DeepStock Forecast: Unveiling Market Movements through Advanced Deep Learning Models
2024 International Conference on Emerging Systems and Intelligent Computing (ESIC)(2024)
Abstract
This study uses deep neural network (DNN) methodologies, including auto encoder, deep belief network (DBN), and backpropagation neural network (BPNN), to predict stock prices over 15 and 30 days. Utilizing BSE Sensex and NSE Sensex datasets with diverse technical indicators, the research highlights DNN’s superior training accuracy, measured by minimal mean squared error (MSE). The study’s key contribution is applying DNN to predict stock market volatility. While acknowledging the limitation of relying solely on training error, the study incorporates additional measures like RMSE, MAPE, MAE, and ARV during testing. Notably, DNN’s MAPE results fall within a narrow range (0.0221 to 0.0255), indicating minimal error deviation. In a comprehensive evaluation across all datasets, DNN consistently outperforms DBN and BPNN in both training and testing performance.
MoreTranslated text
Key words
Stock market prediction,Deep Neural Network (DNN),Deep Belief Network (DBN),Back Propagation Neural Network (BPNN)
AI Read Science
Must-Reading Tree
Example
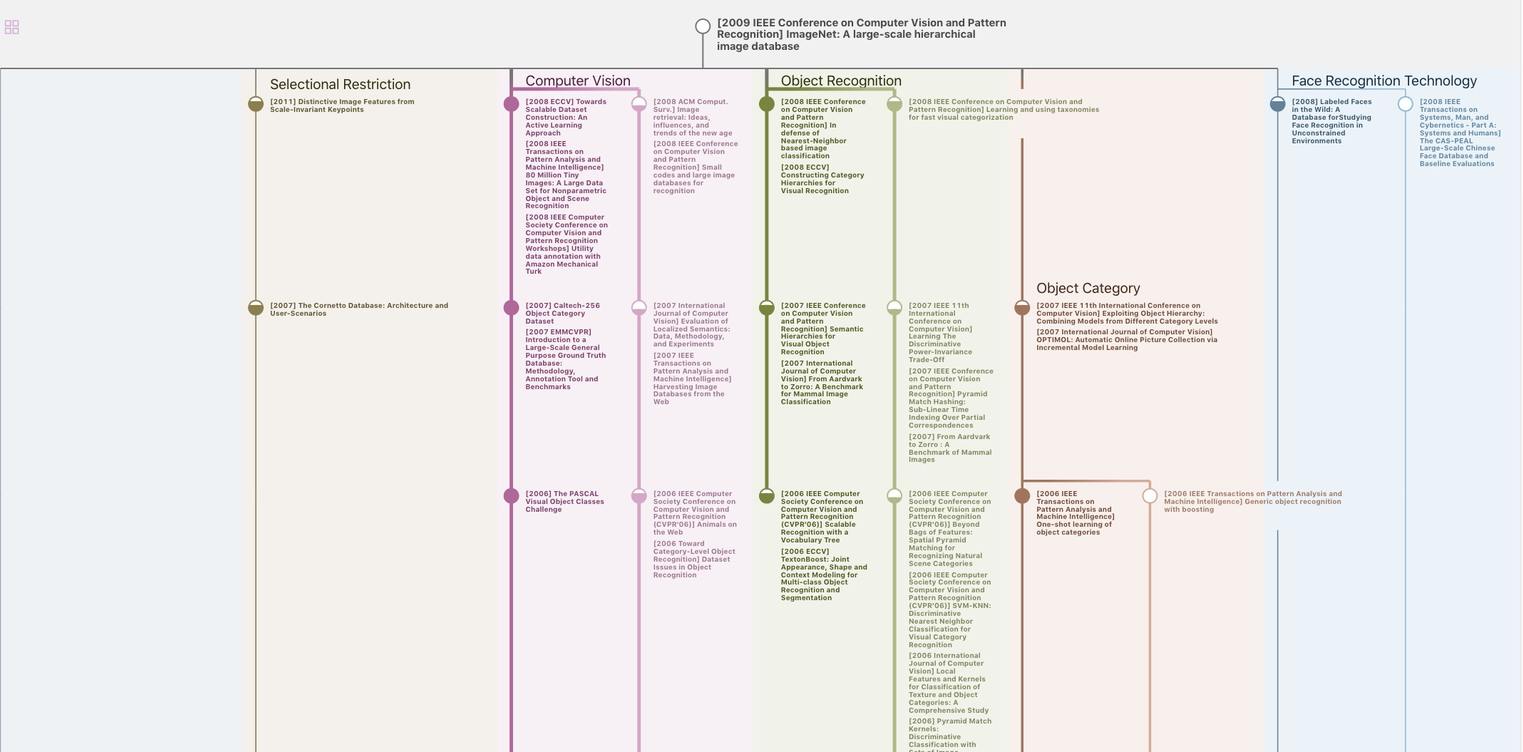
Generate MRT to find the research sequence of this paper
Chat Paper
Summary is being generated by the instructions you defined