Least Squares Inference for Data with Network Dependency
arxiv(2024)
摘要
We address the inference problem concerning regression coefficients in a
classical linear regression model using least squares estimates. The analysis
is conducted under circumstances where network dependency exists across units
in the sample. Neglecting the dependency among observations may lead to biased
estimation of the asymptotic variance and often inflates the Type I error in
coefficient inference. In this paper, we first establish a central limit
theorem for the ordinary least squares estimate, with a verifiable dependence
condition alongside corresponding neighborhood growth conditions. Subsequently,
we propose a consistent estimator for the asymptotic variance of the estimated
coefficients, which employs a data-driven method to balance the bias-variance
trade-off. We find that the optimal tuning depends on the linear hypothesis
under consideration and must be chosen adaptively. The presented theory and
methods are illustrated and supported by numerical experiments and a data
example.
更多查看译文
AI 理解论文
溯源树
样例
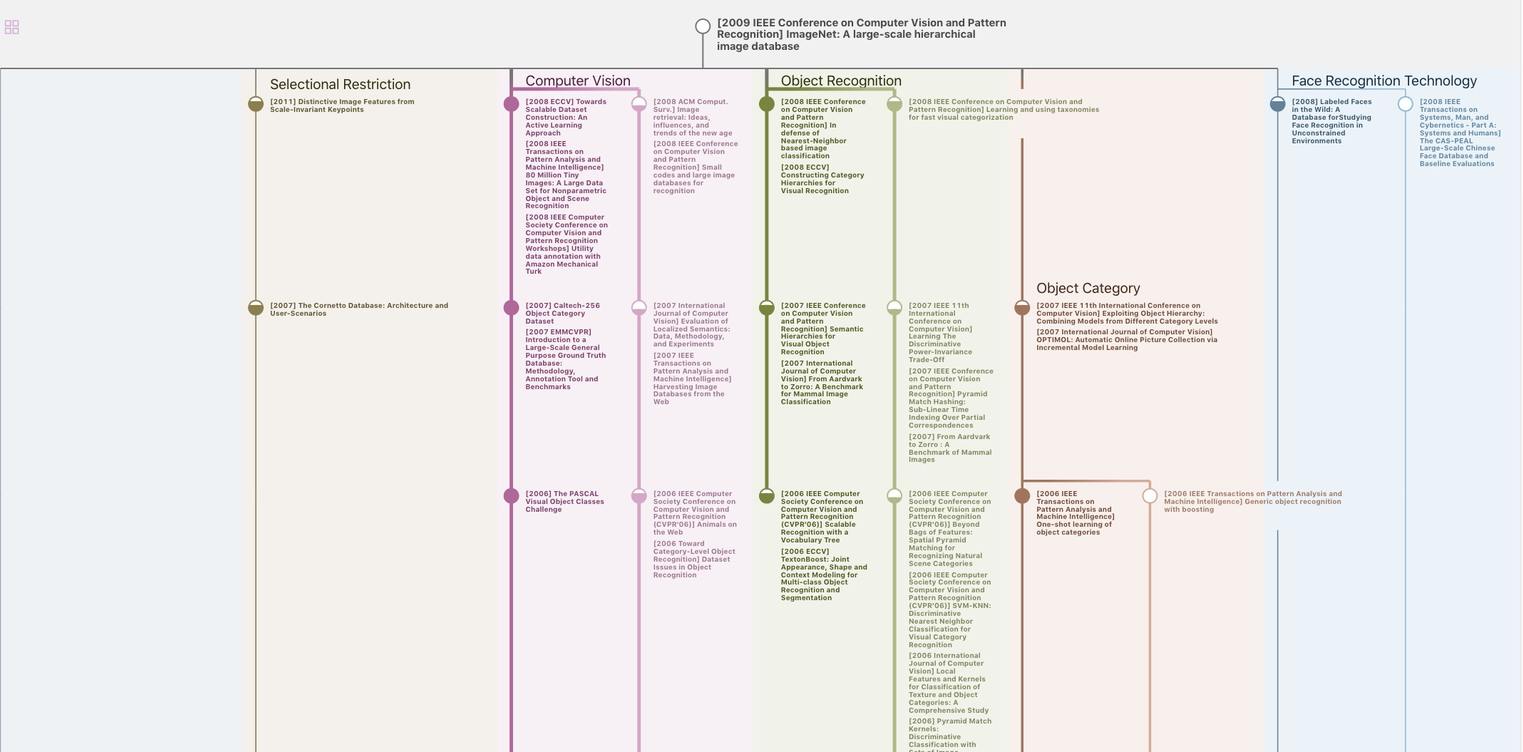
生成溯源树,研究论文发展脉络
Chat Paper
正在生成论文摘要