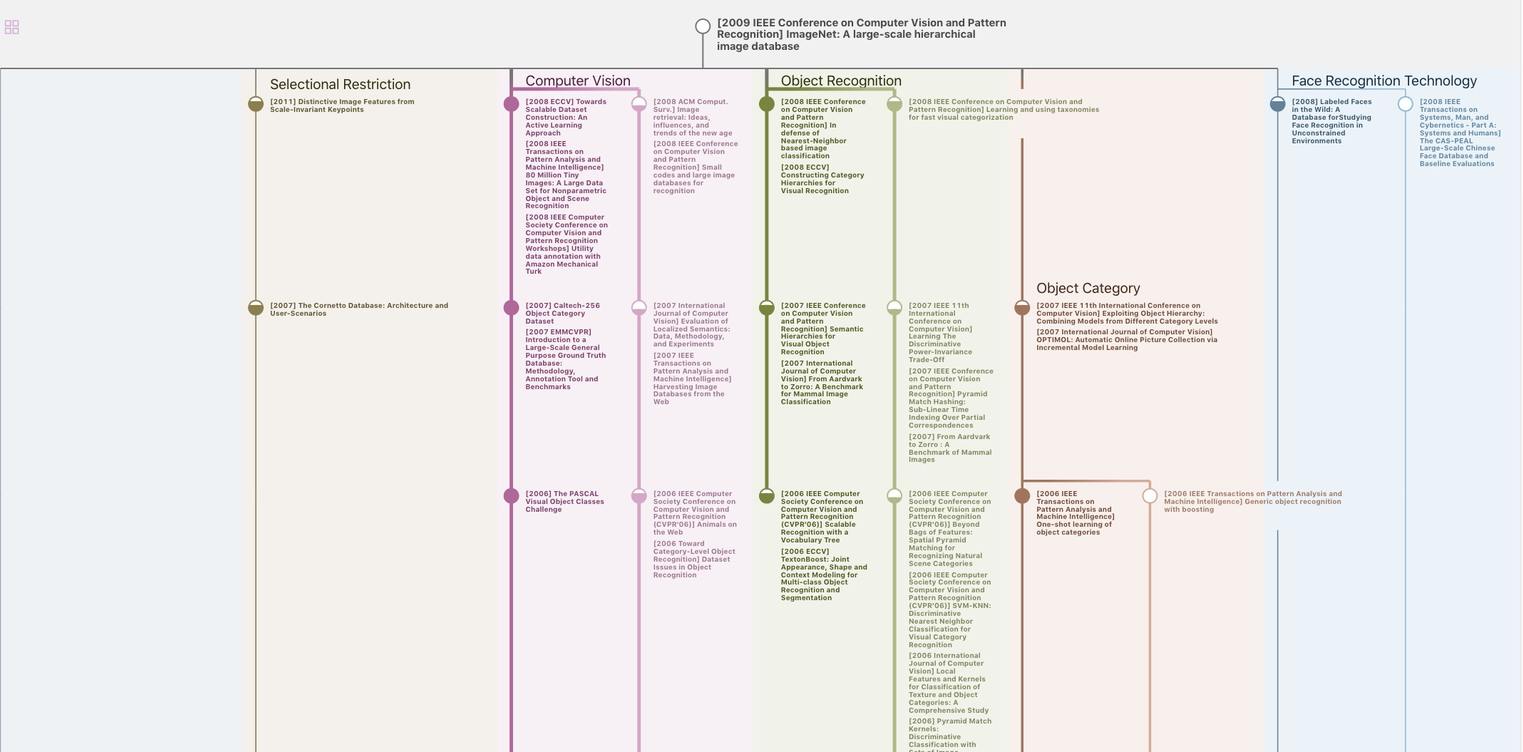
生成溯源树,研究论文发展脉络
Chat Paper
正在生成论文摘要