QUSL: Quantum Unsupervised Image Similarity Learning with Enhanced Performance
arxiv(2024)
摘要
Leveraging quantum advantages to enhance machine learning capabilities has
become a primary focus of research, particularly for complex tasks such as
image similarity detection. To fully exploit the potential of quantum
computing, it is essential to design quantum circuits tailored to the specific
characteristics of the task at hand. In response to this challenge, we propose
a novel quantum unsupervised similarity learning method, QUSL. Building upon
the foundation of similarity detection triplets and generating positive samples
through perturbations of anchor images, QUSL operates independently of
classical oracles. By leveraging the performance of triplets and the
characteristics of quantum circuits, QUSL systematically explores
high-performance quantum circuit architectures customized for dataset features
using metaheuristic algorithms, thereby achieving efficient quantum feature
extraction with reduced circuit costs. Comprehensive experiments demonstrate
QUSL's remarkable performance compared to state-of-the-art quantum methods.
QUSL achieves reductions exceeding 50
while also realizing an enhancement of approximately 20
detection correlation across the DISC21, COCO, and landscape datasets. This
enables efficient quantum similarity modeling for large-scale unlabeled image
data with reduced quantum resource utilization.
更多查看译文
AI 理解论文
溯源树
样例
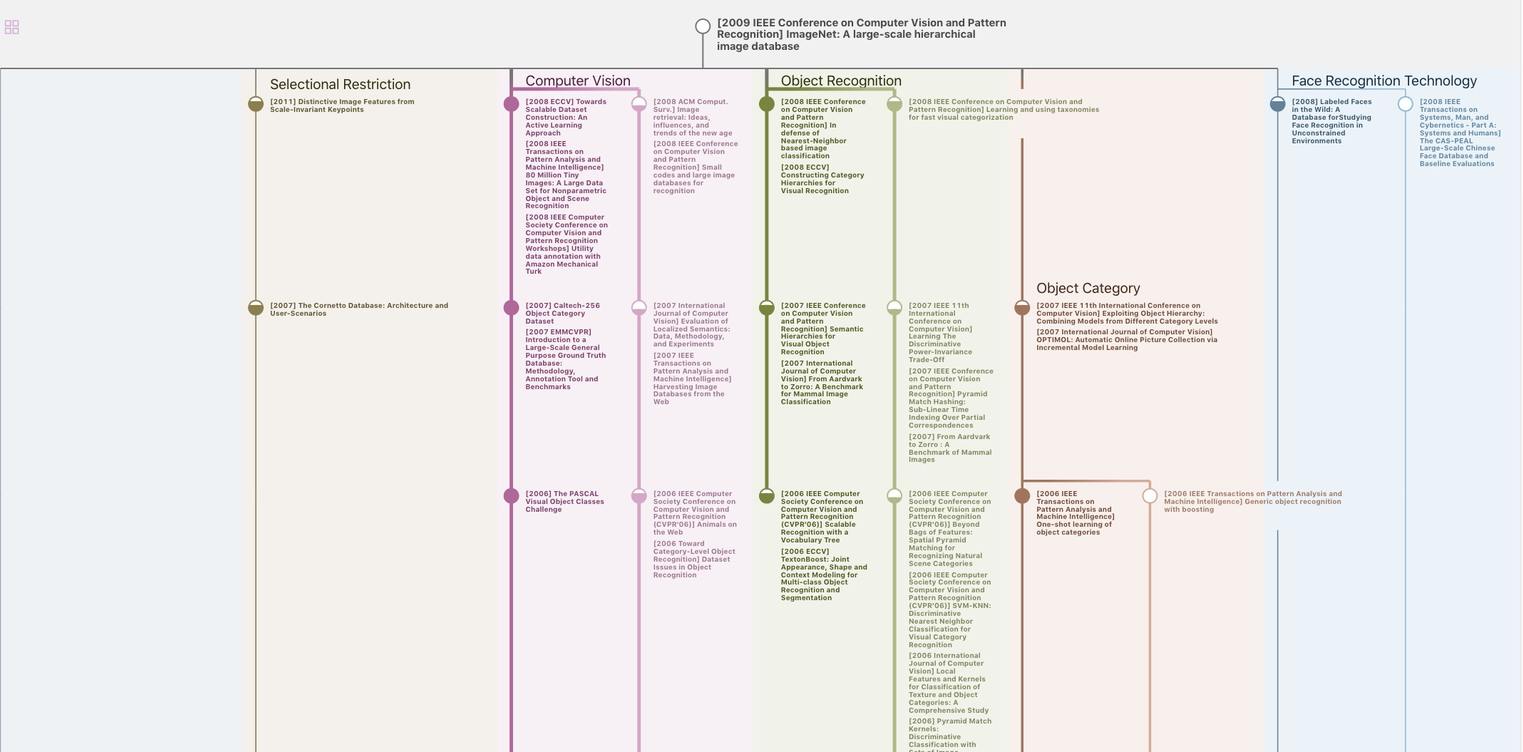
生成溯源树,研究论文发展脉络
Chat Paper
正在生成论文摘要